Open data policies in proteomics are starting to revolutionise the field
Posted: 28 February 2019 | Juan Antonio Vizcaíno - European Bioinformatics Institute and Wellcome Trust Genome Campus | No comments yet
High-throughput mass spectrometry (MS)-based proteomics approaches have developed enormously in recent years and are continuing to do so. The current trend sees proteomics being used by academia and industry to tackle intricate biological questions, often in conjunction with other high-throughput “omics” disciplines such as genomics, transcriptomics and metabolomics. Within the pharmaceutical industry, proteomics has proved to be a valuable platform for protein-based biomarker discovery as well as for target identification and validation.
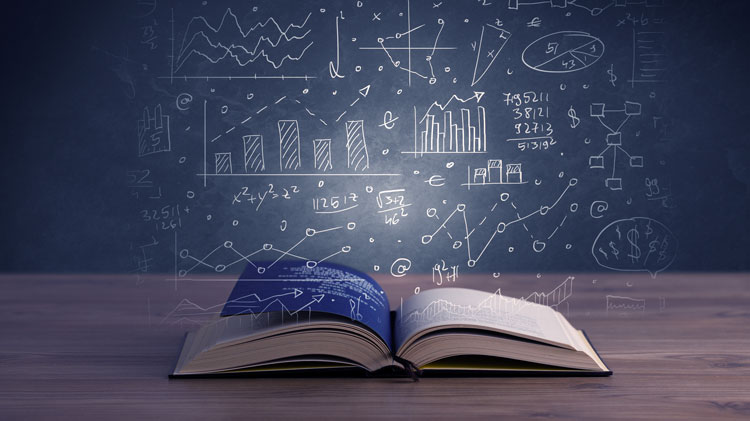
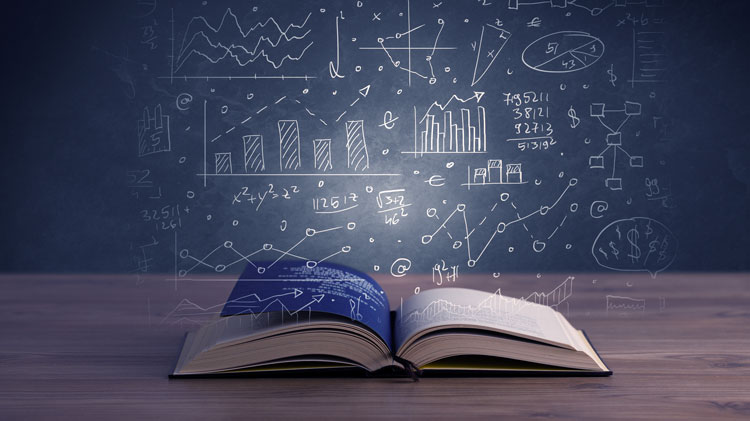
Data growth
The rapid development of the field has primarily been driven by technological progress in areas such as MS instrumentation, chromatographic separation, genomics, and computational proteomics and software. However, open data policies have only recently become more widely accepted in the field and, obviously, one of the main benefits of public data availability is that it enables experimental reproducibility and an independent assessment of the results described in scientific publications. Particularly in the proteomics field, there have been several examples of high-profile, widely debated, controversial findings over the years.1,2 Public availability of proteomics datasets has increased exponentially in recent years, becoming a common scientific practise – as happened in other fields; for example, transcriptomics. For researchers, this change in mentality has been triggered by the stricter data availability requirements from scientific journals and funding agencies, and by the wider movement toward open science practices.
Public proteomics databases
The first MS public proteomics databases were set up around fifteen years ago. A few years later, in 2012, the most prominent resources in the field came together and started to collaborate formally, resulting in unified standard submission and data dissemination practises within the ProteomeXchange (PX) consortium.3 The PRIDE database,4 as part of the bioinformatics services offered by the European Bioinformatics Institute (EMBL-EBI, Hinxton, UK), is currently the world’s most used data repository in the field. During 2018, an average of 275 datasets were submitted to PRIDE per month (Figure 1), amounting to a total of over 10,600 ProteomeXchange datasets by February 2019. The most represented species are human and some of the main model organisms such as mouse, Arabidopsis thaliana, Saccharomyces cerevisiae, and rat. Overall, datasets from more than 1,800 different taxonomy identifiers are stored in PRIDE, including non-model organisms that have a high biotechnological interest.
