Novel methodologies for determining the mineral content of complex multivitamin tablets
Posted: 5 September 2014 | | No comments yet
Pharmaceutical product manufacturing is a conservative environment because of the obligations to abide by rigorous operation protocols aimed at insuring the highest possible product quality. A relatively recent initiativeguidance, from the U.S. FDA (Food and Drug Administration) has encouraged innovation and development of PATs (Process Analytical Technologies) for improved process efficiency. This has created opportunities for technological development and application of available technologies.
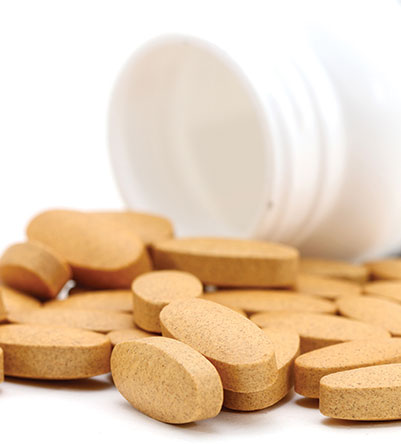
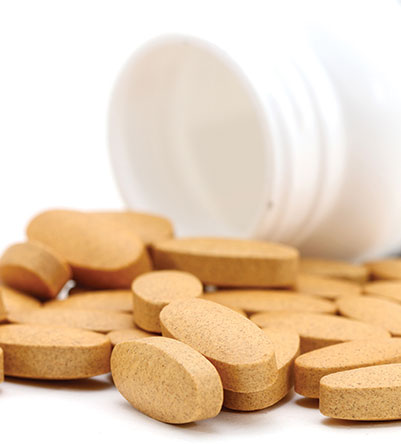
A well-established and widely-used method for quantitative elemental analysis is ICP-AES (Inductively Coupled Plasma – Atomic Emission Spectroscopy). Although powerful and efficient, this technology depends on many consumables and has several drawbacks, such as sample preparation requiring long acid digestions, which poses health and environmental risks. Many alternative spectroscopic techniques have been developed for elemental quantification, and could potentially replace ICP-AES. This is the case of LIBS (Laser Induced Breakdown Spectroscopy) developed over the last 50 years and XRF (X-Ray Fluorescence), which has been used in the mining and metallurgy industry for many years. Furthermore, LIBS has already been present for some time in pharmaceutical research for its potential for 3-D profiling due to its micro-destructive nature6. Tablet coating thickness and uniformity has also been assessed using this technology by applying multiple consecutive laser shots to a tablet until its core’s spectral signature is revealed. Many other LIBS efforts applied to the pharmaceutical industry were for the classification9 and quantification of minerals in pharmaceuticals. These papers show that LIBS has proven effective for microscopically homogeneous samples, but the work done on microheterogeneous complex samples, such is the case for pharmaceutical multivitamin tablets is not as common.
Likewise, XRF is another elemental analysis technology in which pharmaceutical research has increasing interest. Several research efforts demonstrate its applicability for the identification of the presence of impurities in pharmaceutical tablets13,14. Micro-confocal XRF was used to study elemental distributions in tablets, up to a few hundred microns deep15. Contrarily to other industry branches and activities that have lower scrutiny levels, such as Food & Nutrition, Mining & Solid Engineering, few quantification applications of this technology in a pharmaceutical context are presented in the literature.
The objective is to assess the potential of XRF and LIBS for at-line mineral quantification in multivitamin tablets. Ten elements, varying in concentration from traces to major components (< 10 ppm to > 30%), are targeted in this study: Mg, K, Ca, V, Cr, Mn, Fe, Cu, Zn and Mo. Methodology includes the use of univariate and multivariate analysis techniques.
