Advancing high content analysis towards improving clinical efficacy
Posted: 16 February 2011 |
High-content analysis is primed to play a prominent role in a new era of drug discovery research that places greater emphasis on clinical translation at all stages of the discovery process from target identification to proof-of-concept testing. High content analysis provides a technical bridge between reductionist targetdirected drug discovery approaches and new technologies that embrace the biological diversity of human disease.
The drug discovery industry is evolving rapidly, this evolution is stimulated by two key factors; (i) increased accessibility of new technologies such as next generation sequencing, systems biology and imaging that enhance our ability to interrogate complex biological systems and; (ii) the perceived failure of the widely adopted target directed drug discovery operating model to deliver novel medicines. Thus, high content imaging technologies provide a timely, pragmatic solution that enhances the effectiveness of conventional target-directed chemical approaches and provides the necessary biological context for understanding proteomic or genetic signatures. However, the future success of high-content analysis in improving the clinical success rates of drug discovery projects is entirely dependent upon the physiological relevance of the biological models under evaluation.
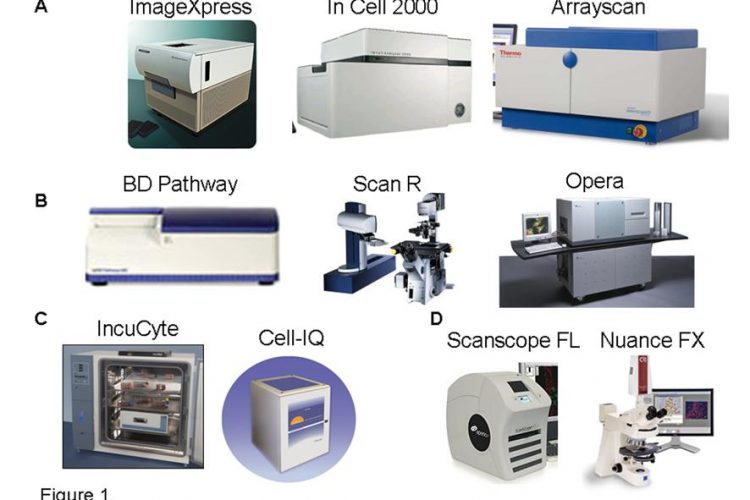
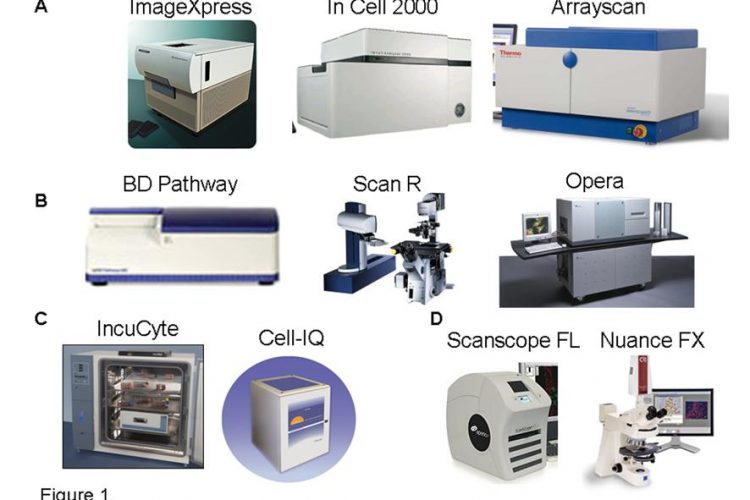
Figure 1 High Content Imaging Technology. The leading fluorescent high content imaging platforms established within the pharmaceutical and biotechnology industry. Represented are; A. Fully automated high throughput high content imaging systems (ImageXpress, InCell, BD Pathway, ScanR, Opera and the Arrayscan). B. Live cell kinetic imaging systems (IncuCyteFLR and Cell-IQ) and C. Fluorescent tissue slide imaging platform (Scanscope FL)
High-content analysis is primed to play a prominent role in a new era of drug discovery research that places greater emphasis on clinical translation at all stages of the discovery process from target identification to proof-of-concept testing. High content analysis provides a technical bridge between reductionist targetdirected drug discovery approaches and new technologies that embrace the biological diversity of human disease.
