Adopting AI for R&D in 2025 – what needs to be addressed?
Posted: 19 December 2024 | Dr Vladimir Makarov (The Pistoia Alliance), European Pharmaceutical Review | 1 comment
Dr Vladimir Makarov, AI Community lead, The Pistoia Alliance, explains that despite the willingness of the life sciences R&D industry to adopt artificial intelligence (AI), data integrity is a key area that must be addressed to ensure successful integration of this fast-evolving technology.
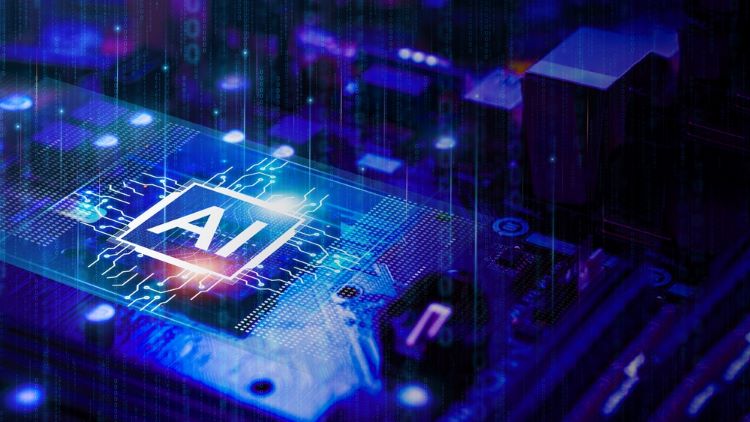
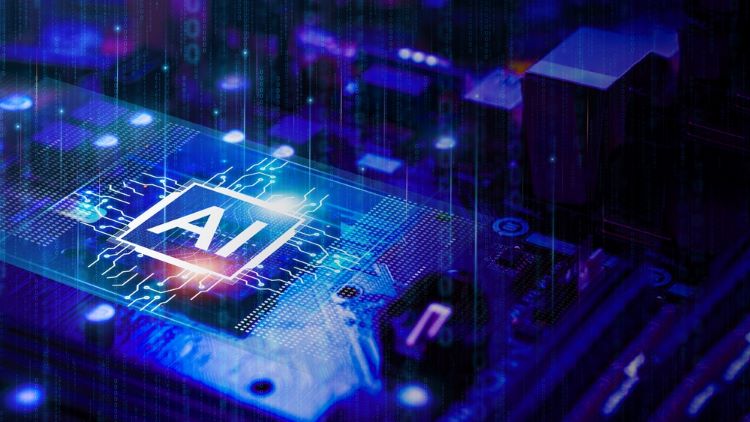
With the life sciences and healthcare sectors increasing integration of artificial intelligence (AI) into their infrastructure, according to research by GlobalData, one-third of healthcare professionals considered data privacy is the primary obstacle, citing challenges such as “robust safeguards, workflow integration, and stakeholder acceptance [being] essential to ensure AI’s ethical implementation and long-term success”.1
These concerns are held alongside the promise of this technology and its potential for “improving efficiency, enhancing patient outcomes, and addressing critical challenges like staffing shortages. However, the road to successful implementation is fraught with concerns around data privacy, workflow integration, and acceptance by both patients and physicians,” stated Sachin Gharat, Associate Project Manager, Pharma at GlobalData.1
What does the AI hype cycle mean for the life sciences R&D?
[For] AI use in life sciences R&D… 2025 will see the industry enter the “plateau of enlightenment”
AI use in life sciences R&D has now passed through most major stages of the Gartner Hype Cycle2 and 2025 will see the industry enter the ‘plateau of enlightenment’. This means that AI technology is widely used, and that at the same time, best practices and applications are still being discovered and recorded. Strengths and weaknesses of AI are generally well understood by the life sciences industry, meaning we are now seeing more rational investments in AI and specific use cases, rather than impulsive spending. Yet, AI remains the top area for investment over the next two years, according to 62 percent of respondents in Pistoia’s 2024 Lab of the Future report.3
How are attitudes changing towards AI from corporate researchers in industries including pharmaceuticals and life sciences?
Low quality and poorly curated datasets is the number one barrier to AI implementation (cited by 52 percent)”
There is a widespread willingness among researchers to use AI, with 68 percent3 of life science professionals using it in 2024, compared to 54 percent in 2023. However, 28 percent of researchers still hold the perception that AI is not trustworthy, reliable, or responsible. Partly this perception is driven by challenges around the quality of content used to train AI. Low quality and poorly curated datasets is the number one barrier to AI implementation (cited by 52 percent).
