Creating a modern business landscape in biopharma
Posted: 13 May 2019 | Unjulie Bhanot | No comments yet
There are many business challenges to developing a biologics drug, one of which is effective data management. In this article, Unjulie Bhanot focuses on efficient data management policies and systems, and how they could improve biologics product development processes.
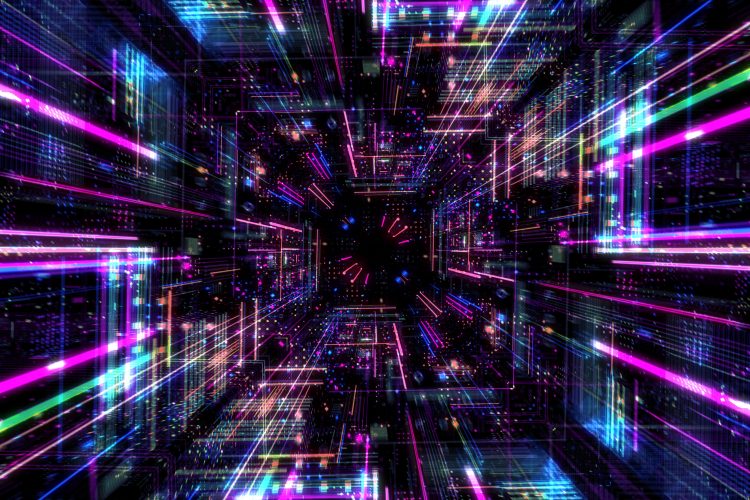
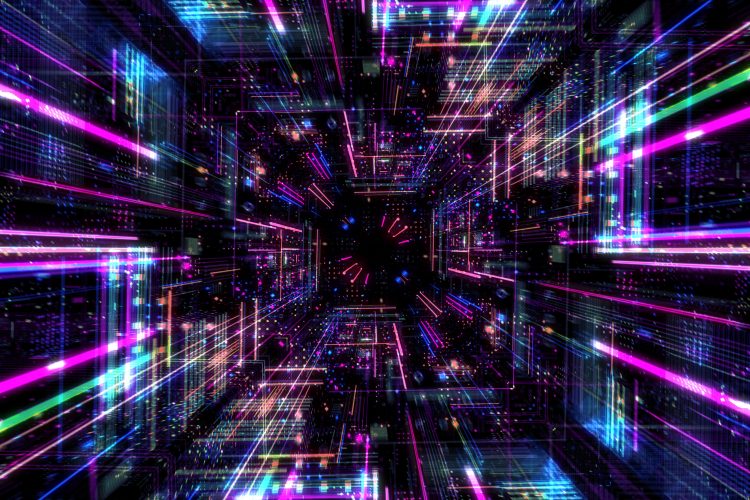
Biologics therapies, in the form of monoclonal antibodies and recombinant proteins, have proven success in the medical market with six out of the global top 10 treatments taking this form in 2017.1 However, the development journey of a biologics drug to its final destination in the market can take up to 12 years;2 a journey that always comes with its own scientific, operational and technological challenges. Biopharma organisations are also under pressure to reassess their development strategies; factors such as the rise in scientific advances and changing therapeutic requirements are amplifying this pressure. Such organisations must ensure they benefit from the latest scientific and process innovations to shorten their time to market and release relevant and targeted molecules – all while adhering to the growing list of regulatory and compliance requirements and government policy reforms.
Players in the space must also compete with the shift of drug development to growing markets, such as India and China, and their ability to support lower value markets. Consequently, these large biopharma organisations are looking to partner with technology firms to leverage their talent to support the digital therapeutic market. Examples include the partnering of Pfizer and IBM to deliver technology that performs real-time monitoring of the symptoms of Parkinson’s,3 UCB and MC10 creating sensors that monitor key parameters to develop therapies for neurological disorders,4 as well as Biogen and Verily (Google’s life sciences arm) using sensors and software to study the biological and environmental factors contributing to multiple sclerosis.3 In-vitro companion devices are also being implemented in combination with drug development, to gauge which patient cohort will most likely benefit from the therapeutic biological product in development and identify those patients most likely to be at increased risk of non-beneficial side effects.5 By using these technologies in tandem, organisations can target the right therapeutic areas and make decisions early on about whether to develop a molecule for a specific disease.
Tackling the business challenges
Owing to this combination of factors, organisations rely on four key areas to help support the development of a drug and overcome the business’s challenges:
- Science and technology
- Resources and project planning
- Outsourcing and collaboration
- Business-driven compliance policies and procedures.
In the lab, these tools are tied together by the thread of data and information – such as performing scientific data analysis to understand the impact of a given instrument; using information from personnel training to plan experiments; or managing sample transfers based on release data.