The drug discovery industry is evolving rapidly, this evolution is stimulated by two key factors; (i) increased accessibility of new technologies such as next generation sequencing, systems biology and imaging that enhance our ability to interrogate complex biological systems and; (ii) the perceived failure of the widely adopted target directed drug discovery operating model to deliver novel medicines. Thus, high content imaging technologies provide a timely, pragmatic solution that enhances the effectiveness of conventional target-directed chemical approaches and provides the necessary biological context for understanding proteomic or genetic signatures. However, the future success of high-content analysis in improving the clinical success rates of drug discovery projects is entirely dependent upon the physiological relevance of the biological models under evaluation.
Challenges in drug discovery
The challenge of providing new medicines for the most common disease syndromes afflicting modern society including cancer, neurodegeneration and chronic obstructive pulmonary disorder (COPD) have never been so critical for the drug discovery industry. Despite significant scientific and technical advances over the past two decades, including the identification of an unprecedented number of potential new drug targets through genome sequencing, increased research and development (R&D) investments have not provided the anticipated return of more novel and more effective drugs. Indeed, a grave concern to all associated with the drugdiscovery industry is that increasing investments in R&D and enhanced information of human disease at genetic and proteomics levels have coincided with a decline in the number of new drugs reaching the market. Such a disconnect between technology advances, enhanced understanding of disease mechanisms with the discovery of new effective medicines implies that current drug discovery strategies are sub-optimal. While advances in combinatorial chemistry and high throughput screening have undoubtedly improved the quality of novel agonist and antagonists on the basis of potency and selectivity, high attrition of candidate drugs in the later stages of preclinical development or in clinical trials prevails. Attrition resulting from poor efficacy is particularly acute in complex diseases where underlying disease mechanisms are heterogeneous within and between individual patient groups, or where mechanisms can adapt to therapeutic treatment. Consequently, high throughput target-directed drug discovery strategies, on their own, no longer represent an attractive business model and need to be refined1,2. The scientific challenges of discovering effective drugs for the most complex of diseases are exacerbated by rigorous regulatory standards, widespread use of relatively inexpensive established medicines and restrictions on costs of new medicine, imposed by healthcare providers and payers. In response to such challenges, the pharmaceutical and biotechnology industries are actively revising their R&D strategies and placing increased emphasis on translational sciences and more innovative paradigms that complement and challenge the widely adopted targetdirected drug discovery approaches.
High content ambitions
Emerging technologies such as next generation sequencing, systems biology and advances in in vitro and in vivo imaging are rapidly becoming accepted as valuable tools for incorporating complex biological knowledge back into the drug discovery process. In contrast to sequencing and computational technologies, microscopic cellular imaging has an established role in the history of scientific research and was the foundation technology in the biological evaluation of many early generation medicines. Traditionally, imaging been applied subjectively to biology systems through small scale manual endeavours that limit both the quality and throughput of the biological evaluation. However, the design of automated fluorescent microscope systems and associated image analysis algorithms, suitable for quantifying several features across multiple cells in multi-well plates, has created the new discipline of high-content cellular imaging. High-content analysis describes the quantitative analysis of multiparametric features extracted from fluorescent or brightfield images of cells in an automated fashion. High content imaging microscopes and image analysis tools have advanced rapidly over the past 10 years with the design of more efficient, user friendly platforms that facilitate and expand both endpoint and live-cell studies (Figure 1). High content analysis technologies have now matured and become established in drug discovery as evidenced by a shift in focus from technology development to new biological applications3.
