Protein crystallography in drug design: current bottlenecks
Posted: 21 September 2007 | | No comments yet
Protein crystallography is an integral component of the structure-guided drug discovery process. Rapid access to structural information about drug targets as well as bound ligands has been pivotal in accelerating lead identification and optimisation processes…
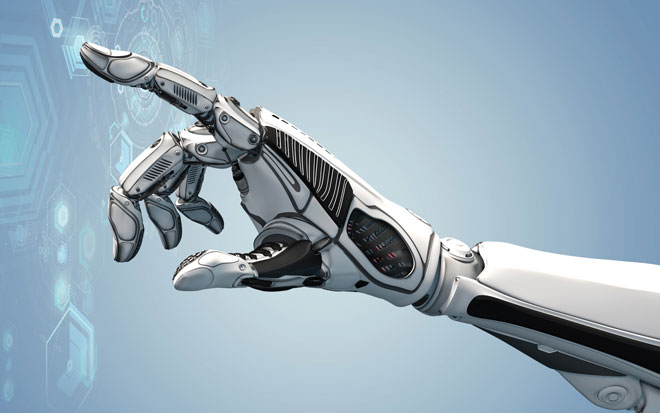
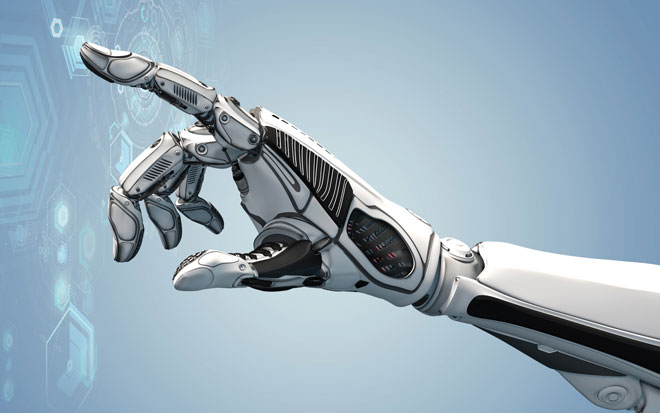
While automation and robotics have been employed at every stage along the gene-to-structure path, significant challenges remain in increasing successful outcomes and in reduction of timelines. Advances in high through-put technologies to automate protein expression and crystallisation, the two weakest links in the gene-to-structure process chain, are beginning to address these issues. This article will highlight the importance of rapid structure determination of protein-ligand complexes in lead optimisation, and describe recent developments towards overcoming these bottlenecks.
Role of structural biology in drug discovery
Structure-guided optimisation of lead compounds has rapidly gained ground and become an important player in the early drug discovery process. The goal of structural biology within the pharmaceutical industry is to aid medicinal and computational chemistry. The primary product of a structural biology team is information: three-dimensional structures of target proteins bound to lead compounds. The availability of structural information about a target early in the lead identification stage can significantly accelerate the lead optimisation process and concurrently help address issues pertaining to selectivity, pharmacokinetics, and patentability. With increasing competition and rapidly shrinking patent space, structural biology can also play a critical role in discovering and designing novel leads via fragment or focused library screening, and, in conjunction with computational chemistry, facilitate target-based virtual screening.
Maximising impact on lead optimisation
To have the greatest impact, today’s structural biology team needs to be involved early on in the discovery process, working collaboratively with the chemists. It is much more important for structural biology to help define where chemistry should go rather than to explain where they have been; that is, structural biology is best used as part of the lead optimisation process rather than only explaining why and how a pre-clinical candidate binds to its target.
To reach that goal, it is important for structural biology to be aligned with high-through-put screening (HTS). Due to the time consuming process described below, it is important that structural biology begin work at least as early as the initiation of an HTS campaign. Optimally, a suitable crystal will be ready and waiting for hits from HTS; in that case, structural biology can provide information throughout the hit-to-lead and lead optimisation process. Once screening hits are pursued, the workflow of medicinal chemistry, computational chemistry, and structural biology should be tightly integrated so that the composite team can quickly learn from each other’s results and make informed decisions. Determining structures of scaffolds that the chemists are no longer interested in, is usually a wasted effort.
