Designing a novel continuous manufacturing plant with superior monitoring and control
Posted: 6 January 2016 | | 2 comments
There is a growing interest in manufacturing the pharmaceutical product continuously . Along with other advantages , it provides an appropriate platform to implement suitable monitoring and control architecture, to improve the product quality and minimise product rejection and operating expenses. Continuous pharmaceutical manufacturing can be also considered as a ‘data rich’ operation since data is continuously generated from the substantial experiments employed in the process development activities or process operation. Therefore, a systematic framework is needed through which suitable sensing and control architectures can be designed, developed, evaluated and implemented into a continuous pharmaceutical manufacturing plant. In addition, an efficient data management system is required for data storage, organisation and subsequent applications. The objective of this article is to introduce a continuous pharmaceutical manufacturing process and pilot plant, and highlight the process analytical technology (PAT), control and data management system integrated with it.
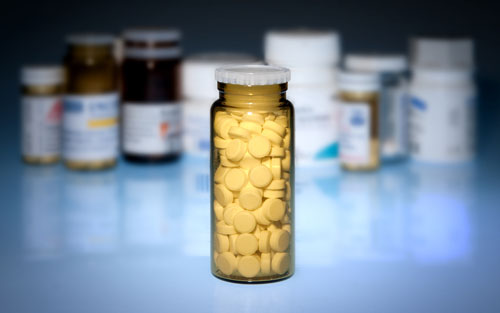
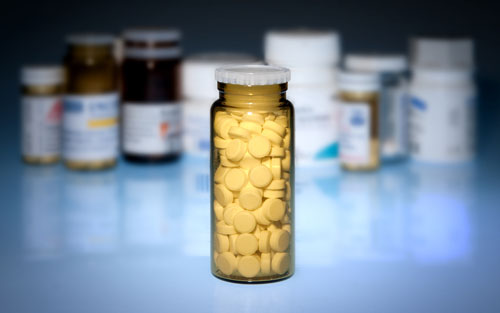
The pharmaceutical industry is a global business sector with around $1 trillion annual sales focused on performance-based products designed to address the healthcare needs of the world’s population. Traditionally, pharmaceutical products involving solid dosage forms were produced using batch processes. One major advantage of batch processing is flexibility of using the same equipment for multiple purposes. However, batch manufacturing processes have some inherent limitations that can be overcome through continuous manufacturing2, which itself offers the advantages of high efficiency, ability to enhance product quality, better controllability and reduced need for space, labour and resources.
Driven by these benefits and as a result of initiatives taken by United States National Science Foundation Engineering Research Center on Structured Organic Particulate Systems (C-SOPS), the pharmaceutical industry is currently undergoing a paradigm shift from batch to continuous manufacturing. The US Food and Drugs Administration (FDA) has also been very encouraging and supportive of the changes1. Consequently, most of the large pharmaceutical companies are in process of either building continuous pharmaceutical manufacturing plants or filing for approval to regulatory agencies such as the FDA. One continuous pharmaceutical manufacturing plant, described in this article, recently received regulatory approval, which indeed serves as a motivating example for this paradigm shift.
The benefits of harnessing the rich data accumulated in continuous pharmaceutical manufacturing is that a clear landscape about target product and process is generated, and the operational space of pharmaceutical processes optimised3. However, less attention has been given to data utilisation, as indicated by a survey which demonstrated less than 10% of data use by pharmaceutical companies. This can be traced to a lack of systematic organisation of the data. Furthermore, few approaches have been developed to extract information from such data sets automatically. To address this challenge, a general computational framework has been developed that allows the process data to be harnessed for the development of a continuous manufacturing process.
An integrated process flowsheet model of continuous pharmaceutical tablet manufacturing has also been developed and it has several applications such as virtual experimentations, process design, process optimisation, risk assessment, sensitivity analysis, feasibility analysis, flexibility analysis, design space generation, sensing and control architecture design4,5. The current focus is to further modernise continuous pharmaceutical manufacturing through integration of efficient in-line/on-line real-time monitoring tools, advanced feed-forward/feed-back control and systematic data management systems.