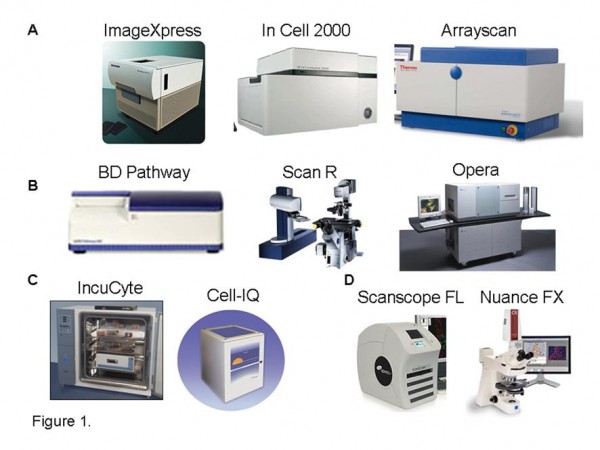
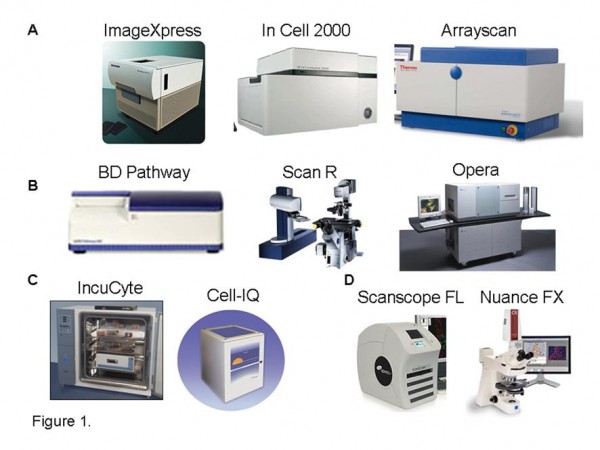
Figure 1 High Content Imaging Technology. The leading fluorescent high content imaging platforms established within the pharmaceutical and biotechnology industry. Represented are; A. Fully automated high throughput high content imaging systems (ImageXpress, InCell, BD Pathway, ScanR, Opera and the Arrayscan). B. Live cell kinetic imaging systems (IncuCyteFLR and Cell-IQ) and C. Fluorescent tissue slide imaging platform (Scanscope FL)
The adoption of high content analysis by the pharmaceutical and biotechnology industry indicates a willingness to incorporate more complex biological endpoints into early phase drug discovery projects. High content screening is typically placed as a secondary screening strategy to confirm and/or stratify hits identified from high throughput screening (HTS) campaigns. However, several examples of where high-content analysis has been placed as a primary HTS are becoming more common4. A significant advantage of placing high-content analysis at the earliest phase of drug discovery is demonstrating proven activity within complex cellular environments providing higher quality target validation and hit compounds. It has been proposed that the application of high content analysis may reduce the high attrition of compounds observed at late stages in preclinical and clinical development by frontloading the evaluation of chemical libraries in complex biological systems. The clinical predictivity of high-content screens, however, remains to be determined and is solely dependent upon the physiological relevance of the biological models and endpoints under evaluation. Advances in the design of fluorescent reporter molecules based on natural proteins (green fluorescent protein; GFP) and engineered spectrally distinct variants expressed in cell lines have contributed to the increased application, throughput and adoption of high content analysis5. However, the ability of engineered reporter cell lines to accurately recapitulate complex human disease pathophysiology and heterogeneity is limited.