Principal steps in protein crystallography
X-ray crystallography continues to be the workhorse of structural biology in providing high resolution protein structures. The principal steps in protein crystallography are shown in Figure 1. For a typical project, the structural biology team will:
- Design a small library of expression plasmids based on literature, bioinformatics, and corporate knowledge. Key variables include the N- and C-termini, fusion partners, protease cleavage sites, and functional or surface mutations
- Clone the expression plasmids
- Screen the construct library in various expression hosts and scale up the most promising variants
- Develop purification methods and find suitable buffer conditions to maximize protein solubility
- Analyse protein for parameters such as mass, post-translational modifications, oligomeric and oxidation state, specific activity, and confirmation of binding affinity for ligands or inhibitors
- Screen purified protein for crystallisation and optimise crystals to yield high resolution diffraction
- Collect and process diffraction data and refine the structure for a few or a few dozen protein: ligand complexes
- Share the structures throughout the organisation
This process is not unidirectional and the results from each step affect the entire workflow. To optimise efficiency and enable high resolution structures, a circular workflow develops and additional constructs are created.
Through efforts in both the structural genomics and pharmaceutical communities, the past five years have seen significant advances in high-throughput methods to accelerate the structure determination process1-3. Tremendous efforts in structural genomics (SG) have yielded many benefits for the entire crystallographic community4-8. Interestingly, many of the goals of SG consortia and pharmaceutical scientists are orthogonal. Whereas SG can be successful in solving only a moderate portion of their targets, industrial pressures dictate a nearly 100% success rate. Similarly, SG requires only a few quality crystals from which they can solve the structure, while industry needs many robust crystals for each target, particularly in the lead optimisation stage for solving dozens of structures with different ligands. Finally, the optimal protein crystal for drug discovery purposes diffracts to high resolution, withstands a few percent DMSO for soaking experiments, and is packed in such a way as to allow ligands to exchange with solvent; the same criteria are not present for SG.
Despite those differences, the process and workflow for each team of crystallographers is quite similar and SG has made significant contributions to the pharmaceutical industry processes. Automation is now routinely used at many points in the process, from cloning to crystal imaging, to data collection. SG and other consortia have developed automated software for the routine tasks of data processing and structure refinement. Finally, the protein structures solved by SG are now readily available for use by the scientific community at large, including protein crystallographers and computational chemists.
Bottlenecks in the process
In spite of these advances in automation, there are still two significant impediments to structure-guided drug discovery. The most important and yet difficult stage in the process is generating an optimal crystal which will supply high resolution structures of protein: ligand complexes. In order to find that crystal, great effort is spent screening and optimising crystals, a labor and time intensive task. In addition to the usual crystallisation variables (precipitant, pH, temperature), it is also important to treat the protein itself as a variable; the implicit assumption is that different constructs have different solubility and crystallisation propensities. Not all variants will be expressed, be soluble, or crystallise; therefore, testing a reasonably large number of constructs of a target protein should increase the probability of success. Automated crystallisation screening reduces the burden created by this increase in proteins screened. Unfortunately, the production of large amounts of pure, soluble protein for crystallisation screening is labor intensive and less amenable to automation. Therefore, the primary bottleneck of making high resolution protein: ligand crystals, creates a secondary bottleneck at the stages of protein expression and purification.
Protein expression
With automation, a large number of constructs can be made easily, allowing the inclusion of different hosts, vector backbones, promoters, fusion partners, purification tags, and cleavage sites. Rational or combinatorial design of constructs to rescue protein expression can further increase the number of constructs made. Several different approaches to high-throughput cloning have been optimised for different robotic platforms9-11. One such approach uses GatewayTM (Invitrogen) cloning technology that we adapted to the Biomek FX and NextGen EF platforms, allowing simultaneous production of 192 expression plasmids in 11 working days12. The number of constructs made ultimately should be determined by the down stream capacity for expression testing and protein purification.
