Transforming pharmaceutical manufacturing: The AI revolution
Posted: 9 January 2024 | Dr Gonesh Chandra Saha (Bangabandhu Sheikh Mujibur Rahman Agricultural University) | No comments yet
The integration of artificial intelligence (AI) in pharmaceutical manufacturing is set to open a brand-new chapter in this industry’s development, as well as unprecedented opportunities for strengthening quality control and improving decision-making. In this article, Dr Gonesh Chandra Saha, Head of the Department of Computer Science & Information Technology at Bangabandhu Sheikh Mujibur Rahman Agricultural University, Bangladesh, considers questions such as AI’s application, effects, benefits and the requirements from the industry, and offers a future outlook for pharma manufacturing.
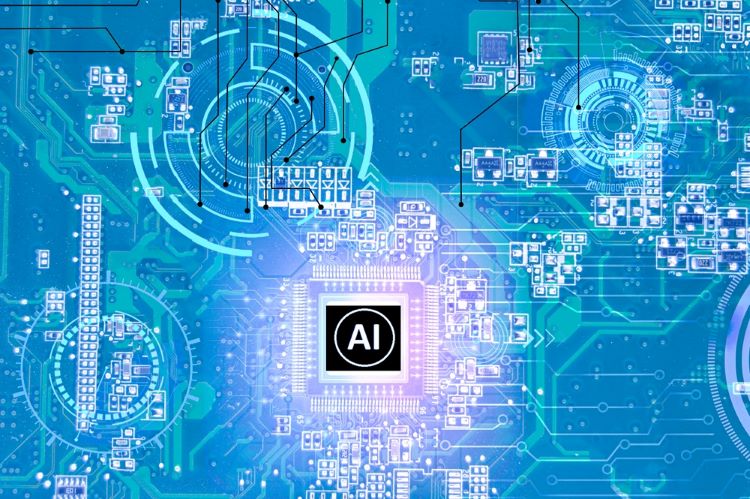
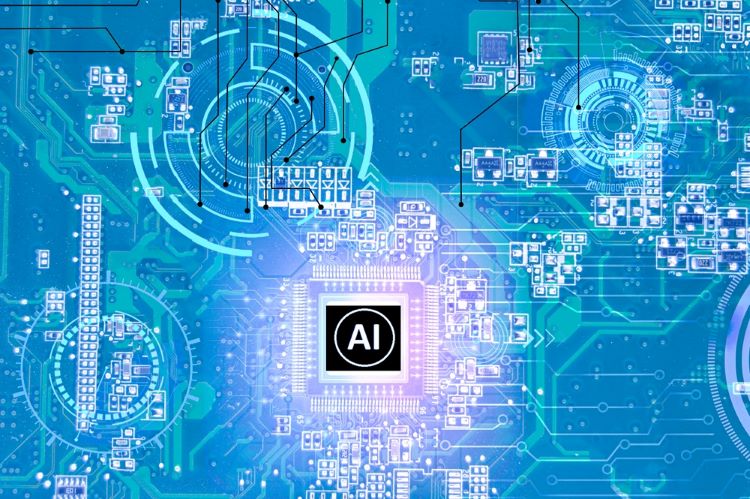
Revolutionising quality control
In the backdrop of stringent quality standards and regulatory demands inherent to pharmaceutical manufacturing, the addition of AI technologies introduce a paradigm shift. This article highlights the manifold applications of AI, particularly cutting-edge image recognition and computer vision systems, which profoundly impact quality control. These innovations bestow visual inspection processes with unprecedented precision, identifying imperfections and anomalies that often elude human scrutiny.
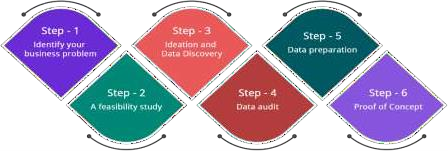
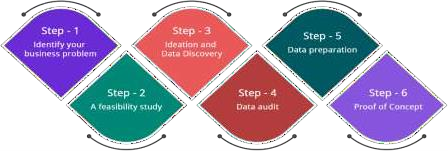
Figure 1: adapted from Saha, G. C. (2023). Integrative Analysis of Multi-Omics Data with Deep Learning: Challenges and Opportunities in Bioinformatics. Tuijin Jishu/Journal of Propulsion Technology, 44(3), 1384-1392.
With the added benefit of Internet-of-Things (IoT)-enabled real-time monitoring, AI can maintain constant compliance with stringent quality standards that tends to data integrity. Considering the transformative power of data, by drawing on an abundance of information and making best use of emerging technologies, we see the potential for reshaping decision-making.
By screening through huge amounts of data, as well as crunching those not suitable for the totally automated mode along with them at a time, AI provides stakeholders actionable information that they can use in strategic planning and resource allocation. The technology’s predictive capabilities are employed to anticipate and avoid potential risks arising from supply chain variables, changes in regulations, or irregularities caused by quality issues. Thus, project progress can proceed at a much quicker rate than through traditional manual testing whereby each step is awaited prior to the posting of new results.
Challenges and considerations of artificial intelligence
when already established manufacturing systems integrate AI, it is important to plan carefully so that there are no disruptions in operations”
Nevertheless, there are pitfalls in the path of adopting AI for pharmaceuticals manufacturing. The accuracy of the model depends on a variety of different datasets, and this is essential to determining whether AI systems will be effective. Another challenge is regulatory compliance, where AI systems must meet stringent requirements for validation of the data used and quality assurance. In addition, when already established manufacturing systems integrate AI, it is important to plan carefully so that there are no disruptions in operations.