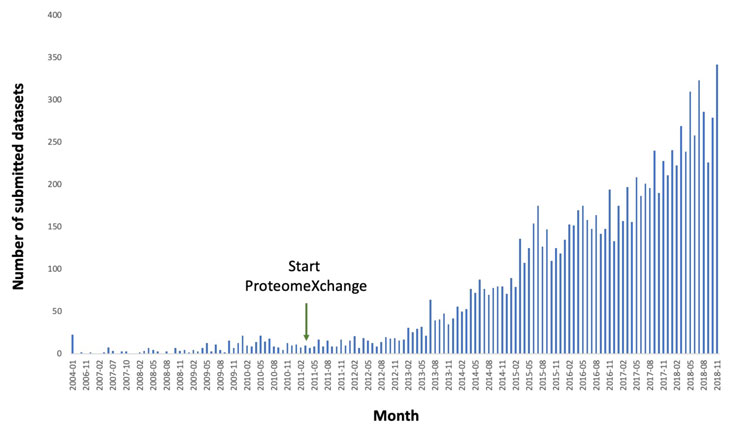
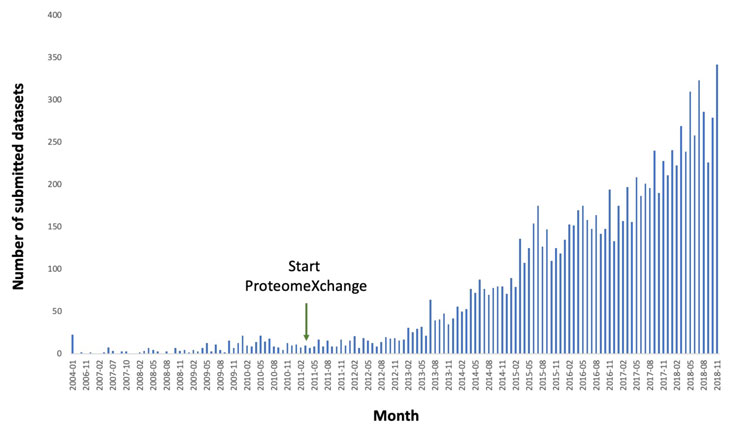
Figure 1: Number of datasets submitted per month to PRIDE from the beginning of 2004 until November 2018, the month with the highest number of submitted datasets so far. The start of the ProteomeXchange Consortium is also indicated.
At the time of writing, in addition to PRIDE, the following six resources are also part of ProteomeXchange: PeptideAtlas and PASSEL,5,6 MassIVE and Panorama Public7 (USA), jPOST8 (Japan), and iProX9 (China). For those less familiar with the field, PRIDE (and other PX resources) usually provide an analogous functionality to what is offered by widely used resources in transcriptomics such as the Gene Expression Omnibus (GEO, NCBI) and ArrayExpress (EMBL-EBI).
Use, reuse, reprocess, repurpose
Systematic public data reuse in transcriptomics has been the norm for a long time, both in academia and in industry.10 Data reuse of public proteomics datasets is now flourishing and increasingly being used for multiple applications. There are two detailed review articles focused on this topic.11,12 In these, we established four categories for public proteomics data use: use, reuse, reprocess, and repurpose. A simple example of the direct use of data is provided by connecting information between proteomics data resources (those included in ProteomeXchange) and protein knowledgebases, such as UniProt (https://www.uniprot.org/). One area of intense interest is the annotation of post-translational modifications (PTMs) such as phosphorylation, which are currently under-represented in UniProt. In fact, it is important to highlight that proteomics approaches provide the unique means to detect protein PTMs.