Protein expression is a multidimensional, empirical problem that relies on individual experiences and serendipity for successful outcomes. Due to the large number of variables to explore, comprehensive screening and optimisation of expression becomes labor intensive, creating a bottleneck for rapid production of protein samples for crystallisation screening. This constraint can skew exploration of protein expression in favor of “easy-to-express” proteins. These choices can hardly be afforded when going after a validated therapeutic target. Screening for increased expression can be done at the protein and genetic levels, and at the expression host and growth parameter levels. A number of screening approaches have been developed for optimisation of genetic constructs for improved soluble protein expression; however, host-related expression variables are too numerous to be screened systematically. While different solutions exist for medium- to high-through-put protein expression13-17, development of a “universal” system is less likely. Rapid screening and optimisation of protein expression can have a profound impact on downstream protein purification and crystallisation strategy. Although some automation has been developed to assist in protein purification, it continues to be labor intensive, with each protein needing “individualised” care for maximal yield18-22. Automated purification approaches are likely to perform better with highly expressed constructs; therefore, in order to leverage automated purification, it is critical to screen and optimise expression levels.
Clonal selection – in vivo screening
Protein length and termini are the primary sequence variables considered when searching for maximal protein expression; changing the start and stop of the open reading frame can drastically alter how well the ribosome reads through the message and how soluble the expressed protein is in the cell and during purification. Other methods for changing expression outcomes include changing the promoter used, changing codon usage to match the expression host, and making specific mutants to alter the nature of the expressed protein. In an effort to rapidly screen through dozens or even thousands of different expression constructs, several methods of clonal selection or directed evolution have been developed23.
Genetic selection of highly expressed proteins in E. coli based on a “split GFP” system has been successfully used24. This system exploits the reconstitution of the fluorescent GFP through self association of fragments of GFP. A short peptide of the GFP (residue 214-230) is fused at the C-terminus of the target protein. This construct is co-expressed with the larger GFP fragment (residues 1-214). Upon expression, association of the two fragments results in reconstitution of green fluorescence, thus reporting soluble expression of the target protein. This system is similar to the LacZα complementation system of β-galactosidase25.
The twin-arginine translocation (TAT) pathway in E. coli also holds promise for genetic selection of soluble and well-folded proteins that are translocated into the periplasmic space26. In this system, the protein of interest is expressed as a tripartite fusion between an N-terminal Tat signal peptide and a C-terminal TEM1 β-lactamase reporter protein. Presence of β-lactamase in the periplasmic space confers antibiotic resistance on Gram-negative bacteria. E. coli grown in the presence of ampicillin thus can report successful expression and folding of the fused partner.
High-throughput expression testing – in vitro screening
Different prokaryotic and eukaryotic systems have been used for heterologous protein expression12. E. coli and baculovirus-mediated insect cell systems are predominantly used for structural biology purposes. Yeast and mammalian cell based alternative expression systems have also been successful in producing significant amounts of protein for structural analysis27. Also, as costs drop, cell free expression systems are making a resurgence to support structural biology28.
Due to the biological complexity of expression hosts and the intrinsic attributes of individual proteins, a generalised approach to effectively sample “expression space” appears unlikely. In order to allow optimisation of target-specific expression conditions, The Automation Partnership (TAP), in collaboration with a consortium of protein expression experts, developed the PICCOLO system to facilitate rapid screening of protein expression29. This fully integrated platform automates expression and single-column purification of up to 1152 unique culture samples using seed cultures, basic media, and either E. coli or insect cells. The heart of this platform is a 24 well culture vessel block (CVB) and its aeration assembly that supports culture densities up to 20 OD units at 600 nm. Each CVB can process 24 unique 10 ml cultures of E. coli or 12.5 ml cultures of insect cells and the system can handle 48 CVBs over 5 days to screen expression. Expression levels are quantified off-line by analyzing the His- or GST-tagged purified protein samples. Follow-up runs on PICCOLO can be used to process identical cultures to produce large scale growths, thereby obviating growth and expression issues often encountered when taking large scale growths off-line.
This platform has the potential to not only rapidly identify optimal expression conditions but also to minimise errors due to user intervention. The PICCOLO software includes a dynamic scheduling engine that efficiently manages the screening campaign and robustly handles error recovery. In addition, all of the experimental and culture parameters can be recovered throughout the run.
Several examples of the utility of PICCOLO have now been reported. TAP successfully screened expression of β-galactosidase in E. coli, showing minimal variance in cell growth within or between the CVBs29. Impact of host strain and culture conditions were successfully screened for six different proteins, using different strains of E. coli, and 720 culture conditions in quadruplicate30. Finally, expression screening in insect cells as well as E. coli has been performed on a number of proteins with positive results (Dawn Hall, personal communication). While PICCOLO like platforms may be part of the answer, more robust expression systems need to be developed.
