Discovery and validation of protein biomarkers
Posted: 10 July 2012 |
Biomarkers are biological characteristics that are objectively measured and evaluated as indicators of normal biological processes, pathogenic processes or pharmacological responses to a therapeutic intervention. Biomarkers can be used to determine disease onset, progression, efficacy of drug treatment, patient susceptibility to develop a certain type of disease or predict efficacy of treatment at a particular disease stage. Protein molecular biomarkers are particularly popular due to the availability of a large range of analytical instrumentation, which can identify and quantify proteins in complex biological samples. Proteins are key compounds in biosynthesis, cell, tissue and organ signalling and provide cell and tissue structural stability in living organisms. The primary protein sequences are encoded in the genome; however, their complex posttranslational modifications (PTMs) and three dimensional structures are fairly unpredictable from genomic information. In this mini-review, we will provide an overview of the current state, challenge and important aspects of protein biomarker discovery and validation…
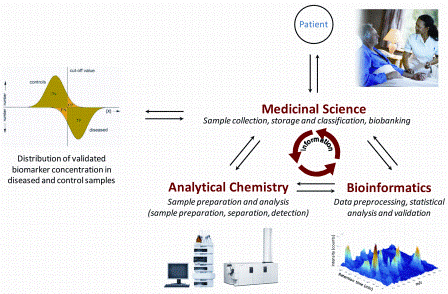
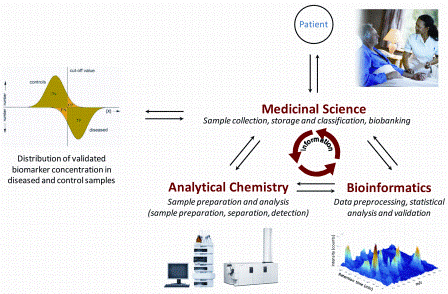
FIGURE 1: Parties involved in the biomarker discovery and validation process. Medical Science is responsible for sample collection, pre-classification and storage in biobanks. Analytical Chemistry is responsible for developing sample preparation protocols and analytical platforms both for comprehensive biomarker discovery on low numbers of samples as well as for the targeted validation in large sample cohorts. Bioinformatics is responsible for performing the data pre-processing and statistical analysis as well as the validation of data and clinical information provided by the analytical and medical partners. A close collaboration and information exchange is essential for the success of biomarker research
Biomarkers are biological characteristics that are objectively measured and evaluated as indicators of normal biological processes, pathogenic processes or pharmacological responses to a therapeutic intervention1. Biomarkers can be used to determine disease onset, progression, efficacy of drug treatment, patient susceptibility to develop a certain type of disease or predict efficacy of treatment at a particular disease stage2. Protein molecular biomarkers are particularly popular due to the availability of a large range of analytical instrumentation, which can identify and quantify proteins in complex biological samples.
Proteins are key compounds in biosynthesis, cell, tissue and organ signalling and provide cell and tissue structural stability in living organisms. The primary protein sequences are encoded in the genome; however, their complex posttranslational modifications (PTMs) and three dimensional structures are fairly unpredictable from genomic information. In this mini-review, we will provide an overview of the current state, challenge and important aspects of protein biomarker discovery and validation.
Biomarker research should start with defining the target normal biological processes, pathogenic processes, or pharmacological responses to a therapeutic intervention, what the biomarker should highlight. This is followed by setting up a team with medical, analytical and bioinformatics partners (Figure 1).
The medical partner is responsible for sample collection, storage and pre-classification of samples based on accurate clinical data (disease phenotype), and store samples and clinical information in biobanks. The analytical participant is responsible for the development of an analytical platform both for the discovery stage comprehensively measuring a low number of samples and for validation. Validation includes the targeted analysis of a preselected list of biomarker candidates in a large sample cohort. The bioinformatics partner is responsible for management, processing and accurate evaluation of all data acquired during the study. All of the three parties need to collaborate closely, and the work requires careful planning before starting the sample collection and analytical work3.