In the case of reuse, data is not only linked, but also reused in novel experiments, with the potential of generating new knowledge. One generic type of data reuse, also popular in other disciplines, involves analysing data from a large number of independent datasets in combination – a so-called meta-analysis study, to obtain new knowledge not accessible from any single dataset. Although there are some examples of this type of study in proteomics,13,14 they remain relatively scarce. However, in this context, “big data” approaches such as machine and deep learning methodologies constitute promising tools that are increasingly applied to large datasets, in order to significantly improve data analysis approaches. Additionally, there are multiple examples of reuse of public datasets for benchmarking purposes, facilitating the development of improved software analysis tools.
In the case of reprocess, data are reanalysed to provide an updated view of the results as protein sequence databases and analysis software evolve. Such studies, also common in other disciplines, have the same or similar goal as the original experiment, but can deliver novel findings. Resources such as PeptideAtlas routinely reprocess many datasets using their dedicated bioinformatics tools and pipelines, reusing data from single species proteomes (eg, human, mouse, pig, among many others), or sub-proteomes (eg, human plasma).
Finally, repurposing includes those cases where the datasets are reused in a context that is different to the original experiment. Proteogenomics approaches represent one attractive application of this type. In proteogenomics, proteomics data is combined with genomics and/or transcriptomics information, typically by using sequence databases generated from DNA sequencing efforts, RNA-Seq experiments, or Ribo-Seq approaches.15 Proteogenomics benefits greatly from the availability of public datasets, given the likelihood that the number of novel events detected in a single dataset (eg, novel splice junctions, small Open Reading Frames, etc) will be very small compared to the data acquisition effort. Indeed, an increasing number of studies have been performed where public data have been repurposed with this aim.16,17
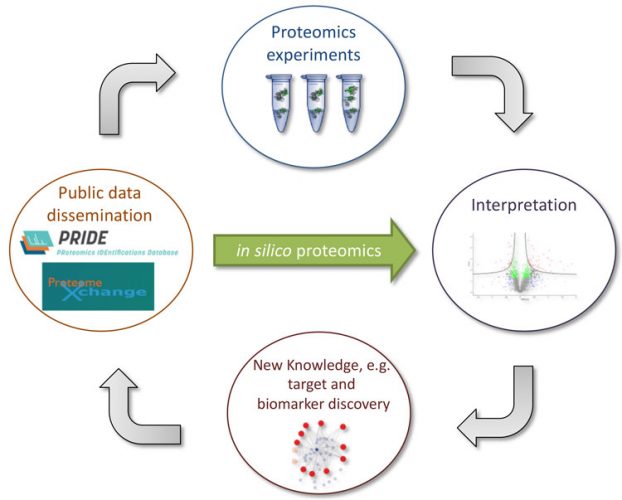
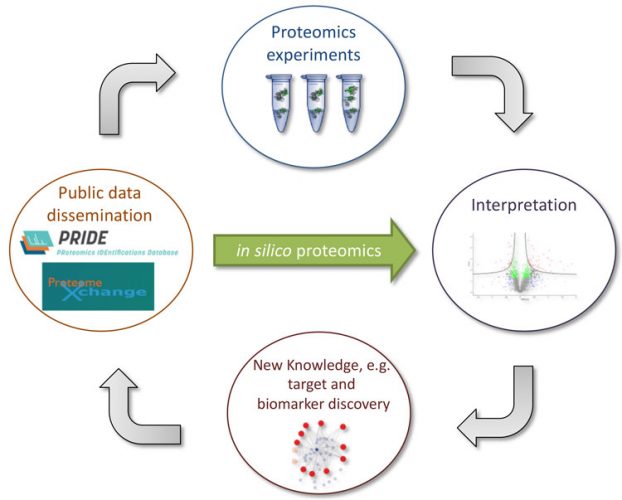
Figure 2: The rapidly growing amount of publicly-available proteomics data in the PRIDE database and the rest of the ProteomeXchange resources opens up the opportunity for in silico proteomics, instead of requiring the generation of new experimental data. (Figure adapted from Vaudel et al11)
Proteogenomics approaches also highlight the fact that proteomics approaches are increasingly becoming parts of wider multi-omics studies; for example, in the context of personalised medicine approaches. Indeed, open data facilitates integration of proteomics datasets to study, for example, the effects of genetic variation on protein function and the subsequent identification of driver genes in cancer.18 In this case, it is often not trivial for researchers to connect public datasets that have been generated in multi-omics studies, since they are stored in different public resources, which are seldom cross-linked. The Omics Discovery Index (OmicsDI) is an EMBL-EBI portal that enables researchers to discover and access datasets from different omics approaches and online resources.19
Importance of open data standards
