RNA: Converting imaging-based cell biology to high-throughput biology
Posted: 19 February 2014 | | No comments yet
The last 10 years in biomedical research marks the period of deepening our understanding of the human genome. In the context of cancer research, The Cancer Genome Atlas (TCGA) and related international genomics efforts have now revealed the full complexity of genomic aberrations in human cancers that are postulated to contribute to the aspects of cancer pathophysiology. It is plausible that an ensemble of the numerous aberrations in each individual tumour collaborate at various strengths to deregulate master signalling pathways of cells, thereby enabling the established cancer ‘hallmarks’1.
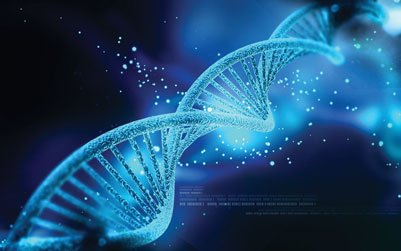
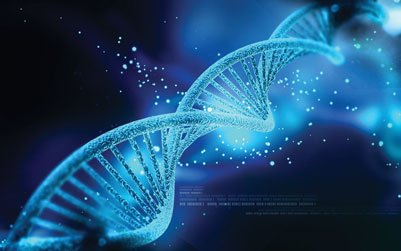
However, the functional roles of the majority of these newly identified gene mutations in cancer are largely unknown. Association of the genomic aberrations with specific cell biological processes and identification of potential cancer driver genes will require extensive systematic cell biological studies. Whereas RNAi screening technologies provide the opportunity to rapidly address gene functions in virtually any cell biological process, the majority of current RNAi screen projects still rely on simple bulk assays focusing on cell viability, thus limiting the organisation of gene functions into networks of specific cellular processes. Here, microscopy-based RNAi screening is compared with cell viability assays to highlight the advantages that imaging-based cell biology can provide for high-throughput biology.
Current state of RNA interference screening in high-throughput biology
During the on-going ‘post-genomic era’ of cell biological research, the scope and diversity of functional cell based analyses has moved onto a level unimaginable only a decade ago. The information produced by the genetic profiling efforts of human diseases across thousands of individuals has led to the development of a detailed ‘landscape’ view of human genetics. This has been complemented with parallel development of genome covering reagent collections of e.g. cDNA, siRNA and miRNA libraries which have transformed the nature of biological inquiry of cellular processes by allowing organism-scale functional explorations.
The substantial increase of samples included in cell biological experiments has also given rise to a whole new class of analysis instrumentation to compensate for the needs of high-throughput biology. Adaptation of RNA interference to laboratory practice for loss-of-function genomic studies is undoubtedly among the most fundamental novel techniques emerging in life science research during the last decade. RNAi has profoundly altered the concept of experimental approaches used to study gene functions in vitro and led to the rapid and widespread use of this technique in all areas of cell biological research. Caenorhabditis elegans and Drosophila melanogaster were the first model organisms for systematic loss-of-functions RNAi screens2-4. The success of these first described RNAi screens facilitated development of commercially available genome-scale RNAi reagent libraries which thereafter have become broadly accessible to academic laboratories around the world. Soon after the description of RNAi in the initial model organisms, it was discovered that RNAi also suppresses gene expression in mammalian cells5. Thereafter, the high-throughput screening (HTS) of RNAi libraries has become an essential tool of biological studies to elucidate gene functions in human cells.