A constraint of many pre-processed image analysis algorithms that accompany high content analysis platforms is their limitation to cell lines, endpoints and 2D culture systems amenable to basic cell segmentation and object definition. Typically, large scale high-content screens and development of multivariate high content analysis approaches have been applied to common immortalised cell lines such as Hela or U2OS cells cultured on two dimensional substrates. Comments on the quality of high content analysis approaches and their impact upon drug discovery to date must take into account the relevance of the biological models under evaluation. Recent advances in the development of increasingly sophisticated context based image analysis software solutions and machine learning approaches enable the design of bespoke image analysis algorithms that can be tailored to more complex biological models6,7. Such adaptive image analysis approaches can be applied to leverage quantitative information from complex 2D or 3D biological models incorporating heterogeneous cell populations or co-cultures derived from cell lines or primary cell isolates (Figure 2). The development of more flexible high content analysis approaches are an ideal companion technology for emerging stem cell research technologies by facilitating both the identification of compounds that direct stem cell fate and the integration of human embryonic and induced pluripotent stem cells into more relevant cell models8. Thus, the development of bespoke image analysis algorithms may prove to be more significant than the development of high-content instrument hardware. The flexibility offered by new image analysis solutions can stimulate the design and expand the diversity of more ambitious experimental models that more faithfully represent disease mechanisms. It is unlikely that a single high content assay shall appropriately integrate all of the necessary biological mechanisms associated with complex diseases to inform clinical translation of experimental finding. Rather, detailed phenotypic profiling of drug candidates across a suite of high content assays monitoring multiple and distinct biological processes implicated in disease can paint a more informative picture of drug responses in complex disease settings. The design and understanding of such relevant high-content assays to enable effective decision making in the drug discovery process requires careful consideration and retrospective evaluation. Retrospective studies of successful and failed drugs already progressed into the clinic shall determine which muliparametric signatures extracted from high-content assays link to clinical outcome. Similarly, the worth of more complex 3D, co-culture, spheroid or organotypic models can be compared with standard 2D culture approaches through retrospective analysis to determine what additional value in terms of clinical prediction can be gained.
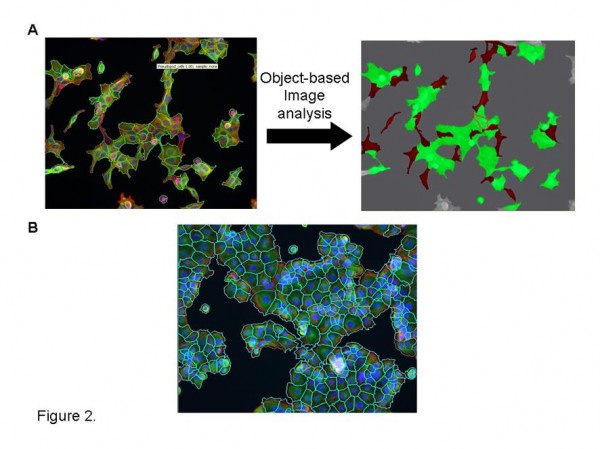
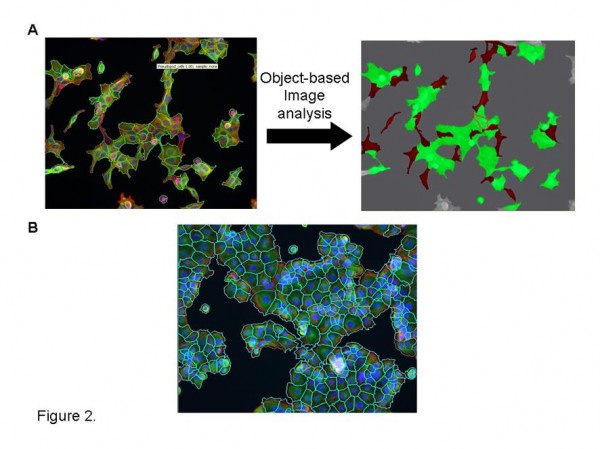
Figure 2 Bespoke image analysis solutions. A. Application of Definiens object based image analysis software to segment morphologically distinct cells from heterogenous cultures for quantitative analysis. Cells masked in red indicate significant pseudopod formation. B. Effective cell segmentation of challenging cell cultures utilizing cytoplasmic markers (cell mask –Invitrogen; phalliodin labelled F-actin -Sigma) and Definiens multiparametric image analysis algorithm
High content analysis typically refers to the analysis of in vitro cellular assays, however, technical advances in imaging technology, optical reporter molecules, image analysis algorithms and laboratory consumables now enable the application of high content analysis to both primary patient derived samples and complex in vivo models. As high content biology is essentially a single-cell analysis tool, highcontent analysis can be robustly applied to small numbers of cells including those directly isolated from patient samples. High content analysis of primary cells can be facilitated by the design of specialised consumables such as lab-on-chip, magnetic sorting or microfluidic devices9 that capture and discriminate between small numbers of cells to permit effective recording of disease and drug response phenotypes, directly upon patient samples. Incorporating patient samples into preclinical drug discovery strategies combines the personalised healthcare approach with the ability to monitor multiple perturbations to identify more optimal therapeutic strategies and reliable biomarkers of drug response.
