AI in pharmaceutical development: hype or panacea?
Posted: 2 April 2025 | Dave Elder (David P Elder Consultancy) | No comments yet
Dave Elder reflects on the array of applications for AI to facilitate successful drug development, though proof remains elusive.
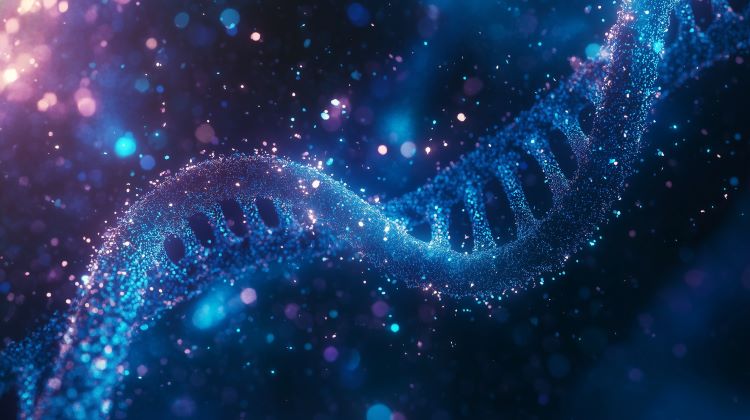
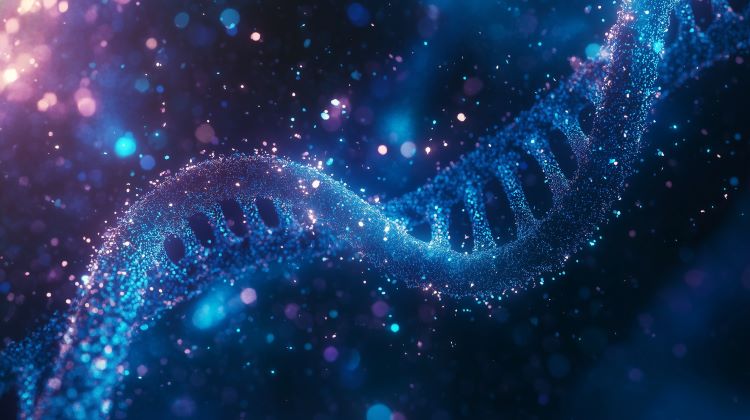
You could be forgiven for thinking that the future is bright, that the future is artificial intelligence (AI). AI-driven clinical development could benefit industry to the tune of up to $25 billion, owing to “smarter trials, better data, quicker results”.1
Most analysts think that AI will help cut down on timelines with AI-assisted clinical development estimated to take three to five years, versus the standard seven to nine years.2 Lilly CEO David Ricks sees “AI augmenting human productivity [and] automating regulatory processes”. He indicates an expectation that AI will “massively change the productivity of the workplace”.3
High attrition rates in early clinical trials have bedevilled the industry in recent years, and the average likelihood of approval (LOA) for a new Phase I drug is now just 6.7 percent.4 However, a recent article highlighted that “AI-discovered molecules have an 80-90% success rate, substantially higher than historic industry averages”.5,6
Nonetheless, a recent Nature editorial indicated that such claims “have come from the companies themselves. Until they can be independently verified, some caution is in order. The findings need to be published in the peer-reviewed literature and authenticated by researchers unaffiliated with the companies involved.”7,8
Some of the challenges arise from the data quality/data sharing/proprietary data that are available as training sets for AI systems.9 Indeed, data – particularly “big data”10 – represents some of the areas wherein AI offers the most opportunities and greatest challenges. Large datasets of well curated data, presented in the same format, are particularly amenable to profiling by AI systems.
