Enhancing rapid microbiology methods: how AI is shaping microbiology
Posted: 22 December 2021 | Tim Sandle (Bio Products Laboratory) | No comments yet
Rapid microbiological methods are being enhanced by artificial intelligence (machine learning in particular). The aim is to speed up analysis; increase accuracy; avoid error and introduce a level of automation. Examples include microscopy, colony counting, and microbial characterisation and matching – each of which is based on improvements in image analysis. Bio Products Laboratory’s Dr Tim Sandle explores further.
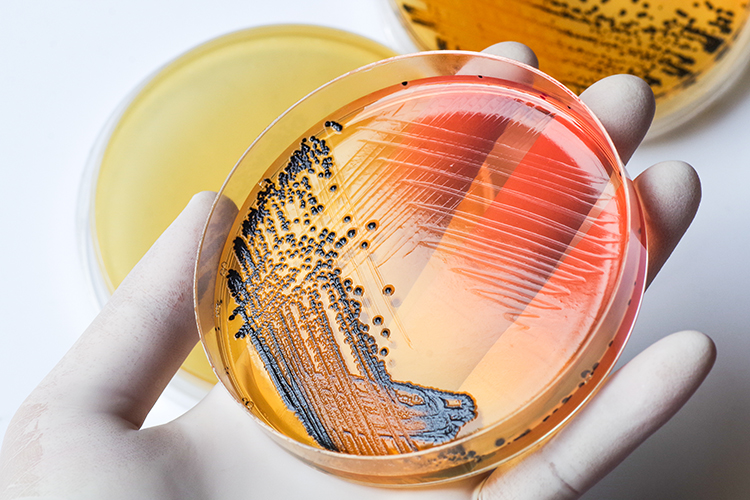
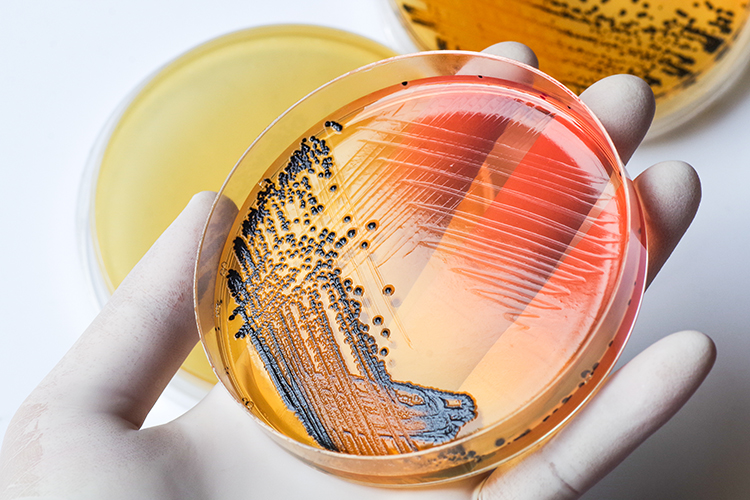
ARTIFICIAL INTELLIGENCE (AI), or more specifically machine learning (ML), is used in many aspects of microbiology research, including microbial classification problems, studying the interaction between microorganisms and the surrounding environment, and for microscopy. This article reviews some recent developments in this emerging field. While more progress has been made in the clinical microbiology space, these emerging technologies have the potential to re-shape aspects of rapid microbiology within the pharmaceutical and healthcare sectors too.
Machine learning
The subject of ML often has an air of mystery, given the concept of an ‘intelligent’ computer. While ML is fast and a well-designed and trained algorithm capable of delivering new insights; the concept can more easily be viewed as a “thing labeller; taking your description of something and telling you what label it should get”.1 Through this, instead of the classic computing approach of providing explicit instructions, the user programmes an algorithm with examples and the algorithm proceeds to find patterns in the data; transforming these into information and instructions that the user would likely not have found themself. The process is advanced in the initial stages by users guiding the algorithm as it learns what is correct and incorrect.
Machine learning is applied to microbiology at various stages of the development curve, including sample processing (pre-analytical, analytical and post-analytical process management), sample tracking, image acquisitions systems, smart incubation and workstation operation. There have also been advances with earlier detection of growth in broth and with headspace analysis, which require rapid accuracy, precision, and limit of detection analyses. Many of these have been designed to address issues of human error that arise from fatigue, high work volume that needs to be processed in a short time, and task repetition;2 thus overcoming some of the data integrity concerns that affect microbiology. Another area of application is drug development, especially with the design of new antimicrobials where ML can help to progress computationally intense problems such as predicting drug targets against specific microorganisms.
