Next generation sequencing: Using RNAseq to identify anti-cancer targets in the tumour vasculature
Posted: 15 December 2013 | | No comments yet
It is possible to attack the vasculature within solid tumours and achieve an anti-cancer effect. In the last decade, a number of studies have utilised cDNA libraries, SAGE analysis and microarrays to identify potential drug targets in the tumour endothelium. Modern sequencing technologies are likely to be a far more powerful and comprehensive tool for characterising the endothelial transcriptome.
Targeting the vasculature of solid tumours has been investigated as a possible therapy for cancer for over a century. The basic idea is that the tumour would be denied nutrients and oxygen once the blood vessels surrounding it are destroyed. This strategy is particularly attractive because the endothelial cells within blood vessels have intimate and direct contact with the blood and therefore the delivery of therapeutic agents should be comparatively easy1.
In 1993, Burrows and Thorpe2 provided proof of principle that it was possible to achieve an anti-cancer effect by targeting the blood vessels of subcutaneous tumours in mice. Their study used neuroblastoma cells expressing murine interferon γ (γIFN), the γIFN inflamed the tumour vasculature and it expressed MHC class II antigens. The MHC class II antigens were then targeted with an anti-mouse class II conjugated antibody, which delivered ricin to the tumour-associated endothelium. The result was haemorrhaging within the tumour, necrosis and tumour regression. Henceforth, the possibility was raised that a therapy could specifically target the vasculature within tumours by exploiting proteins that are only expressed in the endothelium.
Endothelial cells can be isolated from primary tissues (Figure 1), but they represent only a small proportion of cells within tissues. Therefore, the strategies employed to look at the RNA expression profile of endothelial cells must be robust when working with relatively small amounts of RNA, as it is not always possible to extract sufficient endothelial cells to give a significant RNA yield3. The conventional methods of exploring the endothelial transcriptome normal and tumour specific markers include serial analysis of gene expression (SAGE) and microarrays. However, it is now possible to use modern sequencing technologies to enrich our knowledge of the endothelial transcriptome and identify previously unknown potential targets.
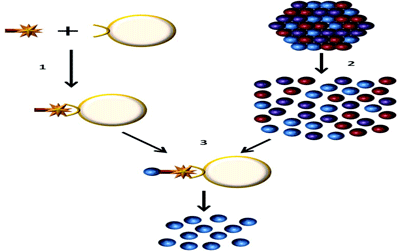
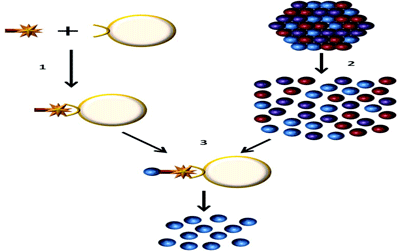
Figure 1: Endothelial cell isolation from primary tissues
1) The ulex lectin biotin complex is bound to streptavadin dynabeads
2) Primary tissue is disassociated to obtain a single cell suspension
3) The endothelial cells are isolated using the streptavadin dynabeads, the cells can then be lysed and the RNA extracted
The differences between ‘healthy’ and tumour endothelium
There are many factors that influence transcriptional changes in tumour associated endothelial cells. Some of these intrinsic differences are caused by environmental differences between tumours and ‘healthy’ tissue4,5. Blood vessels within tumours are often characterised as being structurally abnormal. The blood flow is often convoluted and impeded due to a lack of the normal hierarchical organisation between arteries, capillaries and veins5. This results in changes in the RNA and protein repertoires within endothelial cells, major influences are hypoxia6-8, low pH6,8 and low shear stress9.
Transcriptional changes within the endothelium can occur not only because of the environment within the tumour, but also because there are transcriptional differences between actively angiogenic and quiescent endothelial cells10. Angiogenesis in tumours is promoted by many secreted factors including the vascular endothelial growth factor (VEGF) pathway, which is one of the most significant regulators of angiogenesis by capillary sprouting (Figure 2)11,12. Angiogenesis is the process of developing new blood vessels from existing vessels. Angiogenesis has an essential role in wound healing and normal development. Conversely, angiogenesis can also be a precipitating factor in the etiology of many diseases such as cancer. Endothelial cells contribute to angiogenesis and when activated, contribute towards many regenerative and pathological processes. Recently, a small number of causative molecular differences have been characterised in endothelial cells from various pathologies, however many remain undefined4,11-14.
