Label-free quantitative proteomics: Why has it taken so long to become a mainstream approach?
Posted: 13 June 2013 |
In recent years, mass spectrometry (MS) based proteomics has moved from being a qualitative tool (used to mainly identify proteins) to a more reliable analysis tool, allowing relative quantitation as well as absolute quantitation of a large number of proteins. However, the developed quantitative methods are either specific for certain types of samples or certain types of mass spectrometers. In some cases, developing expertise on how to use a given method may take a long time and the use of these methods is therefore limited to few laboratories. Other quantitative methods are suitable for simple standard protein mixes which are far from the complexity of real samples. As a consequence, the number of available quantitative methods is high and choosing the right one is challenging.
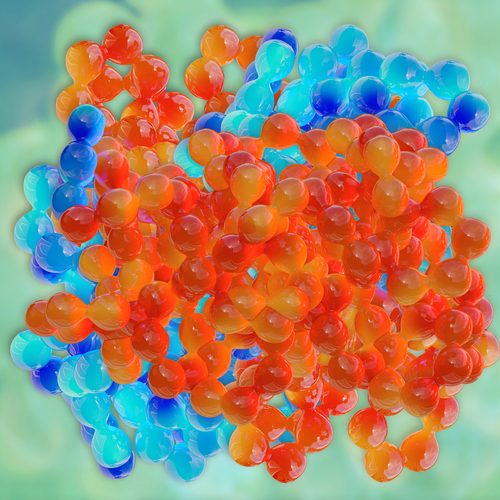
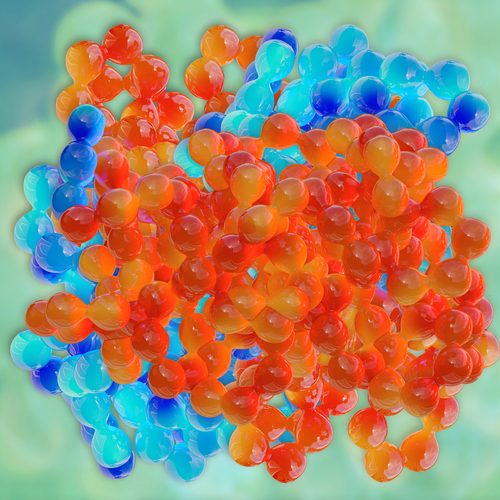
There are several different quantitative strategies which mainly fit into two main categories:
- Based on the use of a stable isotope in the form of in vivo labelling such as metabolic labelling and SILAC or chemical labelling such as iCAT and iTRAQ
- Label-free quantitation (either intensity based or spectra count).
Methods based on the use of heavy stable isotopes allow mixing several samples together (ranging from two to eight samples in one analysis). When doing so, each sample is encoded with a different stable isotope either within the peptide bonds (in vivo labelling) or with chemical groups (in vitro labelling). The principle can be shortly described as follows: one sample can be labelled with a chemical group based on 12C atom (e.g., a dimethyl group 2x12CH3) while the other sample can be labelled with the same chemical group but based on 13C atom (2x13CH3). Both samples consist of similar peptides which are encoded with relatively similar chemical groups but only differ at the mass level. Each peptide will be detected by the mass spectrometer as a ‘doublet’ (a light form based on 12C atoms and a heavy form based on 13C atoms in that example). The intensity of each peak of the ‘doublet’ provides information on the relative peptide abundance between sample 1 and 2.
In the case of in vivo labelling, sample preparation involves the use of relatively expensive reagents. Additionally, it usually requires a good knowledge of culture media components and has therefore been mainly applied to simple and well characterised model organisms although some effort has been made in higher animal models such as mice and chicken. In the latter case, the culturing media is supplemented with compounds containing stable heavy isotopes which are then incorporated within the newly synthesised proteins. In vitro labelling (or chemical labelling) also suffers from several issues which include reagent cost and the specificity of the labelling, although quite good, is sometimes not complete.