The majority of in vivo models employed to study pharmaceutical responses are long standing rodent models incorporating traditional endpoints such as tumour volume, inflammatory responses and behavioural tests. In a similar vein to high throughput screens, preclinical testing is often applied to in vivo models and endpoints that are designed for throughput and speed rather than physiological relevance or detailed mechanistic analysis of drug response. New transgenic models of disease have been developed that recapitulate the genetic components of human disease in appropriate tissue microenvironments that better recapitulate human pathophysiology and drug response10. Advances in the sensitivity of multiphoton microscopes, combined with the cellular resolution provided by intravital imaging techniques enable quantitative imaging of cells and dynamic molecular processes directly in live in vivo environments11. Through incorporation of fluorescently labelled proteins into transgenic animal design complemented with the application of spectroscopic techniques such as, fluorescence resonance energy transfer (FRET), fluorescence lifetime imaging (FLIM), photoacativation and photobleaching, a wide variety of cellular processes and primary endpoints associated with disease can be recorded at tissue, cellular and subcellular levels in vivo. Application of these techniques to live animals provides additional kinetic information, enabling detailed functional analysis of dynamic processes ranging from cell tracking to cell death, adhesion dynamics and enzyme kinetics12. Dynamic intravital imaging provides an almost immediate readout of drug response thereby accelerating the evaluation of drug response in vivo and eliminating the need for extensive histopathological examination of mechanistic biomarkers. Further development of intravital high content analysis approaches that monitor in vivo tissue and cellular phenotypes in live animals are likely to represent wise drug discovery investments, by reducing the cost and number of in vivo studies while expanding the in vivo evaluation of drug response.
Current approaches for diagnosis and patient stratification utilising patient tissue samples are typically univariate, semiquantitative analysis of candidate tissue markers by standard immunohistochemistry (IHC) approaches. The additional spatial and functional information obtained by quantitative tissue imaging can offer more predictive power to prognostic evaluation and drug response biomarkers, in addition to providing further insight into disease mechanism13. Further advances in in vivo and ex-vivo imaging technologies, including more powerful multiphoton lasers, increased sensitivity and utility of microscopes and camera systems including multispectral imaging capabilities (Figure 3) shall promote the application of high content analysis to in vivo models and clinical tissue samples. The incorporation of image-based profiling of complex biological models and clinical samples signal a return to historical research values where detailed physiological evaluation of disease biology in vivo underpins drug development.