Our research paper ‘Artificial Intelligence in Pharmaceutical Manufacturing: Enhancing Quality Control and Decision Making’ published in Rivista Italiana di Filosofia Analitica Junior in August 2023, provides ample examples of the use of AI in pharmaceutical manufacturing, explaining how it can raise levels and improve ease in quality control processes as well as decision-making. Through exploration of various applications, crises and case studies, the paper takes a look at how AI is promising to take pharmaceuticals forward toward ever-higher standards for quality control, compliance and operational efficiency.
AI and future perspectives: a glimpse into tomorrow
The future of AI in pharmaceutical manufacturing holds tremendous promise, with several key perspectives emerging:
The future of AI in pharmaceutical manufacturing holds tremendous promise… [eg,] Personalised medicine… Continuous manufacturing… Advanced quality control… Regulatory compliance”
Personalised medicine and drug development: Some people predict that drug development will be transformed by AI to design drugs developed specifically for an individual patient, which should lead to more effective treatment.
Accelerated drug discovery: Simulations and predictive models powered by AI will speed up the drug development process, effectively slashing time to market for drugs.
Continuous manufacturing optimisation: The real-time process adjustment capabilities of AI will be essential to fully optimising quality control and production efficiency.
Advanced quality control: In the future, quality control systems using AI are expected to be capable of inspecting for even slight product defects while still meeting the strictest standards set out by government regulations.
Regulatory compliance and auditing: Regulatory compliance systems will become simpler to operate and enforce, thanks to automation performed by AI-driven systems.
Adding these developments with the addition of connected IoT devices, supply chain restructuring and consideration for our code of ethics will give us a glimpse at how pharmaceutical production will look in future.
Conclusion: shaping a new era of excellence with AI
AI… [in] pharmaceutical manufacturing… simplifies operations and improves product quality”
Lastly, the application of AI to pharmaceutical manufacturing would be a revolutionary step in guaranteeing quality control and sound decision-making throughout the industry. The numerous ways in which AI can transform the pharmaceutical manufacturing process are becoming clear, as it simplifies operations and improves product quality. One of its most useful aspects is that answers to many questions come readily from data analysis.
The development of AI in and its application to the production process are all going to lead us into a new era where drugs are safer, more effective, and focused on treating patients. Nonetheless, this transformation demands careful consideration of issues related to data privacy, model confidence and general applicability as well as the requirement for specialised expertise. With such efforts in tandem, AI can enter a fresh era of quality control and decision-making for the pharmaceutical manufacturing process.
About the author
Dr Gonesh Chandra Saha is the Head of the Department of Computer Science & Information Technology at Bangabandhu Sheikh Mujibur Rahman Agricultural University in Bangladesh. As an Associate Professor and accomplished researcher, his expertise lies in Computational Biology, AI, ML, and ICT in Agriculture, with a notable focus on “Integrative Analysis of Multi-Omics Data with Deep Learning: Challenges and Opportunities in Bioinformatics.”
References
- Saha GC. Integrative Analysis of Multi-Omics Data with Deep Learning: Challenges and Opportunities in Bioinformatics. Tuijin Jishu/ Propuls. Technol. 2023; 44(3):1384-1392.
- Artificial Intelligence and Machine Learning in Software as a Medical Device. [Internet] US Food and Drug Administration (FDA). 2019. [cited 2023Dec]. Available from: https://www.fda.gov/medical-devices/software-medical-device-samd/artificial-intelligence-and-machine-learning-software-medical-device
- Rajkomar A, Dean J, Kohane I. Machine Learning in Medicine. N Engl J Med. 2019; 380(14):1347-1358.
- Shickel B, Tighe PJ, Bihorac A, et al. A Survey of Recent Advances in Deep Learning Techniques for Electronic Health Record (EHR) Analysis. IEEE J Biomed Health Inform. 2018; 22(5):1589-1604.
- Kourou K, Exarchos TP, Exarchos KP, et al. Machine Learning Applications in Cancer Prognosis and Prediction. Comput Struct Biotechnol J. 2015; 13:8-17.
- Ranschaert ER, Morozov S. Defining the Future of Radiology Through Artificial Intelligence: The Birth of a New Subspecialty. Eur J Radiol. 2016; 85(4):2048-2050.
- Jamshidi N, Eslami M. Artificial Intelligence and the Future of Workforce. J Am Coll Radiol. 2019; 16(9):1297-1299.
- Angermueller C, Pärnamaa T, Parts L, Stegle O. Deep Learning for Computational Biology. Mol Syst Biol. 2016; 12(7):878.
- Ching T, Himmelstein DS, Beaulieu-Jones BK, et al. Opportunities and Obstacles for Deep Learning in Biology and Medicine. J R Soc Interface. 2018; 15(141):20170387.
- Zhou J, Troyanskaya OG. Predicting Effects of Noncoding Variants with Deep Learning–Based Sequence Model. Nat Methods. 2015; 12(10):931-934.
Related topics
Artificial Intelligence, Big Data, Big Pharma, Biopharmaceuticals, Clinical Development, Clinical Trials, Continuous Manufacturing, Data Analysis, Data integrity, Drug Development, Drug Manufacturing, Industry Insight, Manufacturing, Regulation & Legislation, Research & Development (R&D), Technology, Therapeutics