Material and methods
LIBS instrument
LIBS analysis is based on plasma emission spectroscopy and consists of ablating a sample with a high-powered laser. The resulting plasma condenses and emits light that is characteristic of the sample elemental composition. This light is collected with an optical fibre and the signal is transferred via appropriate optical hardware for conversion into a spectrum. The LIBS instrument used for the experiments is a TSI Insight 2012 equipped with a high sensitivity gated iCCD camera (16-bit, UV-enhanced), an Echelle spectrometer (200-1000 nm range) and a 200 mJ Nd:YAG laser (1064 nm, adjustable from 0-100% laser energy). Data was analysed using both the instrument supplier software (AddLIBS) for the spectral line database and Matlab (MathWorks) codes for modelling and data treatment.
XRF instrument
XRF is a technology based on the fluorescence phenomenon. It consists of bombarding a sample with X-rays that will eject inner shell electrons of the elements present in the sample. The resulting vacant spots will be filled by outer shell electrons and will emit the energy difference of the transition quantum states as a photon. The energy of the photons is characteristic of the elements; they are collected and their energy spectrum is analysed.
The XRF instrument used for the experiments is a PANalytical Axios WD-XRF equipped with a Rh-anode Super Sharp Tube (1 kW, 20-60 kV, 16-50 mA), a DOPS goniometer, 11 interchangeable crystals, a gas flow proportional counter and scintillation detector.
Sample preparation and reference method
The tablets tested were composed of over 35 pharmaceutical ingredients with typical concentrations of commercial multivitamin tablets. For confidentiality reasons, only concentration ranges of the 10 elements of interest for this study are presented in Table 1, so:
- Major components: concentration > 1% (wt/wt)
- Minor components: 1% (wt/wt) > concentration > 0.1% (wt/wt)
- Trace components: concentration < 0.1% (wt/wt).
For XRF, the calibration dataset was composed of 11 formulations: 10 formulations prepared at lab scale and one commercial sample. For LIBS, the calibration dataset was composed of 10 formulations prepared at lab-scale. All elemental concentrations varied between 50% and 300% of commercial product label claim.
Verification sets for XRF and LIBS were composed of four formulations: three lab scale and one commercial. Lab formulations were prepared in order to obtain mineral concentrations varying in the calibration range. Formulations were homogenised using a Retsch mixer mill with a single stainless steel ball and compartment for one minute at 50% amplitude. Tablets were compressed using a manual press at 25,000 psi for approximately 45 seconds.
The chosen reference method for elemental concentrations was ICP-MS. The sampling for ICP-MS was done after tablet compression to avoid segregation caused by sample manipulation. For the formulations preparation, care was taken to minimise correlations between the various elemental concentrations. The highest correlation obtained is 0.39 between Fe and Mg which means that a low correlation is present among all minerals.
Sampling
Sampling protocols were adapted to each technology considering the varying sample sizes. Two 7g tablets of each formulation were prepared according to the sample preparation method.
For XRF testing, the two tablets were tested on both sides for a total of four samplings per formulation. In the case of LIBS, the number of shots required for significant sampling of each tablet was determined by a Monte Carlo simulation of the sampling process. The sampling template proposed is a seven (length) x six (width) x seven (depth) shot matrix.
Acquisition parameters
XRF acquisition parameters used were those suggested by the instrument provider software and varied for each element.
The optimal acquisition parameters for LIBS were determined by a full factorial experimental design (DoE) with centre points that optimised the signal-to-noise ratio for each element. The plan varied four factors: laser power, gate delay, gate width and spot size. The design results showed that all elements of interest had similar optimal parameters and thus the following were used: laser power at 75%, gate delay at 3.7µs, gate width at 15µs and spot size at 500µm.
Data analysis
A univariate analysis method was compared to a multivariate approach. The univariate analysis was done by correlating elemental peak intensities with their theoretical concentration. The highest intensity peak free from any overlapping peaks was chosen for the univariate analysis.
In the multivariate approach, PLS models were built for each element and each instrument. The number of latent variables was chosen by minimising the error of prediction while seeking to limit the number of latent variables used by the model.
For the evaluation of model performance, several criteria were used. Mean error of prediction (MEP) was calculated to assess prediction error and the determination coefficient (R2) was used to compare linearity. For multivariate models, linearity of the prediction data was also investigated. Finally, variable importance in the projection (VIP) values represent the variables that were most influent on the prediction. Thus, they contain information regarding model robustness for the analyst by linking these variables with potential cause-effect linkages. All presented p-values correspond to paired one-tailed t-test results.