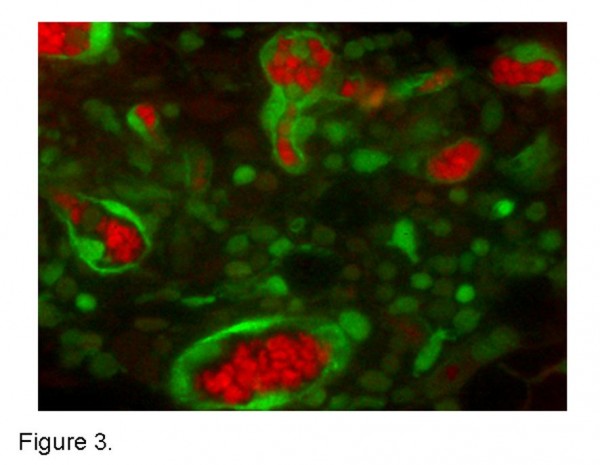
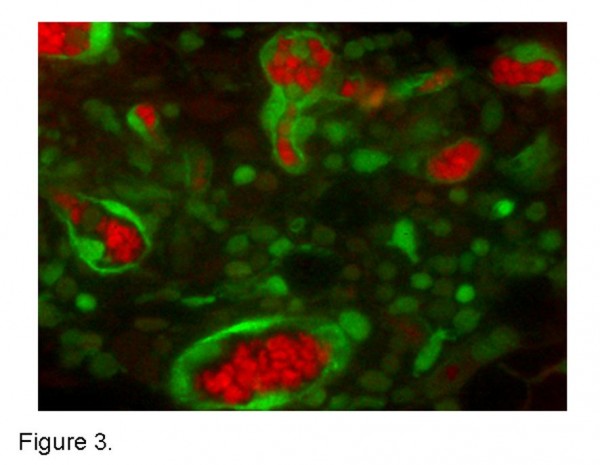
Figure 3 Multispectral tissue imaging. A spectrally unmixed tumour tissue slice from a subcutaneous implantation of unlabelled MC38 adenocarcinoma cells transplanted into a GFP transgenic mouse. Haemoglobin containing red blood cells (red) and GFP labelled host inflammatory and endothelial cells (green) unmixed with a Nuance multispectral microscope and associated software
Future challenges and opportunities
Recent advances in quantitative high-content imaging technology and multiparametric image analysis methods expand the scope, interrogation and application of complex experimental models for drug discovery. Incorporation of innovative image analysis algorithms and multivariate statistical methods has demonstrated added value over conventional strategies by identifying novel compound mechanism-of-action and structure activity relationships (SAR) based upon phenotypic response14-16. Further development of automated data analysis workflows that incorporate advanced multiparametric image analysis procedures empowers and challenges biologists to apply high content approaches to more sophisticated and relevant biological models17. However, the application of high content approaches to more complex biological systems and primary derived material negatively impacts on the throughput of such technologies. Thus, a fundamental shift from the high number-high attrition model that feeds target-directed strategies to more focused applications is necessary for complex high content biology programs. The application of high content approaches to smaller focused chemical libraries representing distinct chemical series or target selectivity profiles can be used iteratively to optimise chemical design based on phenotypic SAR. Advances in transcriptomics, proteomics and bioinfomatics approaches can also aid prioritisation of target selection and optimal compound selectivity profiles for high content evaluation.
High content analysis represents a unique technology platform that provides detailed biological and functional context which complements and enhances other emerging technologies (next generation sequencing, quantitative proteomics, biomarker diagnostics, RNAi library profiling, stem cell technology, systems biology) (Figure 4). However, the true value of high content analysis in improving the efficiency of the drug discovery process will only be realised following demonstrative success in contributing to better informed clinical positioning of new effective drug therapies. The development of advanced models of disease and validation of clinical translation of such models will require closer collaboration between the industrial sector, academic groups and clinical researchers. High content analysis may again provide a bridge by linking the professional goals of academics, healthcare workers and the pharmaceutical/ biotechnology industry. Target agnostic applications of high content analysis including drug reprofiling, evaluation of novel drug combination and scheduling strategies may appeal to clinicians by providing new therapeutic approaches with a faster route to the clinic. Similarly, for academic researchers, high content analysis provides an ideal companion technology for RNAi and ‘omics’ approaches, that probe the complexties of cell biology. For pharmaceutical, biotechnology and contract research organisations, collaboration with academics and clinicians who can provide valuable clinical samples, pre-validation of sophisticated in vivo and in vitro experimental models, maximises the investment that many companies have already made in high content analysis and image informatics. A new era of knowledge-driven drug discovery is dawning where high content technologies and skills will be centrally placed, when used in conjunction with valued clinical samples and experimental models of disease.