The behaviour of endothelial cells associated with different tissues and their seemingly preferential use of different angiogenic routes highlights the potential for finding tissue specific markers of the endothelium (and possibly tissue specific tumour endothelial cell markers). This could be caused by many factors such as the angiogenic mechanisms employed by the endothelial cells within certain organs. Intussusceptive (non-sprouting) angiogenesis is the predominant mechanism in the lung, where endogenous endothelial precursors contribute to angiogenesis. Intussusception can be caused by the proliferation of endothelial cells within a vessel to produce a wide lumen. This enlarged lumen can be split by fusion of capillaries and transcapillary pillars (the development formation of columns by thickened endothelial cells)14,15. Furthermore, environments where progressive tumour growth relies more heavily on angiogensis such as colorectal tumours could contain endothelial cells with a different gene expression profile3. A good example of this tissue specific TEM expression is the roundabout, axon guidance receptor, homolog 4 (ROBO4), ROBO4 is highly upregulated in the tumour endothelium in many tissues, but often not to the same degree in the colon.
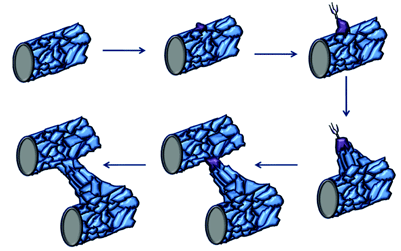
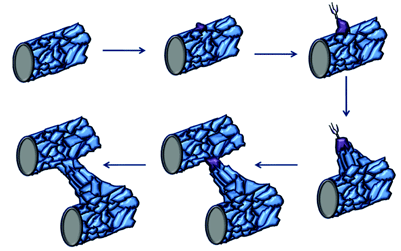
Figure 2: Tip cell formation, angiogenic sprouting and blood vessel growth. When endothelial cells in mature blood vessels are activated in response to angiogenic stimuli, they form tip cells. The tip cell will start to migrate once it has broken through the basement membrane and has lost contact with surrounding mural cells. These tip cells are followed by proliferating endothelial cells, which assemble to form capillary-like sprouts. The new sprouts will eventually fuse with themselves or existing blood vessels to establish blood flow. (Original diagram compiled using information from Adams and Eichmann [15])
Identification of tumour endothelial cell markers
By combining two data mining approaches, Huminiecki and Bicknell16 identified 16 genes specific to the endothelium including four novel endothelial specific genes. The first strategy utilised very high stringency BLAST searches against the ‘UniGene gene index’ to screen a pool of nine human endothelial libraries and 108 non-endothelial libraries from the ‘Expressed Sequence Tags database’ (dbEST). Secondly, the internet-based SAGE library subtraction SAGEmap exprofiler was employed. The cross referencing of the EST and SAGE library analyses was necessary to accurately identify genes preferentially expressed in the endothelium overcome the large numbers of false positives associated with each method individually. Although the main aim of this study was not to identify tumour endothelial specific markers, one of the four novel genes was the tumour specific marker ROBO4. This was possible because some of the endothelial libraries were derived from endothelial cells that were actively undergoing angiogenesis, such as HUVEC (human umbilical vein endothelial cells). ROBO4 was later shown to be tumour endothelial specific and play a role in angiogenesis by Huminiecki et al.17. Furthermore, the prospect of using ROBO4 as an anti-antigenic therapy and therefore anti-cancer therapy has been explored by Yoshikawa et al.18 using drugs conjugated to anti-ROBO4 antibodies.
The Bicknell group later improved a cDNA data mining method to screen for genes preferentially expressed in endothelium19,20. Both the assignment of expressed sequence tags (ESTs) and statistical analysis were improved to eliminate the false positive rate associated with the analysis conducted by Huminiecki and Bicknell16. These improvements allowed for the accurate prediction of 14 endothelial specific genes, such as the Rho-related GTP-binding protein (RhoJ) (a further 160 genes were also predicted to be significantly upregulated in endothelial cells).This approach was again combined with SAGEmap xProfiler, which allowed for the accurate prediction of 58 endothelial specific genes, such as the RhoJ (a further 459 genes were also predicted to be significantly upregulated in endothelial cells). Finally, bulk tumour cDNA libraries were subtracted from normal tissue cDNA libraries, which allowed for the prediction of 27 potentially tumour endothelial specific genes.