In a typical mammalian RNAi screening experiment today, cultured cells are dispensed onto 96- or 384-multiwell plates and lipid transfection agents are used to mediate RNAi reagent uptake for induction of target gene silencing. Following transfection, cells are analysed for a phenotype of interest, such as cell viability. Cancer research has been in the forefront of RNAi screening in human cells from the beginning. Screens using synthetic siRNAs targeting defined gene families or the complete genome have been used in cancer research to elucidate multiple biological processes from a systemic scope. Among the first large-scale human RNAi screens, synthetic siRNAs were used in HeLa cervical cancer cells to identify modulators of TRAIL-induced apoptosis6 and regulators of chemoresistance7, whereas U2OS sarcoma cells were applied in a genome-wide cell cycle regulation screen8. From these applications to today, a common theme for RNAi screens in cancer research has been the identification of growth inhibitory RNAi targets. Multiple studies panning across panels of cancer model cell lines have used cell viability assays to nominate potential cancer target genes9-11. Outside the cancer context, analysis of kinases involved in endocytosis through clathrin and caveolae-mediated pathways12 was among the first described screens using synthetic siRNAs.
After the early applications, the complexity of siRNA screen designs evolved significantly, including through application of more complex assay techniques. In more recent screening studies, systematic RNAi has been applied in multiple different fields of cell biology, including detailed characterisation of regulation of cell cycle, cell adhesion and motility, metabolism, autophagy, neurite growth, drug resistance, circadian clock, DNA damage responses, anchorage-independent growth of cancer cells, viral infection mechanisms and many more. As many cell biological processes and features, including basic analyses of cell morphology, require use of specific assays non-measurable with bulk detection techniques, a need for more efficient ways to interrogate cell biology in high-throughput mode is evident. Indeed, applying imaging-based cell biological readouts for RNAi screening has been shown to provide highly effective ways to interrogate gene functions. Large-scale screens using imaging-based assays in human cells have been used for identification of genes involved in proteasome functioning13, regulation of cell cycle and mitotic progression14,15, cell motility16, and e.g. activation of integrin cell adhesion receptors17 to name a few. Among the most active fields in imaging-based RNAi screening has been the analysis of viral infection mechanisms with multiple systemic-scale screens described thus far (for a summary analysis, see Snijder et al.18). While these reports establish a solid view that RNAi screening using imaging-based techniques can be employed to perform loss-of-function screens with increased accuracy, it is also clear that the full potential of imaging-based assays for large-scale RNAi applications has yet to be revealed. For example, most described imaging-based RNAi studies have relied on the analysis of a single parameter at a time. Though well suited for a specific biological question, this ignores systems complexity on cell biology. Furthermore, many assays cannot be converted to HTS format due to methodological limitations or cost considerations. For future applications, more flexible screening platforms, such as the miniaturised cell spot microarrays19 can be used to expand large-scale RNAi screens to multiplexed high-end assay techniques20.
Comparison of imaging-based cytometry and enzymatic cell viability readouts
The continuing popularity of using enzymatic assay techniques to measure cell viability in HTS experiments is explained by the simplicity of application (Figure 1A). Bulk detection assays can be automated with relative ease, they provide a cost efficient approach to large-scale experiments and standardisation of these assays is well described21. Microscopy-based assays on the other hand allow targeted interrogation of virtually any cell biological processes, multiplexed readouts, and fixed and time resolved analyses, but depend on greater expertise on assay standardisation, analysis and interpretation (Figure 1A).
As assays measuring cell viability on RNAi screens is the most commonly used approach, an unbiased comparison of identical RNAi experiments using image cytometry and a popular HTS cell viability reagent CellTiter-Glo® (Promega) is a good example to compare the detection differences of these two techniques. For this purpose, human ovarian cancer KFr13 cells were transfected on 384-well plates with two repeats of a kinome-wide siRNA library (Qiagen) covering 639 putative human kinases with two individual siRNAs for each and an ensemble of positive and negative control siRNA constructs21. A replicate screen was used for analysis of cell viability using CTG assay and another was processed for microscopic quantification of cells based on organelle stains for DNA and filamentous actin19. Both replicate screens were normalised using the cellHTS2 package22 for statistical analysis and the siRNA effects on cell viability were scored using B-scores (Figure 1B). At first glance, the cell viability readouts based on quantification of cell counts and the CTG assay appears to have overall similar results (R=0.58). On the whole library level, the fraction of the library that altered growth of the model cells according to both detection methods is quite similar, although the imaging-based readout displays distinct outlier siRNA hits not detected by the CTG assay (Figure 1B). The most prominent cell viability reducing siRNA hits and controls targeting e.g. PLK1 (polo-like kinase 1) are all represented among the common growth inhibitory hits from both experiments (Figure 1B). The most recurrently differentially detected siRNAs in the experiment intriguingly also included a fraction of the positive control siRNAs targeting CDK1 (cyclin-dependent kinase 1) (Figure 1B). The difference in detecting the growth arrest induced by inhibition of CDK119-20 indicates a potential clue to the biological difference of the two detection methods and the assay sensitivity they provide.