The trending of newly emerging adverse events (AEs) of marketed products using AI is currently being assessed.11-13 Recent reports from US Food and Drug Administration (FDA) authors concluded that “AI can usefully be applied to some aspects of ICSR (individual Case Safety Reports) processing and evaluation, but the performance of current AI algorithms requires a ‘human-in-the-loop’ to ensure good quality.”12,13
The use of AI to identify starting materials and a commercially viable synthetic route for drug substances via retrosynthetic analysis is also becoming common place, eg, ICSYNTH.14 This approach can accelerate the investigation of multiple synthetic pathways for complex molecules while reducing the time, cost and effort expended in experimental design. AI is playing an increasing role in sustainable development15 and ‘green chemistry’ by “optimizing chemical processes to minimize environmental impact”.16 However, most of the synthetic data currently published in scientific journals is biased, as only “positive” data are typically published, ie, optimal yields, best reagents, etc.17
AI also has useful applications in GMP manufacturing of drug substance and drug products. AI-based optic systems, for example, can enhance ‘defect screening’ in manufacturing by scanning for packaging defects.18 AI can also facilitate existing risk management processes,19 such as corrective and preventative action (CAPA), root cause deviations and risk assessments.
Other uses include as an adjunct to continuous manufacturing where there is a need for real time release,20 and the complimentary requirement to have enhanced fault detection and continuous in-line/off-line/at-line process monitoring.21 AI can improve existing demand forecast and inventory optimisation approaches, as well as facilitate manufacturing equipment utilisation leading to improved production efficiencies and cost savings.20,21 The existing principles outlined in GMP Computerized System Validation (Annex 11)22 provide an established framework for risk assessments to both manage and mitigate any potential new risks for high-risk AI-based systems (defined as per the new EU AI act).23
In conclusion, the case for AI having a ‘game changing’ effect on pharmaceutical development remains unproven; among the most significant remaining issues are data quality, quantity and bias.24 As summarised by Blanco-Gonzalez et al, AI can “only provide predictions based on the data available… the results must then be validated and interpreted by human researchers”.24
About the author
Dave Elder, PhD
References
1. Generative AI in the pharmaceutical industry: Moving from hype to reality. [Internet] McKinsey & Company. 2024 [cited 2025Feb]. Available from: https://www.mckinsey.com/industries/life-sciences/our-insights/generative-ai-in-the-pharmaceutical-industry-moving-from-hype-to-reality
2. Masson G. Big Pharma, Biotech Relations ‘Won’t Necessarily Be Symbiotic’ In Future AI Landscape: S&P. [Internet] Fierce Biotech. 2024 [cited 2025Feb]. Available from: https://www.fiercebiotech.com/biotech/big-pharma-biotech-relations-wont-necessarily-be-symbiotic-future-ai-landscape-sp
3. Lee YJ. The CEO of Pharma Giant Eli Lilly Shares 3 Ways AI Could Transform His Industry. [Internet] Business Insider. 2023. [cited 2025Feb]. Available from: https://www.businessinsider.com/eli-lilly-ceo-interview-ai-artificial-intelligence-biotech-healthcare-2023-5
4. Chancellor D. Why Are Clinical Development Success Rates Falling? [Internet] Norstella. 2024. [cited 2025Feb]. Available from: https://www.norstella.com/why-clinical-development-success-rates-falling/
5. Jayatunga MKP, Ayers M, Bruens L, et al. How Successful Are AI-Discovered Drugs In Clinical Trials? A First Analysis And Emerging Lessons. Drug Discov. Today. 2024;29(6):104009.
6. Jayatunga MKP, Xie W, Ruder L, et al. AI in Small-Molecule Drug Discovery: A Coming Wave? Nat. Rev. Drug Discov. 2022. [cited 2025Feb]. Available from: https://www.nature.com/articles/d41573-022-00025-1
7. AI’s Potential To Accelerate Drug Discovery Needs A Reality Check. [Internet] Nature. 2023;622(7982):217–7. Available from: https://www.nature.com/articles/d41586-023-03172-6
8. Mock M, Edavettal S, Langmead C, Russell A. AI Can Help To Speed Up Drug Discovery – But Only If We Give It The Right Data. Nature. 2023; 621(7979): 467–470.
9. Ridzuan F, Zainon WMNW. A Review on Data Quality Dimensions for Big Data. Procedia Computer Science. [Internet] Procedia Computer Science. 2024 ;234:341–8. Available from: https://www.sciencedirect.com/science/article/pii/S187705092400365X
10. Yang S, Kar S. Application Of Artificial Intelligence And Machine Learning In Early Detection Of Adverse Drug Reactions (Adrs) And Drug-Induced Toxicity. Artif. Intell. Chem. 2023; 1(2): 100011.