Crystallisation of protein: ligand complexes
Significant efforts and resources are often invested in producing well-diffracting crystals of protein: ligand complexes. For structure-guided drug design, the key endpoint in protein crystallisation is to find the best set of conditions that yield a crystal that diffracts well and can enable ligand-bound structures in a facile manner. While screening for optimal crystallisation conditions now uses multiple approaches and high-throughput technologies, the entire process continues to suffer from a “hit-or-miss” approach31-33.
The large number of variables associated with crystallisation contribute to its uncertainty; these include the crystallisation reagent, the protein buffer, the protein itself, and physical parameters of the experiment. The presence of ligands during purification can have a significant impact on the stability and solubility of the protein, hence influencing crystallisation. Addition of a ligand can have a dramatic effect in the crystallisation of complexes34,35. However, the intrinsic properties of ligands can add to the uncertainty of crystallisation. Ligand solubility and intrinsic binding constants often dictate the feasibility of forming effective complexes.
Three commonly used approaches for rapidly producing structural information of complexes are “soaking” of ligands in ligand free (apo) form of the protein crystal, “back exchange” of a ligand previously bound to the protein with the ligand of choice, and “co-crystallisation” of protein and ligand (Figure 2). The “soaking” approach, if feasible, is the method of choice since it offers minimal barriers to ligand binding. Successful binding is often exclusively dictated by the solubility of the ligand in soaking buffer and by the intrinsic potency of the ligand. Availability of well-diffracting crystals of the apo protein is critical for the “soaking” approach; however, crystal packing and ligand-induced conformational changes can hamper the use of such an approach. The “back exchange” method can be used in solution when the presence of ligand is necessary for protein stability and solubility or in crystal form when optimal protein crystals can be obtained only in the presence of a ligand. Feasibility of this method depends on the solubility, intrinsic binding, and the kinetics of exchange of ligands. The “co-crystallisation” approach, although least desired, is often the only choice when significant conformational changes or crystal packing preclude other approaches. The need to optimise crystals for each ligand complex makes this approach resource and time intensive. At times, the chemical nature or the size of the ligand may even warrant screening new crystallisation conditions.
Automating the process to rapidly determine protein-ligand structures can significantly reduce the timelines for providing structural information for lead optimisation. Different laboratories have developed locally optimised strategies ranging from automation of critical steps to complete automation36. Here we describe the use of one such approach to support structure guided lead optimisation of BACE inhibitors.
Examples from BACE-1
Crystallisation efforts on BACE-1 (β-site amyloid precursor protein cleaving enzyme), a target for Alzheimer’s disease37, highlight the benefits of exploring multiple clones and the use of the “back exchange” approach for rapid turnaround of structure determination. Several different constructs of BACE-1 were tested for expression and protein purification38. The wild-type enzyme (residues 43-454) resulted in crystals as a complex with a bound ligand (compound 1, Figure 3.a) that diffracted to 2.5 Å resolution. Using “minimisation of surface entropy”39 as a driver for optimisation of crystals, several mutant forms of BACE (43-454) were screened for improved crystals (Table 1). One construct with two mutations (K136A and E138A) was identified that resulted in protein: ligand crystals that diffract to 1.6 Å resolution40. The crystals of the complex were optimised with compound 1. Using these crystals, the “back exchange” approach has been used to determine more than 200 structures of bound ligands with different chemical templates (Figure 3b)41,42. Among the ligands successfully exchanged are linear extended as well as macrocyclic templates ranging in IC50 values from low nanomolar to 150 µM. While apo forms of BACE subsequently have been reported in the literature43, none of our constructs yielded well-diffracting and robust crystals of the apo form. The rapid turnaround of structural information on BACE using the “back exchange” approach has been central to advancing development of novel BACE-1 inhibitors that show Aβ reduction in animal models43.