XRF data analysis
Multiple XRF peaks were compiled for the analysis. All lines were chosen to maximise signal intensity without overlapping with lines of other elements in the formulation. A total of 33 XRF line intensities were collected with their respective baselines, yielding a data matrix of 29 observations x 66 variables.
LIBS data analysis
All LIBS spectra for each analysed tablet were averaged for a total of 252 samples per observation, yielding a data matrix of 29 observations x 40,002 variables. Resulting spectra were normalized for total light according to Equation 1.
var_normi=var(i)i=1nb. varsvar(i)
Equation 1: Total light normalisation equation for LIBS spectra
Where var(i) is the variable i, var_normi is the normalised variable i and nb. vars is the total number of variables in the spectrum. Following total light normalization, Standard Normal Variate (SNV) and autoscaling algorithms were applied to the data. An SNV algorithm consists in an observation-wise standardisation that divides each variable by the observation mean. Similarly, an auto-scaling consists in a variable-wise standardisation in which the variable mean is subtracted from each observation and divided by its standard deviation.
Results and discussion
XRF results
Table 2 shows XRF results obtained from univariate and multivariate analysis methods. Univariate model performance seems to be highly dependent upon elemental nature and concentration. Low concentration elements tend to have higher prediction errors since their concentrations approach the theoretical quantification limit of the equipment. On the other hand, linearity does not seem to be linked to elemental concentration with major elements like K, showing low linearity of the calibration curve.
Figure 1 a and c show examples of XRF univariate and multivariate calibration curves for typical elements with good performance results. A multivariate model has the potential to take into account some elemental interactions (i.e. matrix effects). This can be seen in the VIPs for each model and the high number of latent variables required to minimise the prediction error. The element of interest is always one of the major VIPs in the model but other spectral lines are used for the prediction; this means that there is a possible interaction between these elements. As previously explained, correlations between known elemental concentrations were minimised, limiting the possibility that these correlations are the cause of these VIP observations (some elements are of unknown concentrations in the samples i.e. Na).
Calibration data is much more linear than verification sets; this could be explained by the fact that, in many cases, concentrations in the verification set cover a narrower range. Also, fewer samples are present in the prediction set which could influence linearity.
At a confidence level of 95%, it is possible to conclude that neither of these two approaches (i.e. univariate or multivariate), seems to perform better when it comes to its prediction error (p = 0.48). In contrast, calibration curve linearity is significantly superior in multivariate models (p = 8.2E-4).
LIBS results
Results obtained with the LIBS technology are presented in Table 3. For the method, the univariate approach yields significantly inferior prediction performance when compared to the multivariate approach (p = 0.023). The same conclusions are obtained when comparing both analysis methods based on linearity (p = 9.2E-5). Nevertheless, multivariate models do not seem to be robust since most of them have VIPs based on spectral areas that have no peaks or that present peaks of other elements.
Figure 1 b and d show examples of LIBS univariate and multivariate calibration curves for typical elements with good performance results. Univariate analysis probably performs poorly due to significant matrix effects. This is plausible because there are important variations in the matrix composition from sample to sample. The matrices vary significantly in organic and inorganic compositions, whose thermal properties may influence plasma formation and emission intensity making it difficult to correlate line intensity with concentration17. For the multivariate analysis, such a high dimensionality (40,002 variables) increases the probability of having correlations between noise in the data and the reference values. This can explain why so many VIP values correspond to spectral areas that are not theoretically linked to the element in question.
In general, multivariate models perform better in the case of LIBS based on the two proposed criteria, but much improvement is needed prior to a pharmaceutical industrial application.
Overall comparison of the technologies
In order to compare both instruments, only multivariate model results will be taken into account. With an average error of prediction near 15% for XRF and close to twice that for LIBS, XRF should be considered as the better alternative for mineral quantification in pharmaceutical multivitamin tablets (p = 0.038). Moreover, calibration models tend to be much more linear with XRF (p = 0.002).