Most labelling strategies increase the complexity of the samples, since each peptide will exist as a ‘doublet’ or a ‘triplet’, for example. This increase in complexity by introducing peptide redundancy can decrease the number of protein identification (thus quantitation) significantly. This problem is, however, absent in the case of isobaric labelling strategies such as iTRAQ and TMT. Isobaric reagents have the same masses in MS mode, the sample decoding and quantitation is performed after ion fragmentation in MSMS mode.
Probably one of the most promising commercial applications of proteomics is to improve biomarker discovery which involves the analysis of human tissue samples including high numbers of conditions and replicates. These two aspects make most of the labelling strategies unsuitable for such an approach. Probably as a direct consequence, the initial interest for having a quantitative method which does not involve any form of labelling was developed by the industry, e.g., by Thermo Finnigan1, SurroMed2, MDS-Proteomics3 and Caprion4. Lately, several academic groups have taken up this trend and developed their own label-free platform such as the group of Mann with Maxquant5, Yates with Census6 and SuperHirn from Aebersold & Müller7. Some commercial platforms have also been developed such as SIEVE (www.thermo.com), Progenesis (www.nonlinear.com) and the Rosetta Elucidator to name only a few.
Although there are two different label-free quantitation modes, within this article, we will mainly refer to the intensity based approach. To the reader who is interested in spectra count, we recommend the following review8. Major progress in protein quantitation was accomplished by moving from 2D gel based (relative quantitation done visually and protein identification done by MS) to a gel-free shotgun proteomics where complex samples are analysed and the quantitation is directly performed at the MS level.
A label-free approach based on peak intensity generally includes integration of the chromatographic peak areas for any given peptide in LC-MS runs and linking those ’chrompeaks’ or ‘features’ to the different LC-MS traces (i.e., peak alignment across the different runs). The peak volume is generally proportional to the peptide concentration. In a simplified manner: protein abundance for a given sample can be defined as the intensity sum of all peptides found in this sample that are unique to that protein. Some main advantages of using such a label-free approach are: (i) almost no limitation in terms of range of experimental conditions that are possible (unlike to most of the labelling strategy); (ii) comparison of unequal numbers of LC-MS runs representing two or more different conditions in the range of 10 to 100 of samples is feasible; (iii) almost any type of sample can be used (‘universal aspect’, contrary to any in vivo labelling strategy); (iv) the absence of peptide redundancy associated to heavy stable isotope labelling. Although more proteins can be identified (thus hopefully could result in a higher number of peptide quantitation), several label-free studies still combine the use of sample fractionation at different levels (e.g., cell organelles, proteins or peptides). This fractionation level (at the peptide or protein level) can be challenging to deal with during the data analysis stage. The fractionation has to be robust and a reproducible process. A common strategy is to compare the different samples at the fraction level (i.e., fraction 1 of sample 1 is compared with fraction 1 of sample 2 and so on, the process is repeated for each fraction). The capability of linking ‘chrompeaks’ to the different samples is highly dependent on the instrument mass accuracy and resolution which has significantly improved within the last few years.
However, no quantitation strategy is perfect. One of the earlier arguments against label-free quantitation, which probably hampered its initial development, was the assumption that ion suppression will interfere significantly with the quantitation process. The importance of ion suppression as a significant factor interfering with quantitation has been reviewed in the work of Gangl et al9 who suggested that this issue is negligible under a low flow rate regime. The use of low flow rates are typical conditions of most of the standard proteomics study. Other more significant challenges encountered in a label-free quantitation are associated with the importance of linking the same chrompeak properly to the different LC-MS runs (i.e., the chromatography and the mass spectrometer behaviour has to be reproducible). Contrary to any labelling strategy, there is no sample ‘encoding’ for a label-free analysis, therefore each sample has to be analysed individually, which adds some analysis time and the technical variability has to be assessed and reduced as much possible. Using such a strategy involves the need of reproducible sample preparation as well as reproducible and robust chromatography which necessitates a more extensive initial preparation. Ideally, sample run orders are randomised and it is preferable to run a complete study within a short time range to avoid technical variations associated to temporal variation observed at the HPLC and mass spectrometer level (i.e., changing columns, pre-columns, MS detector variations over time). Those issues have been previously discussed in order to address the importance of randomisation in the experimental design10. The fact that the samples are not mixed together at any step in the process compared to a label-based experiment suggests a more accurate measurement in a label-based analysis than in a label-free analysis. However, this assumption has been challenged in an Association of Biomolecular Resource Facilities (ABRF) study where different quantitative methods have been compared (label-based and label-free)11.