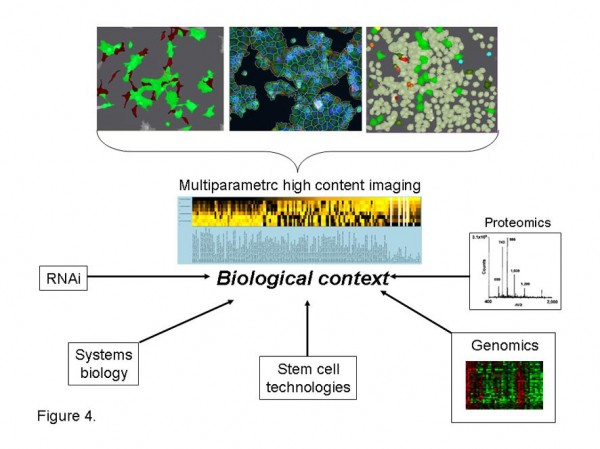
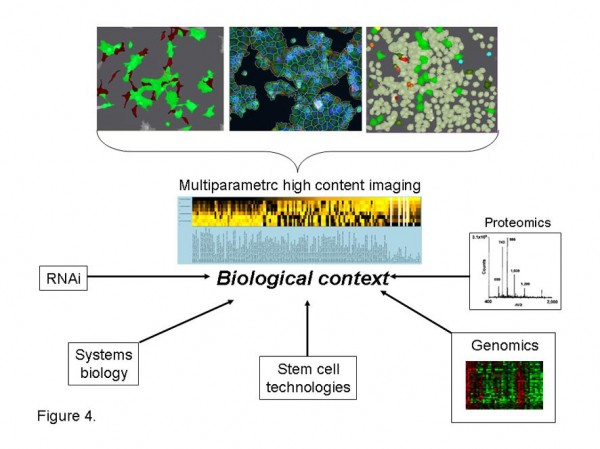
Figure 4 Multiparametric high content analysis. Multiparametric high content imaging of in vitro assays, in vivo models and clinical samples provides functional quantification of cellular and subcellular biology with spatial context unrivalled by other technologies. Multiparametric analysis of biological processes provides detailed phenotypic analysis of complex biological systems providing the necessary biological context to interpret genomic and proteomic signatures and RNAi library screens. Multiparametric high content analysis further facilitates stem cell differentiation studies and provides valuable phenotypic inputs into computational simulations and validation of systems biology algorithms
Acknowledgements
The author wishes to thank all of the staff at the Advanced Science and Technology Laboratory within AstraZeneca for their support and contribution to the findings and data presented in this article.