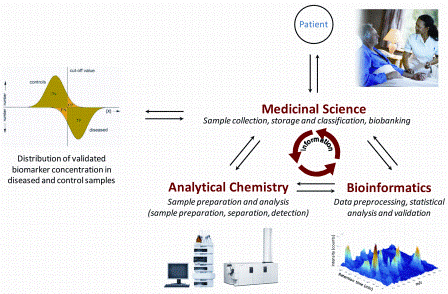
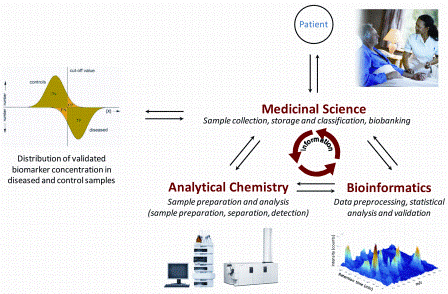
FIGURE 1: Parties involved in the biomarker discovery and validation process. Medical Science is responsible for sample collection, pre-classification and storage in biobanks. Analytical Chemistry is responsible for developing sample preparation protocols and analytical platforms both for comprehensive biomarker discovery on low numbers of samples as well as for the targeted validation in large sample cohorts. Bioinformatics is responsible for performing the data pre-processing and statistical analysis as well as the validation of data and clinical information provided by the analytical and medical partners. A close collaboration and information exchange is essential for the success of biomarker research
Contribution of the medical partner
The first step in biomarker discovery is the selection of the target biofluid, in which the final biomarker should be detected. In most cases, tissues or cells that are affected by pathological changes are not in direct contact with the target biofluid. Therefore, it is advisable to include an investigation of molecular changes such as up or down regulation of proteins or change of protein PTMs at the localisation involved directly by the investigated pathological process, and find out how this molecular change is translated to the target biofluid. This planning should be reflected in the sampling strategy, when samples are taken in a prospective manner. Most biomarker research is performed directly on human samples; however when the pathology is complex, slow developing or rare, animal experiments could replace discovery with human samples when a proper animal model is available. An example of this approach is the use of murine with experimental auto – immune encephalomyelitis to model the acute inflamma tory aspects of relapsing-remitting multiple sclerosis4. However, even with wellbehaved proper animal models it is necessary to validate the discovered biomarkers in humans. There is a huge worldwide endeavour for the collection of biospecimen such as tissues, organs and biofluids from patients with particular pathologies. These biobanks generally contain hundreds or thousands of well characterised samples collected over a long period of time and form an important resource for biomarker research. These biobanks offer the possibility to use samples collected retrospectively, however this implies that sample collection, storage and inherent registered clinical data are well-controlled and standardised5. In recent studies, biomarker discovery included testing the sample stability during collection, storage, sample preparation and analytical measurement6. Most of these studies are performed by testing the different parameters or so-called factors individually, which does not take eventual interaction between factors into account. An experimental design strategy for megavariate data, such as that obtained with proteomics and meta – bolomics experiments, should take interactions between factors into account. ANOVAsimultaneous component analysis should be applied in order to perform a comprehensive assessment of all factors from sampling until the final analysis7.
Other aspects to take into consideration when planning a sample cohort is the availability of samples, which could help to establish the specificity of biomarkers. For example, when the purpose of the biomarker is to diagnose a particular type of early stage cancer, the study should include samples not only from healthy donors and patients with the target cancer, but also from patients with other types of early stage cancer, which could have similar molecular changes or disorders concomitant with the target disease such as inflammation. Other aspects to consider are how to use samples from biobanks efficiently. The biomarker discovery part tries to measure comprehensively all proteins in the final target body fluid requiring complex and slow analytical platforms with low sample throughput. A frequently used strategy is the careful selection of a few well characterised individual samples. This strategy is often improved by collecting samples from the same patient for several conditions such as healthy and different cancerous stages, for example by taking blood samples from the same patient at the stages of cancer diagnosis and after successful treatment. Another less often applied approach is the use of a pool from multiple samples showing the same clinical characteristics. In this case it is necessary to prepare several pools of the same sample group to assess the biological variability of proteins.
Sample management should also consider reserving well characterised independent samples not used in the discovery study for validation. Validation can be performed either using the comprehensive discovery platform when a low number of samples are available for validation, however it is recommended to develop targeted analytical approaches with high sample throughput and analyse a much higher number of samples to establish the statistical power of the biomarker candidates. It is also recommended to obtain samples from different biobanks / clinics and perform the analytical validation in multicentre setup2.
The analytical platform
The role of the analytical platform is to provide data, which contains information on the identity and quantity of all molecular entities in the samples. Identity determination of proteins and natural peptides generally involves determination of the primary sequence. However the biological activity of proteins is also influenced by the second (irregular, α-helix and β-sheet), third (three dimensional structure) and quaternary structures (structure of complex formed by multiple proteins and cofactors). Protein and peptide biomarker research today mainly involves investigations of the primary structure, however a few emerging technologies such as activity based profiling of enzymes are trying to measure biological activity8. When biomarker discovery is performed without any hypothesis about the underlying molecular mechanism of the targeted biological process, the analytical platform is designed to be as comprehensive as possible. However, the molecular complexity in terms of identity (hundreds of thousands of different protein forms) and the large dynamic concentration range (11 order of magnitude in case of human blood) is so huge that this task is impossible to perform today and will require substantial advances in analytical technology. For that reason selection of compounds which may be involved in the molecular mechanism of the investigated biological process increases the chance of successful biomarker discovery. For example, carcinogenesis of cells influences glycosylation patterns of glycoproteins9. Therefore, selective enrichment of glycoproteins using lectin based solid phases or lectin arrays are popular approaches in cancer related biomarker research10.