The biological differences between image cytometry and enzymatic cell viability readouts
As indicated by the head-to-head comparison of the cell viability measurements, detection of potent viability reducing events displays high concordance with both imaging and enzymatic assays. A fraction of the strongest cell growth reducing events indicated by imaging are not detected with the enzymatic readout. This is not surprising, given that this assay is based on measuring metabolite ATP levels as a relative indicator of viable cells and imaging accounts for absolute cell counts. Moreover, closer inspection of the cell cycle distribution (Figure 2A-B) (fraction of cells in G1/S, G2, M-phase and percentage of polyploid cells) available from the image-based cytometry analysis15,19,20 reveals substantial differences between the microscopic analysis and the enzymatic cell viability readout. In general, the majority of siRNA effects resulting in a cell cycle arrest not accompanied with induction of apoptosis are not identified by the CTG cell viability analysis (Figure 2B). Yet a comparison of the highlighted G1 and G2 cell cycle phase arresting RNAi targets (Figure 2A) reveals these to also be identified in previous RNAi cell cycle profiling studies8,15,24. Several factors plausibly contribute to these gene perturbation phenotypes not being detected as growth inhibitory by measurement of ATP levels.
First, a visual inspection of cells transfected with siRNAs (Figure 2C) resulting in e.g. a G2 arrest (MASTL25) or accumulation of polyploid cells (AURKB20), indicates that the cells affected by these knockdowns tend to be significantly enlarged24 or grow more sparsely (CAMK2B) in comparison to the control cells. This could convert to the enlarged / dispersed cells having higher amounts of ATP as well due to the increased cellular volume in comparison to control cells and therefore not being detected by the enzymatic assays. Second, apart from the abnormal cellular phenotypes potentially masking growth inhibition detection by enzymatic assays, considering enrichment of specific molecular pathways can be used to address the basis for the biological difference between the readouts. In the current context, functional annotation26 of the RNAi hits identified by the image cytometry analysis links the majority of these to biologic pathways associated with regulation of cell cycle and response to stress stimuli (Figure 2D). This indicates that the enzymatic cell viability assay could be prone to miss cell cycle arresting perturbations where analysed cells remain viable, yet clearly affected as revealed by the imaging-based analysis.
Biological correlation of detection method specific results
Besides the importance of selecting the appropriate assay technique for a specific cell biological question, selection of representative model cells can define the success of a screening experiment. In cancer research, the established cancer cell lines employed as models are considered to closely mirror many aspects of the genomic profiles of clinical samples in vivo. As growth of cancer cells is driven in part by the overexpressed oncogenes1, loss-of-function and genomics profiling data can be integrated to assess the biological relevance of e.g. RNAi-mediated growth inhibitory phenotypes9-11,19. Thus, a useful approach for the first line of validation of biological relevance of data from high-throughput cell viability studies is to compare gene expression signatures of the RNAi target genes in the model cells (Figure 3A).
