Researchers use data mining to expedite effective prescriptions for depression patients
Posted: 8 December 2017 | European Pharmaceutical Review | No comments yet
In a bid to maximise the effectiveness of treatment for patients, doctors have been using predictive models. New research finds that accessing data from electronic medical records could greatly benefit this practice.
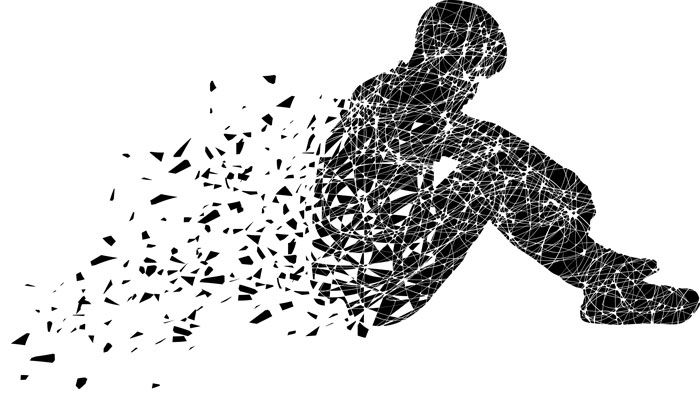
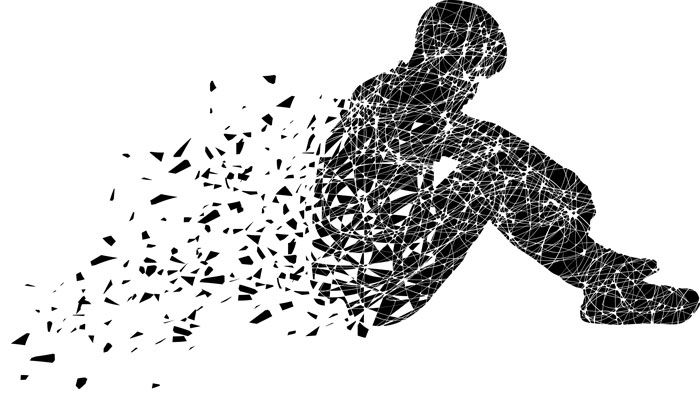
Personalised medicine has been one of the most promising medical developments in recent years and this entails determining which patients will benefit from various treatments.
A new study from Massachusetts General Hospital (MGH) and Harvard University finds that using data from electronic medical records in those models could hold a lot of promise.
Depression is a condition that is notoriously difficult to treat, with patients often having to try several different medications before finding an effective one.
Researchers – MGH’s Roy Perlis and Harvard’s Finale Doshi-Velez, joined by postdoctoral researcher Michael Hughes – examined large amounts of electronic data from the medical records of over 50,000 people with depression. By applying machine learning they were able to sort the data into depression subtypes – represented by clusters of symptoms and features highlighted by the data, such as age, sexual dysfunction, weight gain, or obesity.
Doshi-Velez‘s team also looked at 16 types of antidepressants that were ultimately successful in treating the patients’ depression. From these data, they were able to develop an algorithm to predict an antidepressant’s effectiveness based on depression subtypes.
When implemented in clinical settings, the new tool was found to be more accurate than existing methods. In addition, the clinicians polled by the researchers said they found the tool more user-friendly than existing predictive models.
As there is a 50 percent failure rate among patients trying a first treatment for depression, and a 50 percent failure rate among those who try a second type of treatment, cutting down the inherent guesswork could be life-changing.
Commenting on the value of more accurately pinpointing an effective treatment for patients, Doshi-Velez said: “Each try can take multiple weeks to assess, while you’re playing around with dosages. And these are already people for whom morale is sapped. Even if we could make a modest improvement – for instance, one less try – this could be a very significant result.”
While the researchers focused their study on mining records on depression, the method could apply more broadly to help patients with a variety of health problems – an exciting proposition for the industry and sufferers alike.
Related topics
Related organisations
Related people
Finale Doshi-Velez - Harvard University, Michael Hughes, Roy Perlis - Massachusetts General Hospital