Flexible continuous tablet manufacturing process and pilot-plant
A closed-loop flexible multipurpose continuous tablet manufacturing process has been developed6. In this process, a typical pharmaceutical tablet can be produced via the different paths of direct compaction (route one), dry granulation using roller compaction (route two) or wet granulation (route three), and the most appropriate route is decided on based on the properties of the raw materials and the desired characteristics of the final product. Route one is the simplest process while routes two and three provides enhanced flowability characteristics of the powder blends; route two is particularly suitable for cases where powder feeds are moisture-sensitive. The process is described in detail in Singh et al.6 and has been previously extensively studied2,4,5.
A continuous direct compaction tablet manufacturing pilot plant has been installed and situated at C-SOPS, Rutgers University. A snapshot of the pilot-plant is shown in Figure 1; page 00. The pilot plant is built on three levels at different heights to take advantage of gravitational material flow. The top level is used for feeder placement and powder storage, the middle level is used for delumping and blending, and the bottom level is used for compaction. There are three gravimetric feeders – with the capability of adding more – that feed the various formulation components (active pharmaceutical ingredient [API], excipient, etc.). The feeders consist of a hopper, a load cell that is integrated with a gravimetric controller, and a conveying screw. A co-mill is also integrated after the feeder hopper, primarily to enable the powders to be de-lumped and to create contact between components.
Conical screen mills each consist of a cone-shaped screen with an impeller inserted into the center. The impeller rotates and material is ground between the impeller and the screen until it is small enough to pass through the holes in the screen and leave the mill. The co-mill eliminates any large, soft lumps within the powder, and the lubricant feeder is added after the mill to prevent over-lubrication of the formulation in the mill. These feed streams are then connected to a continuous blender within which a homogeneous powder mixture of all the ingredients is generated. Subsequently, the outlet from the blender is fed to the tablet press via a rotary feed frame. The powder blend fills a dye and is subsequently compressed to create a tablet. The NIR sensor for inline monitoring of blend uniformity, blend composition and blend density has been integrated through a chute placed in between tablet press and blender. The chute is specifically designed to provide an interface within which the new powder layer can form by displacing the old powder layers. The integration of the chute with the blender and sensor is reported in Singh et al.
Integration of PAT
Along with other monitoring tools, PAT has been integrated with the continuous pharmaceutical manufacturing plant described to enable real-time monitoring of critical process parameters. An advanced control system has then been added to control the critical process parameters to achieve desired critical quality attributes. The local level controllers are inbuilt in each feeder in order to control the powder flow rate. A ratio controller has been added that provides the flow rate set points of API, excipient and lubricant feeders for a given total flow rate and API composition7. Six supervisory control loops are added. The first loop is for the PAT-based feed-forward control which takes a corrective action for variations in the powder bulk density8. An NIR sensor together with real-time prediction tool has been used for inline monitoring of the powder blend density9. The signal of powder blend density has then been sent to the feed-forward controller that manipulates the fill cam depth of the tablet press proactively. The second loop is added to control the main compression force of the tablet press. This control loop is in a cascade arrangement with a master controller (loop three) specifically designed to control the tablet weight. The input of this master controller is weight and it generates the set point of the main compression force.
Loop four is designed to control the tablet hardness by manipulating the punch displacement8. Checkmaster (Fette) is used for monitoring the tablet weight and hardness. Loop five is added to control the powder level in the instrumented hopper. A webcam method is used for online monitoring of the powder level and the drug concentration is controlled through the PAT based on the sixth control loop7. The NIR sensor has been used for inline real-time monitoring of drug concentration and the powder bulk density and drug concentration is monitored using a single NIR probe9. Two partial least square (PLS) models are used to predict the powder bulk density and drug concentration separately.