For other types of applications, it is important to consider the other attributes of these two techniques to properly determine which is more suitable.
Table 4 is provided for comparison of qualitative instrument attributes. These attributes render the instruments applicable in different situations and therefore should be considered along with quantification performance results.
Conclusion
Two novel technological applications, XRF and LIBS, were evaluated to assess their potential to replace laborious traditional elemental analysis instruments for mineral quantification in pharmaceutical multivitamin tablets.
XRF provides lower prediction error and better linearity in the calibration curves. When comparing data analysis methods, neither multivariate nor univariate stands out as a better method for XRF. For simplicity reasons, the univariate approach is, thus, recommended, at the present state of the method’s development.
It could also be a solution to take advantage of both analysis methods to improve performance. Since one model is generated per element, it would be advantageous to choose specific analysis methods for each element which would be specific to model interactions or take into account element concentration ranges.
Furthermore, even though LIBS has not yet proven to perform as well as ICP and XRF in the studied case of mineral quantification in complex and heterogeneous multivitamin tablets, we are still well away from a final conclusion regarding its usefulness for pharmaceutical applications. On the contrary, it is a technology that offers much potential for 3D mapping of minerals in heterogeneous samples and could prove useful in reducing sample preparation resources needs (time and cost) for quantification in homogeneous and low-complexity samples.
References
- FDA. Guidance for industry PAT — A framework for innovative pharmaceutical development manufacturing, and quality assurance. . 2004:1.
- Radziemski LJ. From LASER to LIBS, the path of technology development. Spectrochimica Acta – Part B Atomic Spectroscopy. 2002;57:1109.
- ICET. LIBS2010-6th international conference on laser induced breakdown spectroscopy. http://www.icet.msstate.edu/libs2010/LIBS2010_Technical_Program_16July.pdf. Updated 2010. Accessed avril/19, 2012.
- Robertson MEA, Feather CE. Determination of gold, platinum and uranium in south african ores by high-energy XRF spectrometry. X-Ray Spectrom. 2004;33(3):164-173.
- Guo S-, Ge L-, Lai W-. XRF technique for rapid determination of fe ti content of fe ti- metal ore concentrates. Wutan Huatan Jisuan Jishu. 2007;29(5):436-438.
- St-Onge L, Archambault J-, Kwong E, Sabsabi M, Vadas EB. Rapid quantitative analysis of magnesium stearate in tablets using laser-induced breakdown spectroscopy. Journal of Pharmacy and Pharmaceutical Sciences. 2005;8(2):272-288.
- Madamba MC, Mullett WM, Debnath S, Kwong E. Characterization of tablet film coatings using a laser-induced breakdown spectroscopic technique. AAPS PharmSciTech. 2007;8(4).
- Dubey A, Keyvan G, Hsia R, et al. Analysis of pharmaceutical tablet coating uniformity by laser-induced breakdown spectroscopy (LIBS). Journal of Pharmaceutical Innovation. 2011;6(2):77-87.
- Myakalwar AK, Sreedhar S, Barman I, et al. Laser-induced breakdown spectroscopy-based investigation and classification of pharmaceutical tablets using multivariate chemometric analysis. Talanta. 2011;87(1):53-59.
- St-Onge L, Kwong E, Sabsabi M, Vadas EB. Quantitative analysis of pharmaceutical products by laser-induced breakdown spectroscopy. Spectrochimica Acta – Part B Atomic Spectroscopy. 2002;57(7):1131-1140.
- Arantes De Carvalho GG, Nunes LC, Florêncio De Souza P, Krug FJ, Alegre TC, Santos Jr. D. Evaluation of laser induced breakdown spectrometry for the determination of macro and micronutrients in pharmaceutical tablets. J Anal At Spectrom. 2010;25(6):803-809.
- Lewen N. The use of atomic spectroscopy in the pharmaceutical industry for the determination of trace elements in pharmaceuticals. J Pharm Biomed Anal. 2011;55(4):653-661.