Scored RNAi data for the measured phenotypes can be overlaid with mRNA expression levels27 of the targeted genes to nominate candidates that display a correlation between the RNAi phenotype and expression level. To evaluate relevance of the siRNA results from the imaging-based cytometry assay discussed here, a list of the top ranking targets were filtered for kinase coding genes overexpressed in the KFr13 model cell line (cutoff criterion of overexpression > 2, log2 median centered expression of all kinases). Twenty six per cent of these candidate kinase genes were found to be overexpressed. To evaluate clinical relevance of the targets, overlap between the cell line and in vivo genome profiles can be used as a second line of in silico validation. Here, a comparison of the image cytometry hit genes against data from primary ovarian tumours28 indicates several of these genes to be subjects of recurrent copy number gains in vivo. For example, PKN1 (protein kinase N1), which was identified as a G1 cell cycle arresting hit only by the image cytometry, is found amplified in ~15 per cent of the ovarian cancer samples in TCGA29, most frequently of all included tumour types (Figure 3B). In addition, transcriptomics evaluation of PKN1 expression across epithelial cancer samples included in the GeneSapiens (www.genesapiens.org) database30 indicates highest expression levels of this kinase gene specifically in ovarian cancer samples (Figure 3C). Taken together, these results demonstrate that the strategy of using imaging based assays for HTS can be used to identify detailed cell biological connections. Moreover, the in silico validation strategy for evaluation of the biological relevance of candidate genes can be used to link cellular RNAi phenotypes to specific molecular networks and wise versa. Here, this approach revealed a previously un-described link between PKN1 growth inhibitory RNAi phenotype and the overexpression profile in clinical ovarian cancers.
Concluding remarks
Despite the major advancements reached in mammalian cell biology in elucidating gene functions underlying cellular processes using highly parallel RNAi screens, the advantages of implementing more accurate assay strategies in these studies are becoming ever more evident. With application of imaging-based cell biological assays, the general accuracy of loss-of-function and small molecule screens can be improved significantly. This will benefit both systems biology approaches and basic biology research. Multiplexing detection of cellular responses with morphological phenotype analyses such as image-based cell cycle analysis will allow also generation of systems level views on gene functions beyond the initial and most simple responses such as impacts on the viability of cells. Subsequently, the cell biological associations revealed with these analyses can be better correlated with the biological context of the model system for evaluation of biological relevance. In summary, the strategy for functional characterisation of gene functions using imaging based cell biology, and implementing these to high-throughput experiments will be the logical next avenue of high-throughput biology.
References
- Hanahan, D. and R.A. Weinberg, Hallmarks of cancer: the next generation. Cell, 2011. 144(5): p. 646-674
- Fraser, A.G., et al., Functional genomic analysis of C. elegans chromosome I by systematic RNA interference. Nature, 2000. 408: p.325–330
- Piano, F., et al., Gene clustering based on RNAi phenotypes of ovary enriched genes in C. elegans. Curr. Biol., 2002, 12: p.1959-1964
- Boutros, M., et al., Genome-wide RNAi analysis of growth and viability in Drosophila cells. Science, 2004, 303: p.832–835
- Elbashir, S.M., et al., Duplexes of 21-nucleotide RNAs mediate RNA interference in cultured mammalian cells. Nature, 2001, 411: p.494–498.