In order to implement the control system into the pilot plant, the NIR spectra are sent to a real-time prediction engine that utilises the NIR calibration models for blend density and drug concentration and a real-time prediction tool (OLUPX) generates the signals for the control variables in real time7. The generated signals are then sent to a commercially-available control platform via an OPC where the combined FF/FB control loop has been implemented. The blend density is the input for the feed-forward controller while the blend composition together with powder level, tablet weight and hardness are the inputs of the feed-back control system9.
Efficient data management system
This framework includes data and ontology representation, search function and a multivariate data analysis (MVDA) algorithm (see Figure 2; page 00). The data representation can systematically represent process data, which is generated by different characterisation devices or operation units, as a set of human-readable specifications. These specifications can be organised as an XML file, which is a standard data vehicle for none-gap communication between various database/systems. Each data point is an XML file with unique filename as the ID, and in each XML file, the specifications are organised as a hierarchical structure whereby each node represents a specification, i.e., a specific measurement’s name with associated value. Ontology representation describes the relationship of terminologies referred in the data, e.g., paracetamol is a type of API. These terminologies and relationships are usually visualised as a hierarchical structure that has been discussed in detail10,11.
Ontology establishes logic interactions between different data to facilitate data search and selection.
The search function is developed to retrieve desired process data, and it consists of a user interface and search algorithm. The user interface allows the user to define which data should be studied and retrieved, e.g., API name, as well as the numerical criterion to be used for data searching, e.g., ±10% of defined viscosity. The ontology can also be used to structure the data. For example, Zhang (2011)12 described an example of harnessing strain ontology to search Chinese hamster ovary cell data, which could expand the search space for further data understanding. A search algorithm compares the user’s defined specifications with data points organised as individual XML files to confirm the data satisfies the user’s definitions, which would be used for information extraction. An example of the search algorithm and how it works for data searching is provided by Zhang and Hunter et al.11
Based on the returned data, MVDA algorithms serve to extract information. For example, clustering algorithms such as hierarchical clustering and K-mean clustering can quickly sort data into groups that allows users to understand commonalities/differences embedded in the data; regression algorithms, e.g., PLS, can harness returned data to generate the prediction model for estimated results. Such a prediction model could ensure the completeness of process data, e.g., the missing data can be predicted to facilitate process simulation and understanding.
Results and discussions
Continuous plant operation and process dynamic analysis
The dynamics of important continuous tablet manufacturing operating parameters along with PAT and control have been studied. Tablet press unit operation has been considered here as a demonstrative example. The production rate changed from 7500 tablets/h to 30,000 tablets/h. The set point, along with achieved production rate, is shown in Figure 3a (page 00). As shown in the figure, the achieved production rate is delayed in comparison to actual set point. The turret speed is also shown in Figure 3a (page 00). As expected, the production rate is proportional to the turret speed. The dynamics of fill depth is shown in Figure 3b (page 00). The fill depth has been changed from 8mm to 10mm. As shown in the figure, after initial lag time the fill depth approached the set point.
To study the effect of fill depth on critical process parameters such as main and pre-compression forces, the fill depth has been changed from 8mm to10 mm and back to 7mm. The achieved main compression and pre-compression forces are shown in Figures 3c-d (page 00). The achieved fill depth is also shown in the figure. As shown, the main and pre-compression forces follow the profile of fill depth with some delay times. The response of main compression force is relatively more delayed than pre-compression force response and it is essentially because the distance between filling station and main compression station is more in comparison to that of pre-compression station and filling station. Similarly, the dynamics of other process variables have been analysed. Based on the experimental data, a process model can be developed that can be used to analyse the process and design the control system.
The control hardware and software integration13 and closed-loop performance of the continuous tablet manufacturing plant has been previously described7, and the performance of combined feed-forward/feed-back control system has been evaluated by Singh et al8. The real-time monitoring of powder bulk density for FF/FB control has also been previously demonstrated9.