Characterisation and classification
In recent years, microbial sequencing has advanced through high-throughput sequencing technology, which has served to generate massive quantities of microbial data. While the majority of microbial methods performed in microbiology laboratories are phenotypic (biochemical or proteomic based), genotypic methods can prove useful for assessing sterility test and media fill failures, and for tracking the route of contamination as part of a contamination control strategy. Generally, but not exclusively, this is an outsourced activity (an ‘as a service’ concept provided over a network). However the task is undertaken, the ability of ML to find patterns has the potential to make a significant contribution to understanding types of microbial contaminants, their origins, and the relationship between organisms isolated in different areas or from different sources.3 This requires a mixture of supervised (for classification) and unsupervised (for pattern clustering) approaches to ML.
The advantage of ML is rapid detailed pattern analysis that cannot be easily achieved using conventional databases or spreadsheets”
The advantage of ML is rapid detailed pattern analysis that cannot be easily achieved using conventional databases or spreadsheets.4 An example of the ML approach is the placement of microorganisms into operational classification units such as grouping microorganisms according to the similarity of their DNA sequences. For contamination considerations, the main goal is to determine whether an unknown microorganism belongs to a specific species or not and whether two or more unknown organisms are related (or where an unknown organism is related to an organism previously characterised). This may reveal, for example, that microbial isolate A found on a machine bed within a Grade A zone matches microbial isolate B found from the finger plate of the operator who set up the filling line, and perhaps that the same operator recently recovered the same matched organism from an exit gown plate.
A different approach considers microbial interactions, such as predicting co-operative and competitive relationships within the same microbial population. Understanding the likelihood of interactions centred on neutralism, commensalism, synergism, mutualism, competition, amensalism, parasitism and predation,5 can indicate the likelihood of biofilm formation within a water system, for example.
As well as genotypic identification, ML also has the potential to advance the analysis of matrix-assisted laser desorption-ionisation/time of flight mass spectrometry (MALDI-TOF) mass spectral data, thereby applying AI to improve phenotypic characterisation.6
Microscopy
Microscopes enhanced with AI have the potential to aid microbiologists’ examination of organisms and use the collected data for diagnosis or root cause analysis. In a study, microbiologists at Beth Israel Deaconess Medical Centre have demonstrated that an automated AI-enhanced microscope system is “highly adept” at identifying images of bacteria quickly and accurately.7
The process involved using an automated microscope designed to collect high‑resolution image data from microscopic slides. The researchers trained a convolutional neural network (CNN) for the assessment. A CNN is a class of artificial intelligence modelled on the mammalian visual cortex. The CNN was used to analyse visual data and categorise bacteria based on their shape and distribution. The characteristics assessed represented common bacterial morphologies, including rod-shaped bacteria and coccoidal, the round clusters indicative of Staphylococcus species and the pairs or chains indicating Streptococcus species.
Microscopes enhanced with AI have the potential to aid microbiologists’ examination of organisms and use the collected data for diagnosis or root cause analysis”
The process of training was gradual, involving multiple images. This began with an unschooled neural network that reviewed more than 25,000 images from samples. By cropping these images, where the bacteria had previously been identified by human microbiologists, the research team generated more than 100,000 training images. Over time, the machine intelligence learned how to sort the images into the three categories of bacteria (rod-shaped, round clusters and round chains or pairs). At the end of the exercise, almost 95 percent accuracy was achieved.
For the subsequent phase of the study, the researchers challenged the algorithm to sort new images from 189 slides without human intervention. Overall, the algorithm achieved a 93 percent accuracy across all three categories. With further development and training, it is hoped this form of AI-enhanced platform could be used as a fully automated classification system in the future.
As additional functionality, the images can be sent remotely for microbiologists located in other parts of the world.
Colony counting
Colony counting may sometimes appear straightforward, but evidence shows that errors can happen either through failing to spot colonies due to their appearance, or fatigue.8 Advances in visual assessments of images using ML have led to new image systems with higher sensitivity. Such high‑resolution image analysis systems can detect small and mixed colonies, which a human eye cannot.