References
1. Sams-Dodd F. (2005). Target-based drug discovery: is something wrong? Drug Discov Today. 10, 139-147
2. Butcher EC. (2005). Can cell systems biology rescue drug discovery. 4, 461-467
3. Zock JM. (2009). Applications of High content screening in life science research. Combinatorial Chemistry & high throughput screening. 12, 870-876
4. Alcock P, Bath C, Blackett C and Simpson PB (2010). High content cell based primary screening for oncology targets – a perspective. 3. June 25
5. Ting AY, Kain KH, Klemke RL and Tsien RY (2001). Genetically encoded fluorescent reporters of protein tyrosine kinase activities in living cells. Proc Natl. Acad. Sci. USA 97, 2253-258
6. Baatz M, Arini N, Schape A, Binnig G and Linssen B (2006). Object-orientated image analysis for high content screening: detailed quantification of cells and sub cellular structures with the cellenger software. Cytometry Part A. 69:652–658
7. Jones TR, Kang IH, Wheeler DB, Lindquist RA, Papallo A, Sabatini DM and Golland P (2008). BMC Bioinformatics.Nov 15;9:482
8. Barbaric I, Gokhale PJ and Andrews PW (2010) High-content screening of small compounds on human embryonic stem cells. Biochem. Soc. Trans. 38(4), 1046-1050
9. Berthier E Surfus J, Verbsky J, Huttenlocher A and Beebe D (2010). An arrayed high-content chemotaxis assay for patient diagnosis. Integr. Biol. 2 630-638
10. Olive KP, Jacobetz MA, Davidson CJ, Gopinathan A, McIntyre D, Honess D, Madhu B, Goldgraben MA, Caldwell ME, Allard D, Frese KK, Denicola G, Feig C, Combs C, Winter SP, Ireland-Zecchini H, Reichelt S, Howat WJ, Chang A, Dhara M, Wang L, Rückert F, Grützmann R, Pilarsky C, Izeradjene K, Hingorani SR, Huang P, Davies SE, Plunkett W, Egorin M, Hruban RH, Whitebread N, McGovern K, Adams J, Iacobuzio- Donahue C, Griffiths J, Tuveson DA(2009). Inhibition of Hedgehog signaling enhances delivery of chemotherapy in a mouse model of pancreatic cancer. Science. 12;324(5933):1400-1
11. Wang W, Goswami S, Sahai E, Wyckoff JB, Segall JE and Condeelis JS (2005). Tumor cells caught in the act of invading: their strategy for enhanced cell motility. Trends Cell Biol 15: 138-145
12. Serrels A, Timpson P, Canel M, Carragher NO, Frame MC, Brunton VG, Anderson KI. (2009). Comparison of cell adhesion and membrane dynamics in vitro and in living mice using photo-activation and -bleaching. Cancer Res. 69(7):2714-9
13. Mohammed RA, Ellis IO, Elsheikh S, Paish EC, Martin SG (2008). Improved methods of detection of lymphovascular invasion demonstrate that it is the predominant method of vascular invasion in breast cancer and has important clinical consequences. Breast Cancer Res Treat. 113:261-273
14. Perlman ZE, Slack MD, Feng Y, Mitchison TJ, Wu LF and Altschuler SJ. (2004). Multidimensional drug profiling by automated microscopy. Science 004;306:1194–8
15. Tanaka M, Bateman R, Rauh D, et al. An unbiased cell morphology based screen for new, biologically active small molecules.(2005). PLoS Biol 2005;3:e128
16. Young DW, Bender A, Hoyt J, et al. (2008). Integrating high-content screening and ligand-target prediction to identify mechanism of action. Nat Chem Biol. 4:59–68
17. Caie P, Walls R, Ingleston-Orme A, Daya S, Houslay T, Eagle R, Roberts M and Carragher NO (2010). High- Content phenotypic profiling of drug response signatures across distinct cancer cells.Mol. Cancer Therap. 9(6) 1913-1926
About the Author
Neil Carragher has played an influential role in the development of innovative in vitro and in vivo imaging applications for drug discovery both from within industry and academia. He has contributed to several peer reviewed publications and international presentations on the topic of advanced imaging methodology. In 2010, he was appointed Principal Investigator at the Edinburgh Cancer Research Centre within the University of Edinburgh’s Institute of Genetics and Molecular Medicine. The focus of his research group is to advance cancer drug discovery through the application of state-of-the-art imaging approaches to complex experimental models and clinical samples. He previously held a principal scientist post at the Advanced Science and Technology Laboratory within AstraZeneca where his research team focused on the development, evaluation and implementation of new technologies to enhance the drug discovery process. Prior to AstraZeneca, Neil held two consecutive academic posts at the University of Washington in Seattle and Beatson Institute for Cancer Research in Glasgow where his research integrated molecular biology techniques with image based phenotypic analysis in several research areas. Neil obtained his PhD while at the Yamanouchi Research Institute in Oxford, England.