Conclusion
The lead optimisation component of small molecule based drug discovery has benefited significantly from advances in high-throughput crystallography. In the recent past, structural genomics efforts paved the way for development of high-throughput technologies that were eventually adapted in the pharmaceutical sector. Automated solutions for different aspects of gene-to-structure have significantly reduced the timelines for determining crystal structures during the early stages of drug discovery. Reaching this endpoint hinges on the availability of crystallisable protein and optimised protein: ligand crystals. Continued advances in high-through-put expression and crystallisation screening platforms should ease these bottlenecks.
References
- Abola, E., et al. Five years of increasing structural biology throughput – a retrospective analysis. .In Structure-based Drug Discovery, H. Jhoti and A. Leach ed. Springer, Netherlands. 2007, pp., 1-26.
- Blundell, T.L. & Patel, S. High-throughput X-ray crystallography for drug discovery. Curr. Opin. Pharmacol. 2004, 4:490-496.
- Congreve, M., et al. Keynote review: Structural biology and drug discovery. 2005, 10:895-907.
- Chandonia, J.-M. and Brenner, S. E. The Impact of Structural Genomics: Expectations and Outcomes. Science 2006, 311: 347 – 351.
- Rupp, B. High-throughput crystallography at an affordable cost: The TB Structural Genomics Consortium Crystallisation Facility. Acc. Chem. Res. 2006, 36: 173-181.
- Liu, Z., et al. The high throughput protein-to-structure pipeline at SECSG. Acta Cryst. 2005, D61:679-684.
- Heinemann, U. et al. Facilities and Methods for the High-Throughput Crystal Structural Analysis of Human Proteins. Acc. Chem. Res. 2003, 36(3):157-163.
- Service, R.F. Structural Genomics. Trapping DNA for structures produces a trickle. Science 2002, 298:948-950.
- Hartley, J. Cloning technologies for protein expression and purification. Current Opinion in Biotechnology 2006, 17:359-366.
- Alzari P.M., et al. Implementation of semi-automated cloning and prokaryotic expression screening: the impact of SPINE. Acta Cryst. 2006, D62:1103-1113.
- Acto, T.B. et al . Robotic cloning and Protein Production Platform of the Northeast Structural Genomics Consortium. Methods Enzymol. 2005, 394:210:243.
- Kornienko, M., et al. Protein Expression Plasmids Produced Rapidly: Streamlining cloning protocol and robotic handling. Assay and Drug Development Technologies 2005, 3: 661:674.
- Yin, J., et al. Select what you need: A comparative evaluation of the advantages and limitations of frequently used expression systems for foreign genes. J. Biotechnol. 2007, 127(3):335-347.
- Loomis, K.H., et al. InsectDirectTM System: rapid, high-level protein expression and purification from insect cells. Journal of Structural and Functional genomics 2005, 6:189-194.
- Finley, J.B., et al. Structural genomics for Caenorhabditis elegans: high throughput protein expression analysis. 2004, 34:49-55.
- Kosta, T,. et al. Baculovirus as versatile vectors for protein expression in insect and mammalian cells. Nature Biotechnology 2005, 23:567-575.
- Hedrén, M., et al. GRETA, a new multifermenter system for structural genomics and process optimisation. Acta Cryst. 2006, D62, 1227-1231.
- Malawski, G.A., et al. Identifying protein construct variants with increased crystallisation propensity–A case study. Protein Sci. 2006, 15:2718-2728.
- Nguyen, H. et al. An automated small-scale protein expression and purification screening provides beneficial information for protein production, J. Struct. Funct. Genomics 2004, 5:23–27.
- Scheich, C., et al. An automated method for high-throughput protein purification applied to a comparison of His-tag and GST-tag affinity chromatography, BMC Biotechnol. 2003, 3-12.
- Schäfer, F.R., et al. Automated high-throughput purification of 6*his-tagged proteins, J. Biomol. Tech. 2002, 131–142.
- Draveling, C., et al. SwellGel: an affinity chromatography technology for high-capacity and high-throughput purification of recombinant-tagged proteins, Protein Expr. Purif. 2001, 22:359–366.
- Hart, D.J. and Tarendeau, F. Combinatorial library approaches for improving soluble protein expression in E. coli. Acta Cryst. 2006, D62:19-26.
- Cabantous, S., et al. Protein tagging and detection with engineered self-assembling fragments of green fluorescent protein. Nature Biotechnology, 2005, 23:102-107.