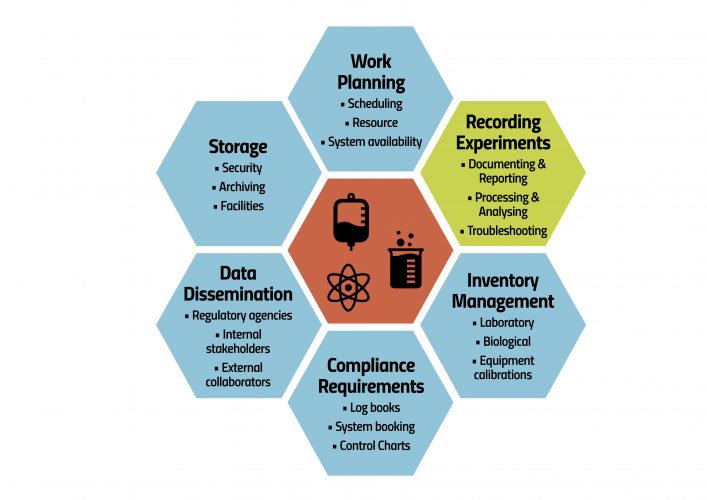
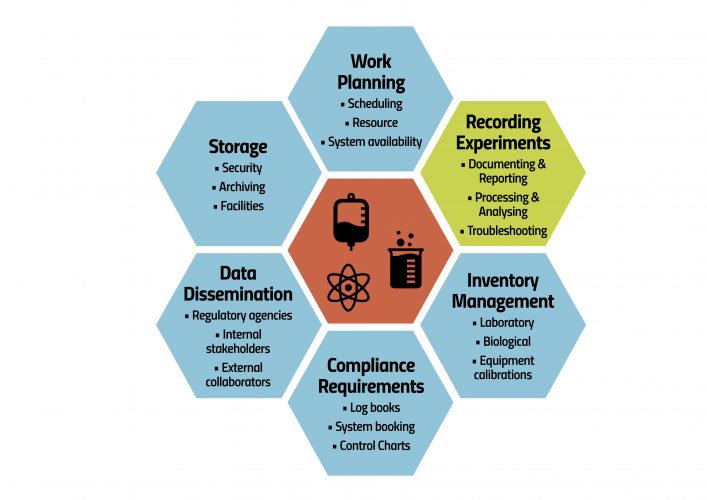
Figure 1: Activities a scientist may encounter in which data is either created, utilised, manipulated or managed
Furthermore, organisations require both top-level and detailed views of their data to make informed decisions about the correct biological formation and purity of the drug, its efficacy and potency, and the impact of supplementary conduits and processes. Organisations also need an overall view of the development strategy and its success. For data to become consumable information, context is critical. The ability to piece together that context, determine what data should be used before extracting relevant data, and compile/aggregate the pertinent data is critical. This requires efficient data management policies and systems. Figure 1 depicts daily activities that scientists may encounter, in which data is either created, utilised, manipulated or managed, with most emphasis given to the scientist’s key role of executing the science.
Current data management in labs
Inept management of any of these tasks has consequences for the business – be they only small oversights that are immediately remediable. Whether the gap takes five minutes to close or a few hours, it’s important to acknowledge that the impact extends to the overall business and could cause incremental damage. Imagine a scenario where an instrument fails its calibration, but this data point is not recorded. Many people then use the instrument and their experiments fail; the mistake only being caught several experiments down the line and perhaps only linked to the instrument after much work has been reviewed. In this scenario, time has been lost, rework has been triggered and may also have exhausted reagent stock, project timelines are delayed and it could cause a compliance failure where experimental results have been used in GMP. In an everyday scenario, the combination of these activities generates lots of data that is acquired or recorded, processed and analysed, stored and then disseminated. To follow the path of this data, and understand the relationship between the data points, scientists must assimilate process data with parameter data, together with experimental results. While evidencing the science performed, scientists may also be expected to duplicate the same metadata across different systems.
Additionally, with the deployment of instrumentation of the modern-day lab, such as high-throughput systems (HTS) or process analytical technology (PAT) tools, scientists are required to be proficient in drawing information from the reams of data these systems can yield.6 To achieve this, they must master the skills needed to decide which sets of data are most relevant, provide the most insight, and should be moved forward for analysis and report compilation. Many R&D organisations are making the investment to ensure they either hire or train personnel to be confident analysing large volumes of data.7
Take a reasonably common example in screening analysis: each well in a 96-well plate may generate an image of 2MB (~200MB per plate), extend this across five time points and suddenly the volume of data jumps to 1GB. Now extrapolate this across 100 plates, across five time points, and 100GB of data from a single experiment can become difficult to manage. The scientist may also need to determine whether to analyse all wells across all timepoints across all plates. There may also be some numerical calculations associated with these wells (absorbance values, concentrations, etc), what’s their format? Can this information be extracted and consumed? Most importantly, are we able to reconcile the image with its corresponding numerical data, with the context of knowing what was in the given well on the defined plate? We can start to see how unmanageable the volume of data can be; and this does not even address the storage challenge.