- Aza-Blanc, P., et al., Identification of modulators of TRAIL-induced apoptosis via RNAi based phenotypic screening. Mol Cell., 2003, 12(3): p.627-637
- MacKeigan, J.P., Murphy, L.O. and Blenis, J., Sensitized RNAi screen of human kinases and phosphatases identifies new regulators of apoptosis and chemoresistance. Nat Cell Biol., 2005, 7(6): p.591-600
- Mukherji, M., et al., Genome-wide functional analysis of human cell-cycle regulators. Proc Natl Acad Sci U S A., 2006, 103(40): p.14819-14824
- Grade, M., et al., A genomic strategy for the functional validation of colorectal cancer genes identifies potential therapeutic targets. Int J Cancer., 2011, 128(5): p.1069–1079
- Herrera-Abreu, M.T., Parallel RNA Interference Screens Identify EGFR Activation as an Escape Mechanism in FGFR3-Mutant Cancer. Cancer Discov., 2013, 3(9): p.1058-1071
- Tan, T.Z., et al., Functional genomics identifies five distinct molecular subtypes with clinical relevance and pathways for growth control in epithelial ovarian cancer. EMBO Mol Med., 2013, 5(7): p.983-998
- Pelkmans, L., et al., Genomewide analysis of human kinases in clathrin- and caveolae/raft-mediated endocytosis. Nature, 2005, 436(7047): p.78-86
- Simpson, J.C., et al., An RNAi screening platform to identify secretion machinery in mammalian cells. J Biotechnol., 2007, 29(2): p.352-365
- Moffat, J., et al., A lentiviral RNAi library for human and mouse genes applied to an arrayed viral high-content screen. Cell, 2006, 124(6): 1283-1298
- Neumann, B., et al., Phenotypic profiling of the human genome by timelapse microscopy reveals cell division genes. Nature, 2010, 464(7289): p.721-727
- Simpson, K.J., et al., Identification of genes that regulate epithelial cell migration using an siRNA screening approach. Nat Cell Biol., 2008, 10(9): p.1027-1038
- Pellinen, T., Rantala J.K., et al., A functional genetic screen reveals new regulators of β1-integrin activity. J Cell Sci., 2012, 125(Pt 3): p.649-661
- Snijder, B., et al., Single-cell analysis of population context advances RNAi screening at multiple levels. Mol Syst Biol., 2012, 8: 579
- Rantala, J.K., et al., A cell spot microarray method for production of high density siRNA transfection microarrays. BMC Genomics, 2011, 28(12): 162
- Rantala, J.K., Kwon, S., Korkola, J., and Gray, J.W., Expanding the diversity of imaging-based RNAi screen applications using cell spot microarrays. Microarrays, 2: p.97-114
- Echeverri, C.J., et al., Minimizing the risk of reporting false positives in large-scale RNAi screens. Nat Methods, 2006, 3(10): p.777-779
- Rantala, J.K., et al., Sharpin is an endogenous inhibitor of b1 integrin activation. Nat. Cell Biol., 2011, 13(11): p.1315-1324
- Pelz, O., Gilsdorf, M., and Boutros, M., Web cellHTS2: a web-application for the analysis of high-throughput screening data. BMC Bioinformatics, 2010, 11: 185
- Björklund, M., et al., Identification of pathways regulating cell size and cell-cycle progression by RNAi. Nature, 2006, 439(7079): p.1009-1013
- Voets, E. and Wolthuis, R.M., MASTL is the human orthologue of Greatwall kinase that facilitates mitotic entry, anaphase and cytokinesis. Cell Cycle, 2010, 9(17): p.3591-3601
- National Institute of Allergy and Infectious Diseases. Database for Annotation, Visualization and Integrated Discovery (DAVID). http://david.abcc.ncifcrf.gov
- Krzywinski, M., et al., Circos: an Information Aesthetic for Comparative Genomics. Genome Res., 2009, 19: p.1639-1645
- Cancer Genome Atlas Research Network. Integrated genomic analyses of ovarian carcinoma. Nature, 2011, 474: p.609–615
- Gao, et al., Integrative analysis of complex cancer genomics and clinical profiles using the cBioPortal. Signal., 2013, 6: pl1
- Kilpinen, S., et al., Systematic bioinformatic analysis of expression levels of 17,330 human genes across 9,783 samples from 175 types of healthy and pathological tissues. Genome Biol., 2008, 9(9): R139
Biography
Juha Rantala obtained his PhD in Biochemistry and Medical Biotechnology from the University of Turku and the Technical Research Centre of Finland in 2011 with work leading to development of the Cell Spot MicroArray method for imaging based RNAi screening. Dr. Rantala transitioned to the Department of Biomedical Engineering and Knight Cancer Institute at Oregon Health and Science University in 2011 to participate on building the new OHSU Center for Spatial Systems Biomedicine. Since 2012, Dr. Rantala has been an Assistant Research Professor in the laboratory of Dr. Joe W. Gray, where he leads research projects aimed on elucidating the functional roles of recurrent genetic aberrations in breast cancers.