11. Syrowatka A, Song W, Foer D, et al. Key Use Cases For Artificial Intelligence To Reduce The Frequency Of Adverse Drug Events: A Scoping Review. The Lancet. 2023; 4(2): E137-E148.
12. Ball R, Dal Pan G. “Artificial Intelligence” for Pharmacovigilance: Ready for Prime
Time? Drug Safety. 2022; 45(5): 429-438.
13. FDA Discussion Paper And Request For Feedback. Using Artificial Intelligence & Machine Learning In The Development Of Drug and Biological Products. [Internet] FDA. 2025. [cited 2025Feb] Available from: https://www.fda.gov/media/167973/download
14. Synthesis V Retrosynthesis: How To Use Both To Optimise Your Experiments. [Internet] Deepmatter. 2023. [cited 2025Feb]. Available from: https://www.deepmatter.io/insights/blog/synthesis-v-retrosynthesis-how-use-both-optimise-your-experiments/
15. Vinuesa R, Azizpour H, Leite I, et al. The Role Of Artificial Intelligence In Achieving The Sustainable Development Goals. Nature Communications. 2020; 11(1): 233.
16. Singh G, Rajaskha ESK, Mounika K, et al. Artificial Intelligence in Green Organic Chemistry: Pathway to Sustainable and Eco-Friendly Chemistry. Asian Journal of Chemistry. 2024. [cited 2025Feb]; 36(12); 2731-43. Available from: https://asianpubs.org/index.php/ajchem/article/view/36_12_3/29886.
17. Brazil R. Illuminating “The Ugly Side Of Science”: Fresh Incentives For Reporting Negative Results. [Internet] Nature. 2024. [cited 2025Feb ]. Available from: https://www.nature.com/articles/d41586-024-01389-7
18. Pace R. Elevating Pharma Inspection With Deep Learning. [Internet] Pharma Manufacturing. 2021. [cited 2025Feb]. Available from: https://www.pharmamanufacturing.com/industry-perspectives-sponsored/article/11293580/elevating-pharma-inspection-with-deep-learning
19. Wittwehr C, Blomstedt P, Gosling JP, et al. Artificial Intelligence for Chemical Risk Assessment. Computational Toxicology. 2020; 13: 100114.
20. Artificial Intelligence In Drug Manufacturing. Discussion paper. 2023 [cited 2025Feb]. [Internet] FDA. Available from: https://www.fda.gov/media/165743/download
21. Application of AI in a GMP / Manufacturing Environment – an Industry
Approach [Internet]. EfPIA. 2024. [cited 2025Feb]. Available from: https://www.efpia.eu/media/vqmfjjmv/position-paper-application-of-ai-in-a-gmp-manufacturing-environment-sept2024.pdf
22. EudraLex The Rules Governing Medicinal Products in the European Union Volume 4 Good Manufacturing Practice Medicinal Products for Human and Veterinary Use Annex 11: Computerised Systems. [Internet] European Commission. [cited 2025Feb]. Available from: https://health.ec.europa.eu/system/files/2016-11/annex11_01-2011_en_0.pdf
23. EU AI Act: First Regulation On Artificial Intelligence [Internet]. European Parliament. [Internet] European Parliament. 2023. [cited 2025Feb]. Available from: https://www.europarl.europa.eu/topics/en/article/20230601STO93804/eu-ai-act-first-regulation-on-artificial-intelligence
24. Blanco-González A, Cabezón A, Seco-González A, et al. The Role of AI in Drug Discovery: Challenges, Opportunities, and Strategies. Pharmaceuticals (Basel). 2023;16(6): 891.
Issue
Related topics
Artificial Intelligence, Big Data, Big Pharma, Biopharmaceuticals, Clinical Development, Clinical Trials, Drug Development, Drug Manufacturing, Good Manufacturing Practice (GMP), Industry Insight, Manufacturing, Regulation & Legislation, Research & Development (R&D), Technology, Therapeutics