- Wigley, W. C., et al. Protein solubility and folding monitored in vivo by structural complementation of a genetic marker protein. Nature Biotechnol. 2001, 19:131-135.
- Fischer, A.C., et al. Genetic selection for protein solubility enabled by the folding quality control feature of the twin-arginine translocation pathway. Protein Sci. 2006, 15:449-458.
- Aricescu, A. R. et al. Eukaryotic expression: developments for structural proteomics. Acta Cryst. 2006, D62:1114-1124.
- Vinarov, D.A., et al. Wheat germ cell-free platform for eukaryotic protein production. FEBS J. 2006, 273(18), 4160-4169.
- Wollerton, M., et al. Automation and Optimisation of Protein Expression and Purification on a Novel Robotic Platform. JALA 2006, 291:303.
- Jones, J.J., et al. Impact of high throughput technology on recombinant protein production. Microbial Cell Factories, 2006, 5(suppl 1):S30.
- Stevens, R.C. High-throughput protein crystallisation. Curr Opin Struct Biolo. 2000, 10(5):558-563.
- Berry, I.M. et al. SPINE high-throughput crystallisation, crystal imaging and recognition : current state, performance analysis, new technologies and future aspects. Acta Cryst. D62:1137-1149.
- Li, F., et al. Automated high-throughput nanoliter-scale protein crystallisation screening. Anal and Bioanal Chemistry 2005, 383:1034-1041.
- Hassell, A.M., et al. Crystallisation of protein-ligand complexes. Acta Cryst. 2007, D63:72-79.
- Vidadi, M. et al. Chemical screening methods to identify ligands that promote protein stability, protein crystallization, and structure determination. PNAS 2006, 103:15835-15840.
- Mooji, W. T. M., et al. Automated protein-ligand crystallography for structure-based drug design. ChemMedChem 2006, 1:827-838.
- Gosh, A.K., et al. Recent developments of structure based b-secretase inhibitors for Alzheimer’s disease. Curr. Top. Med. Chem. 2005, 5:1069-1622.
- Sardana, V, et al. A general procedure for the purification of human b-secretase expressed in E. coli. protein Expression and Purification 2004, 34:190-196.
- Derewenda, Z.S. and Vekilov, P.G. Entropy and surface engineering in protein crystallisation. Acta Cryst., 2006, D62:116-124.
- Coburn, C.A., et al. Identification of a small molecule nonpeptide active site b-secretase inhibitor that displays a nontraditional binding mode for aspartyl protease. J. Med. Chem. 2004, 47:6117-6119.
- Stachel, S.J., et al. Structure based design of potent and selective cell-permeable inhibitors of human b-secretase. J. Med. Chem. 2004, 47:7447-6450.
- Rajapakse, H. A., et al. Discovery of Oxadiazoyl Tertiary Carbinamine Inhibitors of b-secretase, 2006, 49:7270-7273.
- Patel, S., et al. Apo and inhibitor complex structures of BACE. J. Mol. Biol. 2004, 343:407-416.
- Stanton, M.G., et al. Discovery of isonicotinamide derived b-secretase inhibitors: in vivo reduction of b-amyloid. J. Med. Chem. 2007, 50(15):3431-3433.
Sanjeev Munshi
Department of Structural Biology, Merck, Westpoint, PA
Sanjeev Munshi is a protein crystallographer and presently Director in the department of Structural Biology at Merck, West point, Pennsylvania. Sanjeev received his Ph.D. in 1989 from the Molecular Biophysics Unit of Indian Institute of Science, Bangalore, India. Sanjeev was a post-doctoral fellow at Purdue University in Prof. Jack Johnson’s laboratory where he focused on structure and function of viruses. Prior to joining Merck in 1995 Sanjeev worked at NCI-Frederick on the structure guided design of HIV protease inhibitors. At Merck Sanjeev continues to focus on integration of structural biology in early phases of lead discovery and optimisation.
Tim Allison
Department of Structural Biology, Merck, Westpoint, PA
Tim Allison is a protein crystallographer in the department of Structural Biology at Merck, West Point, PA. He received a B.S. in chemistry and biochemistry from the University of Miami and a Ph.D. in biochemistry from the University of Virginia. He was then a post-doctoral fellow at the National Institutes of Health in Rockville, MD.