Optimising wet granulation manufacturing with advanced technologies
Posted: 1 October 2024 | Catherine Eckford (European Pharmaceutical Review) | No comments yet
The approach proposed in the paper suggests how artificial intelligence (AI) and machine learning (ML) could enhance process efficiency and product quality during complex manufacturing.
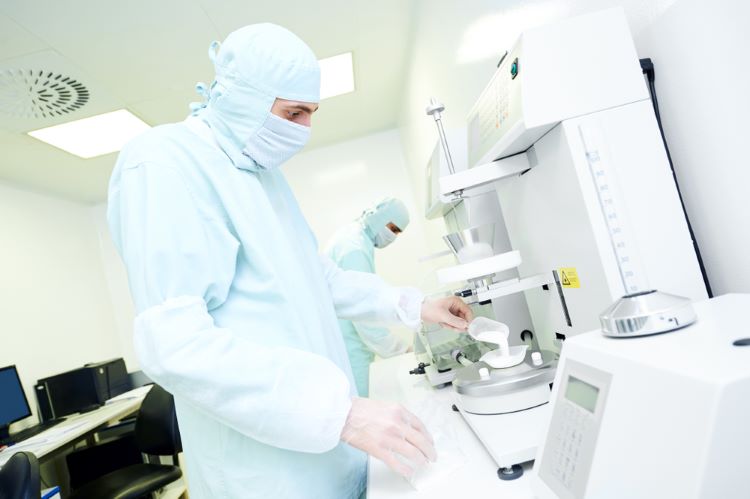
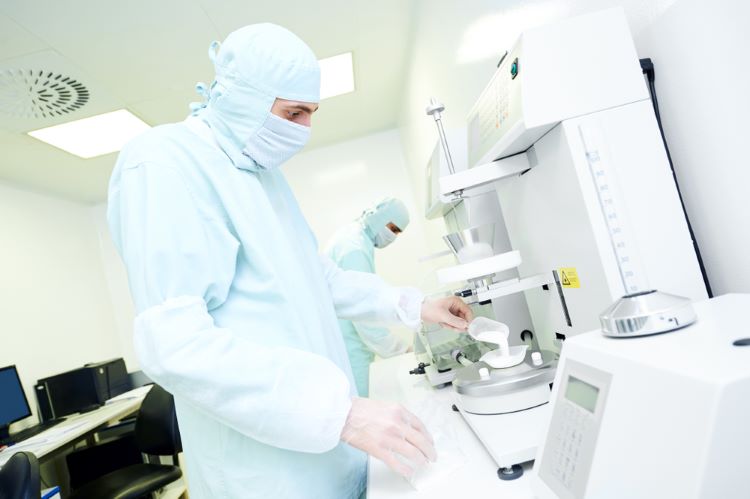
A paper has demonstrated a novel application of optimising processes to implement inverse design in pharmaceutical manufacturing. Machine learning techniques were assessed for use as a surrogate model to optimise a three-unit wet-granulation based flowsheet model for the manufacture of solid dosage forms.
Dan et al. highlighted that recent research has begun to investigate the potential applications of deep learning in process development, however currently, use of this technique in this area “is not yet widespread”.
However, “in recent years, there has been a paradigm shift to the utilisation of model-based strategies, such as process models to simulate several unit operations in powder processing, including mixing”.
Aa novel dissolution model was developed that accounted for how particle size, porosity, and microstructure impacted the dissolution rate.
Two optimisation approaches were compared: an autoencoder-based inverse design and a surrogate-based forward optimisation. In the study, the autoencoder-based inverse design used artificial intelligence (AI) and ML in pharmaceutical process development.
[the approach] holds potential to enhance efficiency and product quality in complex manufacturing”
Dan et al. wrote that these methods “effectively addressed the multi-objective problem of maximising dissolution time while maximising yield to achieve target product quality”.
The surrogate-based optimisation “iterates over the surrogate model to find the optimal solution” and was reported to be “computationally efficient as it completes in under a minute”.
Inverse design optimisation begins by establishing a “pre-defined target product quality and uses computational algorithms to generate the possible combinations of parameters to produce the final product with the specified output”, the authors explained.
Future applications in pharmaceutical process development for manufacturing
Overall, the study showed that the approach proposed holds potential to enhance efficiency and product quality in complex manufacturing. Therefore, it could be “a great alternative option” to the more common surrogate-based optimisation, especially when targeting specific product qualities in these operations, the authors surmised.
The paper is being published in the International Journal of Pharmaceutics: X.