In the context of open science policies, another key movement in proteomics has been the development of open standard file formats under the auspices of the Human Proteome Organization (HUPO) Proteomics Standards Initiative (PSI), which was originally set up in 2002. The availability of data standards is crucial to public data sharing in general, and such standards are therefore instrumental to the advancement of any research field. In addition to data formats, the PSI has also developed standardised minimum information specifications, controlled vocabularies,20 and open source software tools able to handle the data standards. The formats and guidelines are subjected to a formal review procedure called the PSI Document Process.21 At its conclusion, the implementation of open-source software supporting the data standards is promoted and encouraged. Updated detailed information about the current PSI products is available in a recent review.22 The most widely adopted MS-related data standards are mzML (for MS data, the output of the mass spectrometers),23 and mzIdentML (for the output of the identification analysis).24 Most instrument and software vendors have widely implemented mzML and mzIdentML, demonstrating the demand for users to be able to work with open data standards. Although other alternatives are becoming available, one of the main advantages of using mzML is avoiding the dependence on the Microsoft WindowsR operating system. As also mentioned, there is a collection of open source software libraries and tools that enable researchers to read and write the PSI standards (22). Updated information about current implementations can be accessed at http://www.psidev.info/mzML and http://www.psidev.info/tools-implementing-mzidentml. Additionally, other PSI file formats are increasingly used, such as mzTab, highlighting (as is also the case for mzML) that proteomics data formats can be extended to represent MS metabolomics data as well.
Conclusions
A plethora of public proteomics datasets is now available courtesy of resources such as EMBL-EBI’s PRIDE database and other ProteomeXchange resources. These datasets are increasingly reused for multiple applications, which contribute to improving our understanding of cell biology through proteomics data. These studies can only become more popular, for instance in the context of big data approaches. As technological and analytical approaches also improve, these data will be invaluable tools for drug discovery and the development of personalised medicine.
To finalise, interested parties in PRIDE-related developments can follow the PRIDE Twitter account (@pride_ebi). For regular announcements of all new publicly-available datasets, users can follow the PX Twitter account (@proteomexchange).
Acknowledgements
I would like to thank Oana Stroe and Jessica Vamathevan for their useful suggestions regarding the content of this manuscript. And The Wellcome Trust for grant number 208391/Z/17/Z.
Biography
Dr Juan Antonio Vizcaíno is the Proteomics Team Leader at the EMBL-European Bioinformatics Institute (EMBL-EBI, Cambridge, UK). His team is responsible of the development and maintenance of the PRIDE database. In addition, he is coordinating the ProteomeXchange Consortium, aiming to standardise data submission and dissemination in proteomics resources worldwide. He has also heavily contributed to the development of proteomics data standard formats (mzIdentML, mzQuantML, mzTab, proBed, proBAM) and related software. Overall, he has published more than 110 articles with over 9,400 citations.
References
- Asara JM, Schweitzer MH, Freimark LM, Phillips M, Cantley LC. Protein sequences from mastodon and Tyrannosaurus rex revealed by mass spectrometry. Science. 2007;316(5822):280-5.
- Ezkurdia I, Vazquez J, Valencia A, Tress M. Analyzing the first drafts of the human proteome. Journal of proteome research. 2014;13(8):3854-5.
- Deutsch EW, Csordas A, Sun Z, Jarnuczak A, Perez-Riverol Y, Ternent T, et al. The ProteomeXchange consortium in 2017: supporting the cultural change in proteomics public data deposition. Nucleic acids research. 2017;45(D1):D1100-D6.
- Perez-Riverol Y, Csordas A, Bai J, Bernal-Llinares M, Hewapathirana S, Kundu DJ, et al. The PRIDE database and related tools and resources in 2019: improving support for quantification data. Nucleic acids research. 2019;47(D1):D442-D50.
- Deutsch EW, Lam H, Aebersold R. PeptideAtlas: a resource for target selection for emerging targeted proteomics workflows. EMBO reports. 2008;9(5):429-34.