One important aspect of a label-free quantitation is to ensure a proper normalisation strategy. Ideally, the amount of samples and its nature are quite similar across the overall study. Data normalisation can be performed across each LC-MS trace either by extracting the overall peak intensities or using the median or average of all MS peaks. When comparing different groups of samples, several strategies exist, although the simplest one is to use a one-way ANOVA. Under those conditions, ratio intensity and p-value are the common extracted parameters.
The typical quantitative proteomics analysis is based on the use of criterions which are considered ‘acceptable’ but are not often supported by any statistical analysis. Such studies often make use of ’biological triplicates’, a ratio intensity cut-off of 1.5 to 2 and p-value of 0.5. A more rational and statistically established approach should be considered such as the ones suggested by Levin12 and Karp and Lilley13 which is based on power analysis. In such cases, the number of replicates needed and the applied cut-off is not defined randomly but depends on the quality of the data obtained. By doing so, one will be able to report meaningful small differences between sample groups (or intensity ratio close to 1) and to acquire higher number of replicates under experimental conditions which are reproducible. In that case, the main variation contributing to the noise will be the inherent variation of the biological replicates.
The main approach in the proteomics field up to now is often described as a ‘discovery mode’ as no a priori hypothesis is made regarding the composition of the samples. Although an interesting approach, it is strongly biased towards the most abundant proteins. The identification of a high number of proteins in a given sample often involves several fractionation processes. These processes can be time consuming and are often performed at the expense of exploring other dimensions (biological variation, time series, different stresses conditions etc.).
A strategy that is becoming more popular consists of generating a list of potentially differentially expressed proteins using a limited number of replicates, fractions and very few conditions (ideally two extreme conditions) using any quantitative method described above. Those candidate proteins which are limited in number can then be studied in more detail using a targeted MS approach across a broader range of conditions. Such an approach is based on the use of Single Reaction Monitoring (SRM) or Multiple Reaction Monitoring (MRM) which, due to their more targeted nature, allows more detailed analyses of the proteome without requiring intensive fractionation in order to detect less abundant peptides as the ‘discovery mode’ would need.
Conclusion
Early label-free MS quantitation capabilities were limited to only a few laboratories with high resolution MS instruments, computer and bioinformatics capacities in order to be able to process the large amount of peaks (to detect, align and quantify these across the several LCMS runs). In addition, label-free quantitation was not globally accepted by the scientific community. During the last years, several MS vendors came with affordable high mass accuracy and high resolution mass spectrometers combined with a higher number of available software (either free or relatively accessible). Also computing power and infrastructure rendered the label-free technique more popular and eventually more accepted by the scientific community. According to an analysis of the scientific literature performed by Evans et al14, label-free quantitation analysis had a higher publication volume in 2012 compared to other quantitative methods such as iTRAQ and SILAC method. Despite this trend, there are still specific applications which can only be performed using a label-based approach such as protein turn-over analysis by in vivo labelling … at least for now.
