AI technology significantly improves human kidney analysis
Posted: 17 January 2018 | Dr Zara Kassam (European Pharmaceutical Review) | No comments yet
The ability to quantify the extent of kidney damage and predict the life remaining in the kidney is now possible using a computer model based on artificial intelligence…
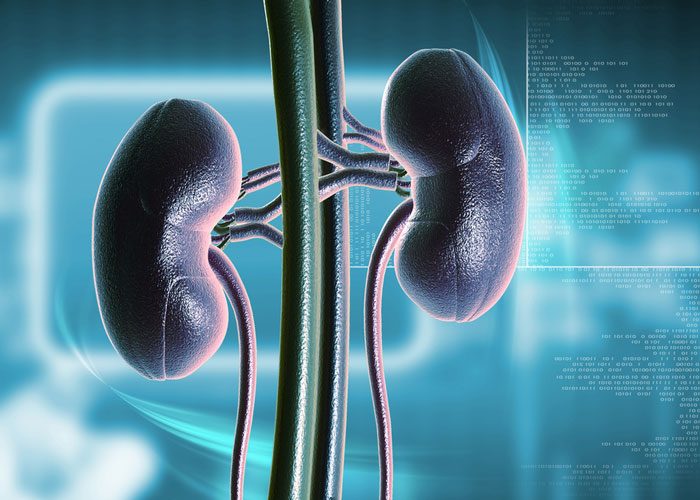
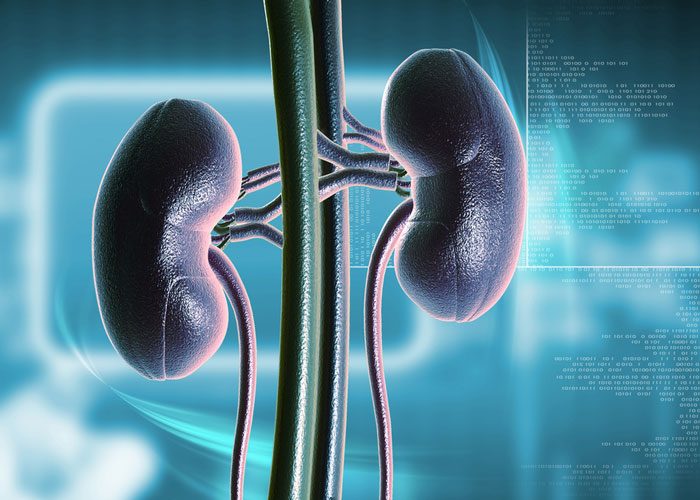
The ability to quantify the extent of kidney damage and predict the life remaining in the kidney, using an image obtained at the time when a patient visits the hospital for a kidney biopsy, now is possible using a computer model based on artificial intelligence (AI).
The findings can help make predictions at the point-of-care and assist clinical decision-making.
According to the researchers, the application of machine learning frameworks, such as convolutional neural networks (CNN) for object recognition tasks, is proving to be valuable for classification of diseases as well as reliable for the analysis of radiology images including malignancies.
To test the feasibility of applying this technology to the analysis of routinely obtained kidney biopsies, the researchers performed a proof of principle study on kidney biopsy sections with various amounts of kidney fibrosis (also commonly known as scarring of tissue).
The machine learning framework based on CNN relied on the pixel density of digitised images, while the severity of disease was determined by several clinical laboratory measures and renal survival. CNN model performance then was compared with that of the models generated using the amount of fibrosis reported by a nephropathologist as the sole input and corresponding lab measures and renal survival as the outputs. For all scenarios, CNN models outperformed the other models.
“While the trained eyes of expert pathologists are able to gauge the severity of disease and detect nuances of kidney damage with remarkable accuracy, such expertise is not available in all locations, especially at a global level. Moreover, there is an urgent need to standardise the quantification of kidney disease severity such that the efficacy of therapies established in clinical trials can be applied to treat patients with equally severe disease in routine practice,” explained corresponding author Dr Vijaya B. Kolachalama, Assistant Professor of Medicine at Boston University School of Medicine. “When implemented in the clinical setting, our work will allow pathologists to see things early and obtain insights that were not previously available,” said Dr Kolachalama.
The researchers believe their model has both diagnostic and prognostic applications and may lead to the development of a software application for diagnosing kidney disease and predicting kidney survival. “If healthcare providers around the world can have the ability to classify kidney biopsy images with the accuracy of a nephropathologist right at the point-of-care, then this can significantly impact renal practice. In essence, our model has the potential to act as a surrogate nephropathologist, especially in resource-limited settings,” said Dr Kolachalama.
The study appears in the journal Kidney International Reports