Designing an effective data management strategy
An ideal solution need not be a complicated one; however, it should be purposeful, and its role and position should be fully defined. The strategy designed should serve both the scientists and the overall organisation.
It is here where seamlessly integrated systems hold most value – by creating and understanding the full laboratory and organisation landscape of all the moving parts, dependencies, human interventions and, most importantly, data collection and handoff points. Software can be strategically utilised to facilitate smoother data transaction, thus reducing the burden on the scientist while maintaining data integrity. Systems that permit data to be recorded vicariously as part of performing a procedure and do not need manual duplication, will be the least burdensome on scientists. This will allow them to focus on their core work and for organisations to make an impact on both their returns and main business goals.
Equally, systems that can communicate with one another without requiring human mediation and can streamline data transfers, will enhance reliability of the data in question. When creating the ‘landscape of the lab’, organisations should map out the journey of the data. What purpose does it serve, what question is it trying to answer, who needs to consume the data, and, inevitably, is the quality of the data sufficient to validate the journey of the biologic through its development? Businesses may wish to consolidate business metrics with the reporting of scientific outcomes; for example, what was the duration of a particular stage of work, and was there an impact on its success or failure corresponding to the resources available? Ultimately, organisations aim to deliver novel, high-quality therapeutics to patients faster and more cost effectively. Therefore, it is critical to understand the collective workforce (personnel and instrumentation) that contributes to this pursuit.
Considering our assessment of a day in the life of a scientist (Figure 1), it is clear to see the impact an integrated platform that allows data to be recorded in a consistent, structured manner and connects different factions of the workflow could have. Put simply, organisations would be better supported in the current competitive R&D landscape with their endeavour to bring their biologic to patients faster, with a system that could accurately calculate the length of time an experiment will take when scheduling work, or could track and store sample metadata in tasks managing sample testing.
What about a system that could manage the compliant use of instruments as well as their output? Or one that could ensure that the activities performed to support compliance could be an automatic outcome of users entering data in their experiments? Even with these few examples, it is easy to see how the deployment of an enterprise-ready platform, specifically designed to support the biologics data workflow, could form the core of an effective data management strategy, empower businesses to make better decisions with improved product and process insight, shrink reporting timelines and expedite seamless data dissemination.
Biography
Unjulie Bhanot is a UK-based Solutions Consultant at IDBS and has worked in the biologics R&D informatics space for over five years. Unjulie holds a BSc in Biochemistry and an MSc in Immunology, both from Imperial College London. Since joining IDBS in 2016, Unjulie has been responsible for designing and deploying informatics solutions for biologics-based organisations within Europe. In 2017, she took on a leading role in the development of the IDBS bioprocess solution. Prior to joining IDBS, Unjulie worked as an R&D scientist at both Lonza Biologics and UCB, and later went on to manage the deployment of the IDBS E-WorkBook Platform within the analytical services department at Lonza Biologics in the UK.
References
1. industry statistics, Hardmann & Co, 11 April 2018 https://www.hardmanandco.com/wp-content/uploads/2018/09/global-pharmaceuticals-2017-industry-stats-april-2018-1.pdf
2. Drug development: the journey of a medicine from lab to shelf – The Pharmaceutical Journal, 12 May 2015, Ingrid Torejesen https://www.pharmaceutical-journal.com/publications/tomorrows-pharmacist/drug-development-the-journey-of-a-medicine-from-lab-to-shelf/20068196.article?firstPass=false
3. Wearables: A World of Pharma Partnership And Potential – Pharma Intelligence, 07 June 2017, Melanie Senior https://pharmaintelligence.informa.com/resources/product-content/a-world-of-pharma-partnership-and-potential
4. Press Release from MC10: MC10 and UCB Complete Landmark Collaboration Involving Parkinson’s, January 05, 2017 https://www.mc10inc.com/press-media/mc10-ucb-landmark-home-monitoring-collaboration
5. In Vitro Diagnostics – Companion Diagnostics, U.S. Food and Drug Administration, July 2018 https://www.fda.gov/medicaldevices/productsandmedicalprocedures/invitrodiagnostics/ucm407297.htm
6. Advanced Biopharmaceutical Manufacturing: An Evolution Underway – Deloitte, May 2015 https://www2.deloitte.com/us/en/pages/life-sciences-and-health-care/articles/advanced-biopharmaceutical-manufacturing-paper.html
7. 7 Data Challenges in the Life Sciences – Technology Networks, 02 May 2017, Jack Rudd https://www.technologynetworks.com/informatics/lists/7-data-challenges-in-the-life-sciences-288265
Issue
Related topics
Data Analysis, Pipelines, Regulation & Legislation, Research & Development (R&D), Technology