- Marguí E, Fontàs C, Buendía A, Hidalgo M, Queralt I. Determination of metal residues in active pharmaceutical ingredients according to european current legislation by using X-ray fluorescence spectrometry. J Anal At Spectrom. 2009;24(9):1253-1257.
- Arzhantsev S, Li X, Kauffman JF. Rapid limit tests for metal impurities in pharmaceutical materials by X-ray fluorescence spectroscopy using wavelet transform filtering. Anal Chem. 2011;83(3):1061-1068.
- Mazel V, Reiche I, Busignies V, Walter P, Tchoreloff P. Confocal micro-X-ray fluorescence analysis as a new tool for the non-destructive study of the elemental distributions in pharmaceutical tablets. Talanta. 2011;85(1):556-561.
- Kim K-, Kim G, Kim J-, Park K, Kim K-. Kriging interpolation method for laser induced breakdown spectroscopy (LIBS) analysis of zn in various soils. J Anal At Spectrom. 2014;29(1):76-84.
Biography
Dr. Ryan Gosselin is an Associate Professor at the Department of Chemical & Biotechnological Engineering of the Université de Sherbrooke, Canada. He is a specialist in Process Engineering and on-line quality monitoring through the use of multivariate data analysis and chemometrics. As a member of the Pfizer Industrial Research Chair on Process Analytical Technologies (PAT) in Pharmaceutical Engineering, Ryan’s present work focuses mainly on issues relating to the production and handling of non-reactive particulate systems. Email: [email protected].
Nicolas Abatzoglou is a Full Professor and served as Head of the Department of Chemical & Biotechnological Engineering of the Université de Sherbrooke (2008-2012). He is an Adjunct Professor, University of Saskatchewan, Department of Chemical Engineering and presently Visiting Professor, Department of Chemical Engineering of the École Polytechnique de Montréal. Nicolas is a specialist in Process Engineering involving Thermochemical & Catalytic Conversion as well as Particulate Systems, and Director of the Research Centre GREEN-TPV (Groupe de Recherche en Énergie/Environnement-Technologies et Procédés Verts). Since May 2008, Nicolas is the holder of the Pfizer Chair in Process Analytical Technologies (PAT) in Pharmaceutical Engineering.
Philip Quinn is completing a M.Sc.A. in Chemical Engineering with the Université de Sherbrooke as part of the Pfizer Industrial Research Chair on Process Analytical Technologies (PAT) in Pharmaceutical Engineering. His research goals are to develop rapid analytical methods for API quantification in pharmaceutical tablets using multivariate predictive models. Philip has a Bachelor’s degree in Biotechnological Engineering and his Master’s degree is being completed at Pfizer Montreal’s facility (Canada) as a PAT project leader for technology transfer and development.
Joanny Salvas has a Bachelor’s degree in biotechnological engineering. She has completed a M.Sc.A. in Chemical Engineering with the Université de Sherbrooke, conducting her graduate work at Pfizer Montreal’s facility (Canada), as part of a Chair partnership. Her research is aimed at optimising the development protocol of multivariate predictive models used as part of PAT methods. Joanny currently is a PAT Sr Scientist at Pfizer Montreal, where she pursues several projects with different technologies such as Raman spectroscopy and Rapid Microbiological Methods. She is also leading projects for international sites.
Jean-Sébastien Simard is Senior Manager/Team Leader located in Montreal, Canada. He has a Bachelor and a Master’s degree in Chemical Engineering from Université de Sherbrooke. He is currently pursuing a MBA degree at Université Laval in Québec, Canada. He started in 2002 with Wyeth Canada where he worked as a Product and Process Development Scientist for the pharmaceutical processing unit. Jean-Sébastien then became responsible for the Process Analytical Technology Development Group of the Technical Services in Montréal, and he now leads a team that supports the implementation of PAT across all sites in Pfizer’s Consumer Health and Local Markets operating units. Jean-Sébastien is also the Industrial Responsible of the Université de Sherbrooke/Pfizer Industrial Research Chair on Process Analytical Technologies in Pharmaceutical Engineering.