Efficient data management and applications
To illustrate how this framework works, a demonstration has been developed based on gPROMs, which is a dynamic process simulation platform. The flowchart of demonstration is given in Figure 4 (page 00), where the raw material properties data was stored in excel files that can be represented as XML files. Search function is developed to return relevant data satisfying users’ interests. This returned data is harnessed by PLS algorithm to generate predicted models for prediction of concerned parameters requested by flowsheet modelling in gPROMs. The function referred in this framework can be realised by the general programming languages, Python (i.e., data representation and search function) and C++ (i.e., PLS algorithm), which are selected due to conformability of gPROMs platform.
For a case study, the flowsheet model of continuous direct compaction tablet manufacturing process was used. The bulk density of powder coming out from the co-mill was considered for prediction using the PLS method because it is the key attribute and currently there is no other model available to predict it. Sixteen data points of powder properties were stored in the database, and the search function returned eight data points consisting of six PLS input variables: namely API, excipient, API concentration, excipient concentration, feeder throughput and co-mill rotation speed, and one PLS output variable – namely powder density. These returned data points were harnessed by PLS algorithm to establish a prediction model to predict powder density for the corresponding information defined in the simulation. The whole process and results are given in Figure 4 (page 00). The predicted density was calculated by experimental facts rather than theoretical assumption/calculation which makes the simulation results closer to the real situation.
Conclusions
A novel continuous pharmaceutical tablet manufacturing process integrated with real-time monitoring tools, advanced control and a systematic data management system has been developed. The process operation dynamics have been analysed. The data management framework described in this paper shows that reusing the process data for process development is a promising approach and a flexible one, which can easily be implemented and integrated with pharmaceutical companies’ current data management systems. It provides a cost-effective ‘big data’ strategy to accelerate a product’s commercialisation. Future work will include the implementation of a combined feed-forward/feed-back control system into the pilot-plant.
Acknowledgements
This work is supported by the National Science Foundation Engineering Research Center on Structured Organic Particulate Systems, through Grant NSF-ECC 0540855.
References
- Lee, SL, O’Connor, TF, Yang, X, Cruz, CN, Chatterjee, S, Madurawe, RD, Moore, CM V, Yu, LX, Woodcock, J. Modernizing Pharmaceutical Manufacturing: from Batch to Continuous Production. Journal of Pharmaceutical Innovation. 2015; 10, 191-199
- Singh, R, Ierapetritou, M, Ramachandran, R. An engineering study on the enhanced control and operation of continuous manufacturing of pharmaceutical tablets via roller compaction. International Journal of Pharmaceutics. 2012; 438 (1-2), 307-326
- Paul, SM, et al. “How to improve R&D productivity: the pharmaceutical industry’s grand challenge.” Nature reviews Drug discovery. 2010; 9(3): 203-214
- Boukouvala, F, Niotis, V, Ramachandran, R, Muzzio, F, Ierapetritou, M. An integrated approach for dynamic flowsheet modeling and sensitivity analysis of a continuous tablet manufacturing process: an integrated approach. Comput. Chem. Eng. 2012; 42, 30-47
- Singh, R, Ierapetritou, M, Ramachandran, R. System-wide hybrid model predictive control of a continuous pharmaceutical tablet manufacturing process via direct compaction. European Journal of Pharmaceutics and Biopharmaceutics. 2013; 85(3), Part B, 1164-1182
- Singh, R, Boukouvala, F, Jayjock, E, Ramachandran, R. Ierapetritou, M., Muzzio, F. (2012). Flexible Multipurpose Continuous Processing of Pharmaceutical Tablet Manufacturing Process. GMP news, European Compliance Academic (ECE), http://www.gmp-compliance.org/ecanl_503_0_news_3268_7248_n.html