An effective, automatic and AI-driven colony counter should be capable of the following functionality:9
- Standardised and accurate results. Accuracy is important since colony counting can be affected by numerous parameters related to the physical properties of the colony: size, shape, contrast and overlapping colonies. Achieving this requires automatic colony separation (for when colonies are positioned close to each other)
- Counting colonies within appropriate parameters (such as down to 50 microns and measuring zones accurately to 0.5mm, within detection limits of 0.1mm)
- A good optical response performance (sufficient control of the background noise, contrast, resolution, etc) of the image acquisition tools
- Effective image resolution, file size/ data management, sample lighting and instrument uniformity
- Ability to visualise white light and fluorescent colonies
- The ability to differentiate between chromatic and achromatic images and thus deal with both colour and clear media
- Ability to separate aggregated colonies
- Ability to count the entire plate or sectors of the plate
- Obtain results within one second per plate
- The display of real-time full-colour on‑screen images
- Zoom function for looking at smaller colonies
- Software to allow for data collection and analysis. Data should ideally be transferrable to a laboratory information management system (LIMS).
Machine learning applications for colony counting are not completely reliable, although technology is progressing. The main obstacles are low image resolution; high CFU density; background noise; artifacts on the dish’s boundary; and CFUs located close to the boundary of a Petri dish.10 These issues are being addressed through repeated patterns of learning. The ML approach can also be used for other types of image analysis including Gram stains.
Summary
Applying ML to microbiological analyses can make tasks quicker and more accurate. In conjunction with automation, this can also lead to rapid microbiological methods that can help to overcome tedious, slow and error‑prone process. There will be barriers to the use of some of the technologies, necessitating conversations with regulators and addressing the concerns of laboratory analysts who are used to doing things in certain ways and with making certain decisions. Microbiologists may need to better understand perception, know‑how and infrastructure relating to all aspects of data handling. While these issues must be addressed, they are not insurmountable challenges. ML will be driven by access to data; in particular, accessing large, structured, interoperable and interconnected datasets. This article has presented a few examples of the ML applications that are further advanced. Ideally, in a few years’ time, these methods will be established and new technologies presented.
About the author
Dr Tim Sandle has over 25 years’ experience of microbiological research and biopharmaceutical processing. Tim is a member of several editorial boards and has authored 30 books on microbiology, healthcare and pharmaceutical sciences. Tim works for Bio Products Laboratory Limited (BPL) in the UK and is a visiting tutor at both the University of Manchester and UCL.
References
- Kozyrkov C. (2018) Explaining supervised learning to a kid (or your boss), Towards Data Science, at: https://towardsdatascience.com/explaining-supervised-learning-to-a-kid-c2236f423e0f
- Croxatto A, Prod’hom G, Faverjon F, et al. (2016) Laboratory automation in clinical bacteriology: what system to choose? Clin Microbiol Infect. 22(3):217-235
- Huang YA, You ZH, Chen X, et al. (2017). Prediction of microbe-disease association from the integration of neighbor and graph with collaborative recommendation model. Transl. Med. 15:209. doi: 10.1186/s12967-017-1304-7
- Zou Q, Lin G, Jiang X, et al. (2018). Sequence clustering in bioinformatics: an empirical study. Bioinform. bby090. doi: 10.1093/bib/bby090
- DiMucci D, Kon M, Segre D. (2018). Machine learning reveals missing edges and putative interaction mechanisms in microbial ecosystem networks. Msystems 3:e00181-18. doi: 10.1128/mSystems.00181-18
- Smith K, Wang A, Durant T, et al. (2020) Applications of Artificial Intelligence in Clinical Microbiology Diagnostic Testing, Clinical Microbiology Newsletter, 42 (8): 61-70
- Smith K, Kang A, Kirby J. (2017) Automated Interpretation of Blood Culture Gram Stains using a Deep Convolutional Neural Network. Journal of Clinical Microbiology, 2017; JCM.01521-17 DOI: 10.1128/JCM.01521-17
- Sande T. (2020) Ready for The Count? Back-To-Basics Review Of Microbial Colony Counting, Journal of Validation Technology, 26 (1): https://www.ivtnetwork.com/article/ready-count-back-basics-review-microbial-colony-counting
- Sandle T. (2018) Automated, Digital Colony Counting: Qualification and Data Integrity, Journal of GxP Compliance, 22 (2): https://www.ivtnetwork.com/article/automated-digital-colony-counting-qualification-and-data-integrity-0
- Falk T. (2019) U-Net: deep learning for cell counting, detection, and morphometry, Nature methods, 16 (1): 67-70
Issue
Related topics
Analytical techniques, Artificial Intelligence, Environmental Monitoring, Informatics, Lab Automation, Microbial Detection, Microbiology, QA/QC, Rapid Microbiological Methods (RMMs), Technology