- Farrah T, Deutsch EW, Kreisberg R, Sun Z, Campbell DS, Mendoza L, et al. PASSEL: the PeptideAtlas SRMexperiment library. Proteomics. 2012;12(8):1170-5.
- Sharma V, Eckels J, Schilling B, Ludwig C, Jaffe JD, MacCoss MJ, et al. Panorama Public: A Public Repository for Quantitative Data Sets Processed in Skyline. Mol Cell Proteomics. 2018;17(6):1239-44.
- Okuda S, Watanabe Y, Moriya Y, Kawano S, Yamamoto T, Matsumoto M, et al. jPOSTrepo: an international standard data repository for proteomes. Nucleic acids research. 2017;45(D1):D1107-D11.
- Ma J, Chen T, Wu S, Yang C, Bai M, Shu K, et al. iProX: an integrated proteome resource. Nucleic acids research. 2019;47(D1):D1211-D7.
- Rung J, Brazma A. Reuse of public genome-wide gene expression data. Nat Rev Genet. 2013;14(2):89-99.
- Vaudel M, Verheggen K, Csordas A, Raeder H, Berven FS, Martens L, et al. Exploring the potential of public proteomics data. Proteomics. 2016;16(2):214-25.
- Martens L, Vizcaino JA. A Golden Age for Working with Public Proteomics Data. Trends Biochem Sci. 2017;42(5):333-41.
- Klie S, Martens L, Vizcaino JA, Cote R, Jones P, Apweiler R, et al. Analyzing large-scale proteomics projects with latent semantic indexing. Journal of proteome research. 2008;7(1):182-91.
- Griss J, Perez-Riverol Y, Lewis S, Tabb DL, Dianes JA, Del-Toro N, et al. Recognizing millions of consistently unidentified spectra across hundreds of shotgun proteomics datasets. Nat Methods. 2016;13(8):651-6.
- Nesvizhskii AI. Proteogenomics: concepts, applications and computational strategies. Nat Methods. 2014;11(11):1114-25.
- Volders PJ, Anckaert J, Verheggen K, Nuytens J, Martens L, Mestdagh P, et al. LNCipedia 5: towards a reference set of human long non-coding RNAs. Nucleic acids research. 2019;47(D1):D135-D9.
- Olexiouk V, Van Criekinge W, Menschaert G. An update on sORFs.org: a repository of small ORFs identified by ribosome profiling. Nucleic acids research. 2018;46(D1):D497-D502.
- Mertins P, Mani DR, Ruggles KV, Gillette MA, Clauser KR, Wang P, et al. Proteogenomics connects somatic mutations to signalling in breast cancer. Nature. 2016;534(7605):55-62.
- Perez-Riverol Y, Bai M, da Veiga Leprevost F, Squizzato S, Park YM, Haug K, et al. Discovering and linking public omics data sets using the Omics Discovery Index. Nat Biotechnol. 2017;35(5):406-9.
- Mayer G, Montecchi-Palazzi L, Ovelleiro D, Jones AR, Binz PA, Deutsch EW, et al. The HUPO proteomics standards initiative- mass spectrometry controlled vocabulary. Database (Oxford). 2013;2013:bat009.
- Vizcaino JA, Martens L, Hermjakob H, Julian RK, Paton NW. The PSI formal document process and its implementation on the PSI website. Proteomics. 2007;7(14):2355-7.
- Deutsch EW, Orchard S, Binz PA, Bittremieux W, Eisenacher M, Hermjakob H, et al. Proteomics Standards Initiative: Fifteen Years of Progress and Future Work. Journal of proteome research. 2017;16(12):4288-98.
- Martens L, Chambers M, Sturm M, Kessner D, Levander F, Shofstahl J, et al. mzML–a community standard for mass spectrometry data. Mol Cell Proteomics. 2011;10(1):R110 000133.
- Vizcaino JA, Mayer G, Perkins S, Barsnes H, Vaudel M, Perez-Riverol Y, et al. The mzIdentML Data Standard Version 1.2, Supporting Advances in Proteome Informatics. Mol Cell Proteomics. 2017;16(7):1275-85.