- Singh, R, Sahay, A, Karry, KM, Muzzio, F, Ierapetritou, M, Ramachandran, R. Implementation of a hybrid MPC-PID control strategy using PAT tools into a direct compaction continuous pharmaceutical tablet manufacturing pilot-plant, International Journal of Pharmaceutics. 2014; 473, 38–54
- Singh, R, Muzzio, F, Ierapetritou, M, Ramachandran, R. A combined feed-forward/feed-back control system for a QbD based continuous tablet manufacturing process. PROCESSES Journal. 2015; 3, 339-356
- Singh, R, Román-Ospino, AD, Romañach, RJ, Ierapetritou, M, Ramachandran, R. Real time monitoring of powder blend bulk density for coupled feed-forward/feed-back control of a continuous direct compaction tablet manufacturing process. International Journal of Pharmaceutics. 2015; doi:10.1016/j.ijpharm.2015.09.029
- Venkatasubramanian, V, et al. Ontological informatics infrastructure for pharmaceutical product development and manufacturing. Computers & chemical engineering. 2006; 30(10), 1482-1496
- Zhang, J, et al. A logic-reasoning based system to harness bioprocess experimental data and knowledge for design. Biochemical Engineering Journal. 2013; 74: p.127-135
- Zhang, J, et al. Systematic Data and Knowledge Utilization to Speed up Bioprocess Design. Computer Aided Chemical Engineering. 2011; p.1351 – 1355
- Singh, R, Sahay, A, Fernando Muzzio, Ierapetritou, M, Ramachandran, R. Systematic framework for onsite design and implementation of the control system in continuous tablet manufacturing process. Computers & Chemical Engineering Journal. 2014; 66, 186-200
Biographies
Dr. Ravendra Singh is Assistant Research Professor at the Department of Chemical and Biochemical Engineering, Rutgers University, US. He is also serving as a manager of “multi million dollars, NSF ERC-SOPS projects”. He obtained his MS from IIT Roorkee India, and his PhD from Technical University of Denmark. He is the recipient of a prestigious EFCE Excellence Award given in Recognition of an Outstanding PhD Thesis, from European Federation of Chemical Engineering. He has published more than 43 research papers, written three book chapters, and presented at over 72 international conferences. He is lead guest editor of Journal of Chemistry special issue for PAT. He can be contacted at: [email protected] (phone: [001] 8484454944).
Dr. Jun Zhang obtained his BE from Tianjin University, China, his MS from Peking University, China and his PhD from University College London (UCL), UK. He completed his post-doctoral research at Rutgers University, New Jersey, US. He is currently employed at GlaxoSmithKline, UK. Major awards he won in 2010 and 2011 include Overseas Research Students Awards (from UCL), Bioprocess UK 2010 Conference Award for Poster Competition and UCL Graduation School Conference Award. He has published several papers and delivered presentations to national and international conferences. He can be contacted at: [email protected]
Dr. Marianthi Ierapetritou is a Professor and Chair of Chemical and Biochemical Engineering at Rutgers University. She obtained her BS from National Technical University in Athens, Greece (magma cum laude), her PhD from Imperial College London in 1995 and subsequently completed post-doctoral research at Princeton University (New Jersey) before joining Rutgers University in 1998. Among her accomplishments are the Outstanding Faculty Award in 2012, the Rutgers Board of Trustees Research Fellowship in 2004, and the prestigious NSF CAREER award in 2000. She has published over 190 papers and delivered over 200 presentations to national and international conferences. She can be contacted at: [email protected] (phone: [848] 445-2971).
Dr. Rohit Ramachandran is currently an Assistant Professor at the Department of Chemical & Biochemical Engineering, Rutgers University, in New Jersey (US). He completed his bachelors and masters degrees in Chemical Engineering at the National University of Singapore. This was followed by his PhD in Chemical Engineering at the Centre of Process Systems Engineering, Imperial College London. He then undertook postdoctoral work at MIT in the Process Systems Engineering Laboratory and the Novartis-MIT Center for Continuous Manufacturing. His research interests include modelling, control and optimisation of pharmaceutical and chemical processes. He can be contacted at: [email protected].
Pl send me full article. Thanks in advance.
Nice article.
Sandeep
Hi Sandeep, in order to read the full article you’ll need to register, which you can do here, Regards – Nick