Analytical platforms can be characterised by sample throughput, the number of compounds which can be identified unambiguously, and by the precision and dynamic concentration range of quantifications. Proteomic analytical platforms such as antibody arrays, liquid chromatography coupled to mass spectrometry with multistage fragmentation (LC-MSn) or twodimensional electrophoresis (2DE) differ in these characteristics. In addition, proteins are large compounds, therefore either they are analysed in intact form such as on protein arrays and 2DE, or using the so-called ‘shotgun’ approach by fragmenting proteins into smaller peptides using specific proteases such as trypsin prior to LC-MSn analysis. Protein arrays using proteinspecific antibodies and fluorescence detection of bound proteins is a relatively simple and fast method to identify and quantify protein. However, specificity of an antibody to detect a particular protein is sometimes poor and the resulting crosstalk is difficult or impossible to assess. The 2DE method offers a large separation space for proteins, but has a limited dynamic concentration range for quantification, low sample throughput and requires additional mass spectrometry analysis to determine protein identity. Shotgun approaches using LC-MSn are popular analysis platforms for comprehensive proteomics biomarker discovery11. LC-MSn analysis offers a relatively moderate sample throughput and provides quantification and identification in a single analysis. In LC-MSn analysis, peptide and protein identifications are provided in a narrower concentration range (2-3 orders of magnitude) of high abundant compounds compared to quantification performance (3-4 orders of magnitude)12. Another challenge is that shotgun proteomics in the LC-MSn approach provides identity and quantification of peptides and not for intact proteins and reconstruction of protein quantity and identity from the obtained data is often ambiguous.
None of the above-mentioned proteomics profiling methods are fully comprehensive in terms of protein identify and concentration. An often used strategy to increase the dynamic concentration range of peptide and protein identification and quantification and separation space of compounds is the application of fractionation or multidimensional chromato – graphy using orthogonal separation phases13. However, this comes at the expense of lower sample throughput due to the exponentially increasing analysis time for each fractionation level or chromatography dimension. When biomarker discovery is conducted targeting specific classes of proteins such as glycoproteins for cancer, then the already mentioned specific enrichment e.g. by using lectin solid phases is an often used strategy. Another challenge in the shotgun LC-MSn approach is to gain information on protein PTMs, which alter the activity and the biological function of proteins, and play a pivotal role in numerous biological processes, such as cellular physiology, cell and tissue signalling, the immune system, ageing, protein-protein interactions and disease onset and progression. PTMs of proteins are strongly influenced by environmental factors, diseases onset and progression, and are difficult to predict directly from the genome. There are more than 300 known PTMs of proteins ranging from simple modifications such as oxidation, phosphorylation, nitration, methylation, sulphonation and acylation to more complex types such as glycosylation or lipid modifications14. Identification of PTMs requires the use of nonconventional fragmentation methods such as higher-energy C-trap, electron transfer and electron capture dissociations, providing complex data, which are currently mainly interpreted manually15.
Bioinformatics
The role of bioinformatics in biomarker research is to extract protein and peptide identities – mostly primary sequence including eventual modifications – and quantitative information automatically from the large and complex data acquired by the analytical platforms. Further more, bioinformatics contributes to the selection of protein or peptide biomarker candidates with the support of statistical methods at the discovery phase and to establish a candidate’s statistical power on independent large sample set at the validation phase3. Bioinformatics algorithms are tailored for specific data, and therefore require different algorithms for processing protein array, 2DE or LC-MSn data. In general, bioinformatics workflows are complex and are influenced by the experimental design. For example, a workflow for the analysis of LC-MSn proteomics discovery data requires a preprocessing part with peptide / protein identification and quantification modules, a module to integrate quantification and identification information, a module for statistical analysis and a module to explore the potential involvement of the biomarker candidates in biosynthesis, regu latory or signalling pathways. Quantification modules may involve numerous elements, such as noise filtering, peak identification and quantification, time alignment, normalisation, mass calibration, matching the same peaks across multiple chromatograms to provide a non-exhaustive list of tasks. The current challenge is to assess the performance of these complex workflows accurately, which is supported by the growing availability of standard data with known quantitative and identity information of some or all of the protein components16. Current bioinformatics solutions are poor in the accurate extraction of quantitative and protein identification information and published new developments are often not included in a software environment that allows nonspecialists from the ‘wet laboratories’ to perform the data processing. A good example is the commonly used commercial database-centric peptide identification method for LC-MSn data17, which is poor in identifying unexpected PTMs. However a new series of alternative identification methods are emerging such as spectral libraries18 or archives19, spectral network analysis20 or open modifications search21 as well as de novo sequencing algorithms22 which are much better in detecting simple unexpected PTMs manifesting themselves as mass shifts in the spectra. There is an urgent need for further developments, for example to determine the primary structure of glycopeptides and glycans in an automated way or to develop efficient peak identity transfer approaches to increase the dynamic concentration range of annotated peaks in single stage LC-MSn data.