References
- Chelius, D. and Bondarenko, P. V. (2002). Quantitative profiling of proteins in complex mixtures using liquid chromatography and mass spectrometry. J. Proteome. Res. 1 317–323
- Wang W, Zhou H, Lin H, Roy S, Shaler TA, Hill LR, et al. Quantification of proteins and metabolites by mass spectrometry without isotopic labeling or spiked standards. Anal Chem. 2003;75:4818-26
- Stewart, II, Zhao L, Le Bihan T, Larsen B, Scozzaro S, Figeys D, et al. The reproducible acquisition of comparative liquid chromatography/tandem mass spectrometry data from complex biological samples. Rapid Commun Mass Spectrom. 2004;18:1697-710
- Kearney P, Thibault P. Bioinformatics meets proteomics-bridging the gap between mass spectrometry data analysis and cell biology. J Bioinform Comput Biol. 2003;1(1):183-200.
- Cox J & Mann M. MaxQuant enables high peptide identification rates individualized p.p.b. range mass accuracies and proteome-wide protein quantitation (2008) Nat. Biotechnol. 26, 1367-1372
- Park SK, Venable JD, Xu T, Yates JR 3rd. A quantitative analysis software tool for mass spectrometry-based proteomics. Nat Methods. 2008 Apr;5(4):319-22. doi: 10.1038/nmeth.1195. Epub 2008 Mar 16
- Mueller LN, Rinner O, Schmidt A, Letarte S, Bodenmiller B, Brusniak MY, et al . SuperHirn – a novel tool for high resolution LC-MS-based peptide/protein profiling. Proteomics. 2007;7(19):3470-80
- Neilson KA, Ali NA, Muralidharan S, Mirzaei M, Mariani M, Assadourian G, et al . Less label, more free: approaches in label-free quantitative mass spectrometry. Proteomics. 2011;11(4):535-53
- Gangl ET, Annan MM, Spooner N, Vouros P. Reduction of signal suppression effects in ESI-MS using a nano-splitting device. Anal Chem. 2001 Dec 1;73(23):5635-44
- Bukhman YV, Dharsee M, Ewing R, Chu P, Topaloglou T, Le Bihan T, et al Design and analysis of quantitative differential proteomics investigations using LC-MS technology. J Bioinform Comput Biol. 2008;6(1):107-23
- Turck CW, Falick AM, Kowalak JA, Lane WS, Lilley KS, Phinney BS, et al ; Association of Biomolecular Resource Facilities Proteomics Research Group. The Association of Biomolecular Resource Facilities Proteomics Research Group 2006 study: relative protein quantitation. Mol Cell Proteomics. 2007;6(8):1291-8
- Levin Y. The role of statistical power analysis in quantitative proteomics. Proteomics 2011; 11, 2565-2567
- Karp NA, Lilley KS. Design and analysis issues in quantitative proteomics studies. Proteomics. 2007;7 Suppl 1:42-50
- Evans C, Noirel J, Ow SY, Salim M, Pereira-Medrano AG, Couto N, et al An insight into iTRAQ: where do we stand now? Anal Bioanal Chem. 2012;404(4):1011-27
Biography
Dr. Thierry Le Bihan trained in Biophysics and worked in Toronto in early 2000 in the biotechnology industry at MDS-Protana. He was actively involved in the development of the in-house label-free quantitation platform of MDS-Protana. He moved to set up a functional proteomics laboratory at the CFIBCR at Princess Margaret (University of Toronto). In 2007, Thierry Le Bihan relocated to Edinburgh where he established an academic research group in quantitative proteomics within the Centre for Systems Biology at Edinburgh (now SynthSys). After exploring several different quantitative strategies (15N metabolic labelling and the develop ment of new labelling chemistry strategy based on di-Ethylation), he now mainly concentrates his effort on the use of label-free protein quantification. Dr. Le Bihan uses label-free methods to study the stress response of Ostreococcus tauri, one of the most primitive unicellular algae in order to understand how this organism adapts under low nutrient conditions and to stresses combination. He also maintains several collaborations with different research groups from clinicians to astrobiologists. Dr. Le Bihan is also a member of ‘Scientists without Borders’ and he is interested in some of the global challenges we face and possible sustainable solutions (oil and phosphate peaks, food security and the need to maintain and understand biodiversity).
Issue
Related topics
Biomarkers, Label-free screening, Mass Spectrometry, Proteomics