The case for data analytics in proof of concept studies
Posted: 26 February 2016 | | No comments yet
Here, Rodrigo Barnes, Chief Technology Officer at Aridhia, discusses how data analytics can add value to proof of concept trials…
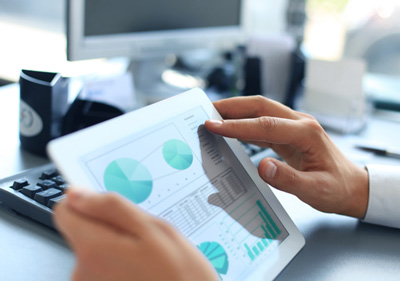
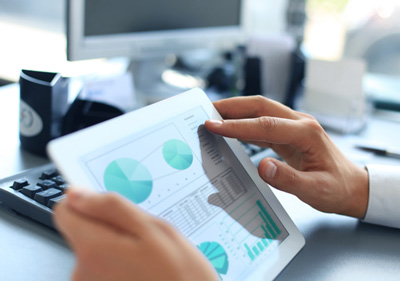
We have more data – clinical, genomic, patient generated, etc. – available than ever before, presenting a significant opportunity for innovation, particularly in precision medicine and the development of new, effective drug candidates aligned to specific patient populations.
And while we are on the verge of a healthcare revolution driven by data and technology, both healthcare delivery and pharmaceutical sectors are also facing significant financial constraints, requiring judgments to be made on what is and what is not affordable.
Clinical trials are massive investments in terms of time, capital and effort, so when a drug candidate fails to reach its expected result, the financial loss is significant. It is becoming increasingly clear that the key to a successful trial would be harnessing complex data and transformative technologies at an early stage to improve the recruitment of suitable patient cohorts into clinical trials and quickly identify drugs that have a positive therapeutic effect.
While adaptive trials are increasingly common, most notably in phase III studies, there is a strong case for using the same approach to mitigate risks during early exploratory phases in order to have a positive impact on late stage success rates. The adaptive trial methodology is therefore an increasingly attractive approach during the proof of concept stage, where safety and tolerability testing begins.
Simple adaptive trials have been shown to add value by speeding up the development of new treatments, at a fraction of the cost, and with fewer trial participants. However, along with these benefits, come challenges.
To ensure further investment and development in a drug, evidence in the form of near real-time data must be presented demonstrating safety and efficacy in human volunteers. However recent advances in precision medicine have demonstrated that a range of factors – including genetic, environmental and lifestyle – affect a patient’s response to a specific treatment. The way in which clinical trials are carried out must therefore respond to this complexity and move towards this more flexible methodology in order to not only determine safety of a drug candidate, and how it is best delivered, but also its efficacy for defined subgroups of patients.
The requirements for such flexibility brings challenges to the capture and analysis of complex data sets – including genomic, micro-sampling and imaging – and linked patient data for multiple patient cohorts throughout all stages of research. The rapid adaptation of a single patient’s study plan or the trial itself requires the collection of large volumes of clean data and timely interim analysis to be made easily available for optimum results.
If we are to routinely apply an adaptive methodology to proof of concept studies, all outcomes must be captured and reported in near real-time, and transferred to a central hub for analysis, necessitating the use of a flexible and responsive analytical environment which reflects the realities of complex decision making in a high-cost, competitive environment.
This will involve the introduction of informatics platforms, such as AnalytiXagility, capable of integrating and analysing streams of data from multiple sources in real-time, enabling critical decisions to be implemented in optimal time frames, and allowing researchers to respond rapidly to emerging results.
The cost and adoption of such advanced analytical technologies can be seen as a barrier, but with the stakes of drug development being incredibly high, and significant capital investment required to develop a new drug candidate, data-driven decision making is becoming increasingly important.
It becomes evident that to simplify drug development pipelines, and bring new treatments to market faster, the focus on building an open business model will play a central role in accelerating this work. Early adopters in this new marketplace are investing in an information platform that enables innovation to take place both at the technological and collaboration levels to increase both the probability of success and decrease development time.
New tools and methodologies, developed collaboratively, and based around advanced data analysis are required bring new treatments to the market: for example, investment in probabilistic models of disease progression or systems biology, repeatable protocols for running adaptive trials as well as trial simulations to understand potential value or harm (or simply information gain) if participants are deployed to different arms of the trial.
This high-quality data analysis undoubtedly adds value to proof of concepts trials, and by leveraging groundbreaking technology, and adding greater collaboration to these studies, we can implement more effective, data-driven proof of concept trials undertaken in near real-time.
By using a safe and transparent analytics technology or platform, advancements in patient care will be made, giving us the ability to better identify the most effective care pathways for patients, and dramatically improve care management, as well as less risky and more profitable research and development.