Bioinformatics should also consider management aspects of the large and complex data sets with variable data models and should deal with dynamic software environments to enable adjustment to different experimental designs. Future initiatives need to develop platforms, with data management, processing and evaluation abilities, similar to Galaxy23 used by the genomics community. This platform should include complex workflow building and assessment ability, integration of large parallel computational resources such as grids and clusters, storage resources and portals providing easy-to-use web pages to access processing services that can be used by non-bioinformatics experts. The availability of such a platform is indispensable to remove the current data processing bottleneck in biomarker discovery.
Conclusion
The complexity of human organisms in terms of molecular composition, concentration, activity and their changes in time makes biomarker discovery and validation extremely challenging and resulted in only a few novel diagnostic tests that have been approved by regulatory authorities such as US Food and Drug Administration (FDA) and the European Medicines Agency (EMEA)24. Biomarker research today is an emerging technology requiring a combined interdisciplinary approach from medical science, analytical chemistry and bioinformatics. We expect that the continuous development of technology in analytical chemistry and bioinformatics matched by the increasing number of well-controlled biobanks will contribute significantly to our under – standing of biological processes and to improving healthcare. Success in biomarker discovery can be improved considerably by focusing on key target biological processes of a disease such as a particular group of compounds involved in a given molecular mechanism. Approval by regulatory agencies such as FDA and EMEA of diagnostics test based on the validated biomarker must be taken into consideration at the beginning of biomarker projects.
References
- J. M. Danesh, R. Collins, P. Appleby, (2001) Biomarkers Definitions Working Group. Biomarkers and surrogate endpoints: Preferred definitions and conceptual framework. Clin Pharmacol Ther, 169. 416-468
- H. Mischak, G. Allmaier, R. Apweiler, T. Attwood, et al,. (2010) Recommendations for biomarker identification and qualification in clinical proteomics. Sci Transl Med, 2. 46ps42.
- P. L. Horvatovich, R. Bischoff, (2010) Current technological challenges in biomarker discovery and validation. Eur J Mass Spectrom, 16. 101-21
- R. A. Linker, P. Brechlin, S. Jesse, P. Steinacker, et al., (2009) Proteome profiling in murine models of multiple sclerosis: identification of stage specific markers and culprits for tissue damage. PLoS One 2009,4. e7624
- D. H. Jackson, R. E. Banks, (2010) Banking of clinical samples for proteomic biomarker studies: a consideration of logistical issues with a focus on preanalytical variation. Proteomics Clin Appl, 4. 250-70
- T. Rosenling, M. P. Stoop, A. Smolinska, B. Muilwijk, et al,. (2011) The impact of delayed storage on the measured proteome and metabolome of human cerebrospinal fluid. Clin Chem, 57. 1703-11
- A. K. Smilde, J. J. Jansen, H. C. Hoefsloot, R. J. Lamers, et al., (2005), ANOVA-simultaneous component analysis (ASCA): a new tool for analyzing designed metabolomics data. Bioinformatics, 21. 3043-8
- R. Freije, T. Klein, B. Ooms, H. F. Kauffman and R. Bischoff, (2008) An integrated high-performance liquid chromatography-mass spectrometry system for the activity-dependent analysis of matrix metalloproteases. J Chromatogr A, 1189. 417-25
- B. Adamczyk, T. Tharmalingam, P. M. Rudd, (2011) Glycans as cancer biomarkers. Biochim Biophys Acta. in press
- P. M. Drake, W. Cho, B. Li, A. Prakobphol, et al., (2010) Sweetening the pot: adding glycosylation to the biomarker discovery equation. Clin Chem, 56. 223-36
- R. Aebersold, M. Mann, (2003) Mass spectrometrybased proteomics. Nature, 422. 198-207
- A. Michalski, J. Cox, M. Mann, More than 100,000 detectable peptide species elute in single shotgun proteomics runs but the majority is inaccessible to data-dependent LC-MS/MS. J Proteome Res 2011, 10. 1785-93, DOI: 10.1021/pr101060v
- P. Horvatovich, B. Hoekman, N. Govorukhina and R. Bischoff, (2010) Multidimensional chromatography coupled to mass spectrometry in analysing complex proteomics samples. J Sep Sci, 33. 1421-37