Researchers are very aware that poor quality inputs can lead to inaccurate and biased AI outputs, which can have huge consequences in a field such as drug development, where patient health is at stake. In 2025, the industry must collaborate on these data challenges to enable the remaining third of researchers who are not using AI to do so safely and confidently.
What about the ongoing need for trusted AI systems and data in a world where everyone has access to AI tools?
For AI to be successful in drug development and other areas of pharma, there are two critical requirements: trustworthy AI systems and Findable, Accessible, Interoperable, Reusable (FAIR) data.
Trustworthy AI4 centres around transparency of what data is being input to models, explainability of results, and confidentiality of sensitive data. These ideas are central to emerging AI regulations, such as such as the 2023 US AI Executive Order,5 the 2024 Memorandum,6 and the EU AI Act, which will require high-risk applications of AI to file conformity assessments to prove their transparency and trustworthiness.
FAIR data is the backbone which underpins any good AI model”
Meanwhile, FAIR data is the backbone which underpins any good AI model. Adhering to the FAIR principles ensures data can more freely move through the research environment, so greater value can be unlocked over longer periods of time, including enabling more effective secondary reuse.
However, from speaking to our members, there is still some way to go before the industry ticks both the FAIR and transparency boxes. Yet 38 percent still cite data that is not FAIR as an AI barrier.
In 2025, organisations must focus on addressing data integrity challenges. Learning from each other’s mistakes and successes will be key to advancing AI adoption. The Pistoia Alliance has long been a pioneer of FAIR data, and we invite organisations to access some of the resources we have developed with the industry. For example, our 2024 paper on good machine learning practices,7 or our FAIR Toolkit.8
About the interviewee
References
1. One Third Of Hcps Prioritize Data Privacy As The Top Challenge For AI In Clinical Practice, Says GlobalData. [Internet] GlobalData. 2024. Available from: https://www.globaldata.com/media/pharma/artificial-intelligence-in-clinical-practice-physician-perspective-2024/
2. Gartner Hype Cycle – Interpreting Technology Hype. [Internet] Gartner. Available from: https://www.gartner.com/en/research/methodologies/gartner-hype-cycle
3. Lab of the Future Survey 2024. [Internet] Pistoia Alliance. 2024. Available from: https://www.pistoiaalliance.org/blog/lab-of-the-future-2024-global-survey/
4. AI/ML Webinar – Trustworthy AI. [Internet] Pistoia Alliance. 2022. Available from: https://www.pistoiaalliance.org/pistoia-webinars/trustworthy-ai/
5. Safe, Secure, and Trustworthy Development and Use of Artificial Intelligence. [Internet] US Federal Office. 2023. Available from: https://www.federalregister.gov/documents/2023/11/01/2023-24283/safe-secure-and-trustworthy-development-and-use-of-artificial-intelligence
6. Memorandum on Advancing the United States’ Leadership in Artificial Intelligence; Harnessing Artificial Intelligence to Fulfill National Security Objectives; and Fostering the Safety, Security, and Trustworthiness of Artificial Intelligence. [Internet] wh.gov. 2024. Available from:
https://www.whitehouse.gov/briefing-room/presidential-actions/2024/10/24/memorandum-on-advancing-the-united-states-leadership-in-artificial-intelligence-harnessing-artificial-intelligence-to-fulfill-national-security-objectives-and-fostering-the-safety-security/
7. Makarov V, Chabbert C, Koletou E et al. Good Machine Learning Practices: Learnings From The Modern Pharmaceutical Discovery Enterprise. Computers in Biology and Medicine. 2024; 177: 108632.
8. FAIR Implementation. [Internet] Pistoia Alliance. 2024. Available from: https://www.pistoiaalliance.org/projects/current-projects/fair-implementation/
Related topics
Artificial Intelligence, Big Data, Data integrity, Drug Development, Industry Insight, Regulation & Legislation, Research & Development (R&D), Robotics, Technology, Therapeutics
This article highlights critical considerations for integrating AI in life sciences R&D and healthcare, shedding light on challenges like data integrity, privacy, and stakeholder acceptance. Dr. Vladimir Makarov’s emphasis on data integrity aligns with the industry’s increasing reliance on high-quality data for effective AI deployment. It’s essential to address these challenges proactively to realize AI’s potential for improving efficiencies and patient outcomes.