Using mass spectrometry data to expedite drug discovery
Posted: 15 October 2021 | Hannah Balfour (European Pharmaceutical Review) | No comments yet
A new algorithm uses mass spectrometry data and machine learning to predict whether a molecule has been discovered before, saving drug discovery time and cost.
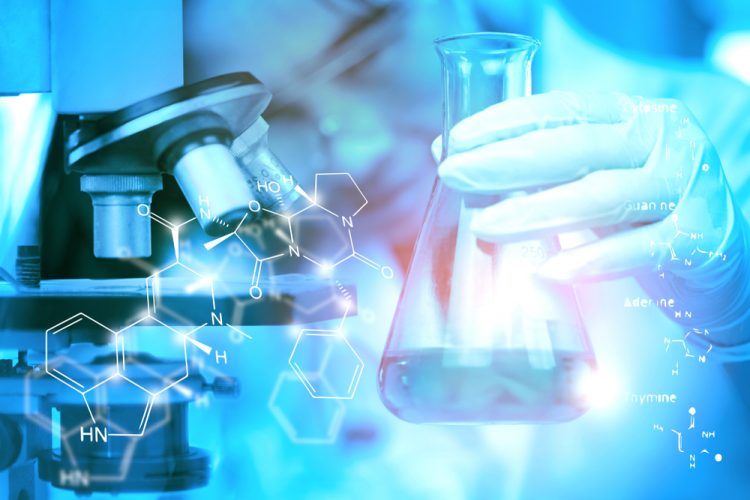
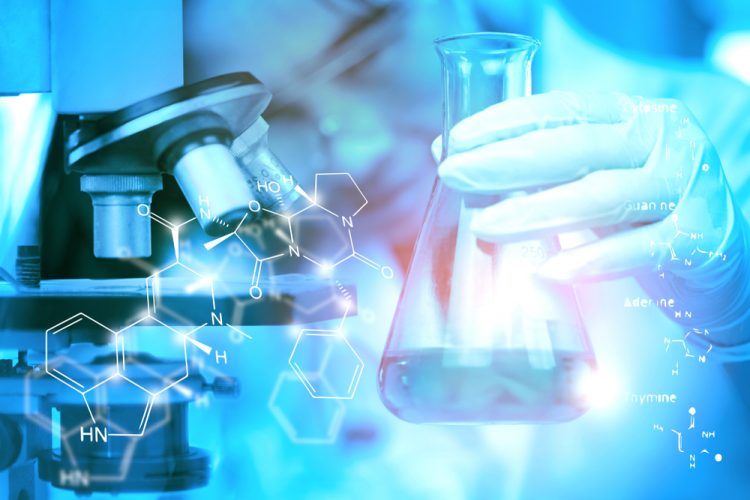
Researchers have developed an algorithm, called MolDiscovery, which uses mass spectrometry data from molecules to predict the identity of unknown substances. According to the developers, the algorithm can tell scientists early in their research whether they have discovered a new molecule, or one identified before, saving time and money in the drug discovery and development process.
MolDiscovery was created by researchers at Carnegie Mellon University’s Computational Biology Department, US, and St Petersburg State University, Russia, and research related to it was published in Nature Communications.
“Scientists waste a lot of time isolating molecules that are already known, essentially rediscovering penicillin,” explained Hosein Mohimani, an assistant professor in Carnegie Mellon University’s Computational Biology Department and part of the research team. “Detecting whether a molecule is known or not early on can save time and millions of dollars and will hopefully enable pharmaceutical companies and researchers to better search for novel natural products that could result in the development of new drugs.”
According to Mohimani, whose research in the Metabolomics and Metagenomics Lab focuses on the search for new, naturally occurring drugs, after a scientist detects a molecule that could be a potential drug in a marine or soil sample, it can take more than a year to identify the molecule with no guarantee that the substance is new. MolDiscovery uses mass spectrometry measurements and a predictive machine learning model to identify molecules quickly and accurately.
MolDiscovery predicts the identity of a molecule from the mass spectrometry data without relying on a mass spectra database to match it against, since there is no repository of mass spectrometry data for all previously discovered molecules.
The team hopes MolDiscovery will be a useful tool for labs in the discovery of novel natural products. They explained that MolDiscovery could work in tandem with NRPminer, a machine learning platform developed by Mohimani’s lab, that helps scientists isolate natural products. Research related to NRPminer was also recently published in Nature Communications.
Related topics
Analytical techniques, Artificial Intelligence, Drug Development, Drug Discovery, Mass Spectrometry, Research & Development (R&D)