- Y. Zhao, O. N. Jensen, (2009) Modification-specific proteomics: strategies for characterization of posttranslational modifications using enrichment techniques. Proteomics, 9. 4632-41
- J. Wiesner, T. Premsler, A. Sickmann, (2008) Application of electron transfer dissociation (ETD) for the analysis of posttranslational modifications. Proteomics, 8. 4466-83
- M. Sandin, M. Krogh, K. Hansson and F. Levander, (2011) Generic workflow for quality assessment of quantitative label-free LC-MS analysis. Proteomics, 11. 1114-24
- D. N. Perkins, D. J. Pappin, D. M. Creasy and J. S. Cottrell, (1999) Probability-based protein identification by searching sequence databases using mass spectrometry data. Electrophoresis, 20. 3551-67
- H. Lam, (2011) Building and searching tandem mass spectral libraries for peptide identification. Mol Cell Proteomics, 10. R111 008565
- A. M. Frank, M. E. Monroe, A. R. Shah, J. J. Carver, (2011) Spectral archives: extending spectral libraries to analyze both identified and unidentified spectra. Nat Methods, 8. 587-91
- N. Bandeira, D. Tsur, A. Frank, P. A. Pevzner, (2007) Protein identification by spectral networks analysis. Proc Natl Acad Sci, 104. 6140-5
- E. Ahrne, M. Muller, F. Lisacek, (2010) Unrestricted identification of modified proteins using MS/MS. Proteomics, 10. 671-86
- S. Kim, N. Gupta, N. Bandeira, P. A. Pevzner, (2009) Spectral dictionaries: Integrating de novo peptide sequencing with database search of tandem mass spectra. Mol Cell Proteomics, 8. 53-69
- J. Goecks, A. Nekrutenko, J. Taylor, (2011) Galaxy: a comprehensive approach for supporting accessible, reproducible, and transparent computational research in the life sciences. Genome Biol 2010, 11. R86 24. J. M. Rhea, R. J. Molinaro, (2011) Cancer biomarkers: surviving the journey from bench to bedside. MLO Med Lab Obs, 43. 10
About the authors
Péter Horvatovich is an Assistant Professor at the University of Groningen in the research group of Analytical Biochemistry at the Department of Pharmacy. After obtaining a Masters degree in analytical chemistry at the Eötvös Lóránd University in Budapest, he studied physical-chemistry at the University of Louis Pasteur. In 2001, Péter earned industrial experience at Chinoin. In 2003, he was awarded the prestigious joint scholarship of Alexander von Humboldt and Hertie foundation and performed two years postdoctoral research at the German Federal Institute for Risk Assessment in Berlin developing a method for detection of illegal use of recombinant somatotropin in lactating cattle. In 2005, he earned a postdoctoral position at the University of Groningen in the research group of Analytical Biochemistry led by Professor Rainer Bischoff. He developed an analytical platform for biomarker discovery of cervical cancer, and changed his research focus to proteomics related bioinformatics. Since January 2008 he has been working in proteomics related bioinformatics as an assistant professor. Peter’s research group has developed several time alignment algorithms for complex proteomics LC-MS data, data preprocessing workflows and contributed in the analysis of several biomarker discovery projects. Dr. Péter Horvatovich is an author of 33 peer-reviewed publications.
Rainer Bischoff is full professor and head of the Department of Analytical Biochemistry at the University of Groningen. He received his PhD in chemistry from the University of Gottingen. After spending time as a postdoctoral researcher at the Department of Biochemistry at Purdue University, he worked as a research scientist and group leader at Transgene S.A. He joined AstraZeneca R&D in 1998 where he started as leader of the Protein Chemistry Team with responsibility for protein purification and analysis within the Department of Cell & Molecular Biology and later as head of the Target Development Section. In 2001, he was appointed to the chair in Analysis of Biological Macromolecules at the University of Groningen. Professor Bischoff is a board member of the Netherlands Proteomics Platform and of the working Group on Pharmaceutical and Biomedical Analysis (FABIAN) of the Royal Dutch Chemical Society. He was chairman of the Analytical Chemistry study group of the Dutch Scientific Organisation (NWO) between 2008 – 2010. Professor Bischoff has authored approximately 150 peer-reviewed publications and holds 12 patents.