St. Croix et al.3 were able to identify a number of tumour endothelial specific markers by generating two SAGE libraries from purified normal colon endothelium and colorectal tumour associated endothelial cells. These two libraries contained a total of roughly 93,000 tags, of which 50,298 were unique and it was estimated that roughly 32,703 tags were generated for unique transcripts. These tags were compared to those of cell lines derived from colon tumours, which allowed for the identification of 93 transcripts that were at least 20 fold higher in the primary endothelial cells. Furthermore, by comparing the tags from the tumour endothelium to the normal endothelium, 46 tags were found to have a 10 fold greater expression in tumour endothelial cells. The top 25 most differentially expressed tags corresponded to 11 known genes, of which six genes were known markers of endothelium that were active in angiogenesis. The remaining 14 tags were from areas of the genome not characterised as being genes, nine of which were confirmed to be tumour endothelial specific using in situ hybridisation. Further research has been conducted on many of these novel TEMs, for example TEM8 (anthrax toxin receptor 1), which has been targeted using modified forms of the anthrax toxin21,22 and antibodies23 as potential anti-angiogenic therapies for the treatment of multiple tumour types.
The EST libraries are generally constructed with data from SAGE, CAGE (cap analysis of gene expression) and MPSS (massively parallel signature sequencing), which as described above can yield some interesting results. These tag-based sequencing techniques generate short tags that due to their length often cannot be uniquely mapped to the human reference genome and for the same reason cannot distinguish between splice variants. The generation of data from patient matched endothelium from normal and tumour tissue improves the chances of identifying tumour endothelial markers. However, these techniques are often based upon ‘Sanger sequencing’, which has a very low throughput and is expensive24-26. Therefore it is often not feasible to generate new EST data if the existing libraries won’t enable you to answer your hypothesis; conjointly if publically available EST data is available the libraries might have been sequenced to an insufficient depth.
Microarrays can provide a high throughput alternative to EST data acquisition and can more easily give a lot of information regarding the transcriptome of endothelial cells from many sources10,27. Ho et al.28 utilised custom microarrays to analyse 672 genes in cultured HUVEC, human coronary artery endothelial cells (HCAEC), human aortic endothelial cells (HAEC) and human lung microvasculature endothelial cells (HMVEC). Data was also generated from five different types of cultured cells of non-endothelial origin using the custom chip to determine which transcripts were expressed in cells other than the endothelium. Sixty four potential pan-endothelial markers were identified to be 3-55 fold more highly expressed in endothelial cells when the four endothelial cell datasets were compared to the five non-endothelial cell datasets, several of which had not previously been described. In addition to looking at the transcripts upregulated in all of the four endothelial cell types, Ho et al.28 also looked at the differences between the transcripts upregulated in each of the four endothelial cell types.
Despite this study using microarray technology that was fairly advanced for its time, it is limited due to the use of probes for only 672 of the 20,000 genes in the human genome. Three hundred and eighty four of these genes were chosen based upon a virtual subtraction of gene expression data and 288 genes from subtraction cloning from endothelial cells stimulated with TGF-β. Therefore, the probe selection would have been limited by the number of transcripts generated by the subtractive hybridisation and number / variety of tags in the SAGE libraries. However, even when using chips with a more comprehensive range of probes tiling the genome were used, it is not always possible to detect splice variants and previously unknown genes because of the targeted nature of microarray probes26. Furthermore, unlike the tag-based sequencing methods, microarrays provide expression data in terms of an analysis of continuous measures (fold change to a reference) and do not provide the raw count of the gene expression. This makes it very difficult to compare transcript expression levels across different experiments25. ‘Whole transcriptome shotgun sequencing’ on the other hand has the strengths of both techniques.
Whole transcriptome shotgun sequencing of the tumour endothelium
Whole transcriptome shotgun sequencing can also be known as next (or second/third) generation sequencing (NGS), deep sequencing and ‘RNAseq’. RNAseq is capable of collecting data regarding the whole transcriptome in high throughput manner and produces data that is formed of discrete counts (reads). The ability to view the fold change and view the read count is a significant advantage over microarrays because it is possible to easily determine which genes are both differentially expressed and highly expressed or absent in a dataset25,29. Furthermore, splice variants and novel expressed transcripts can be more easily identified and quantified, because unlike the EST approaches, multiple reads can be generated across the full length of a transcript26.
The basic distinction between the second and third generation sequencing platforms is that third generation sequencers do not require the sample to be amplified by PCR and detect the sequence in real time. Third generation sequencers capture the addition of a nucleotide to the complementary strand directly. Ion Torrent, Pacbio and Nanopore are examples of third generation sequencers. At the moment at least, it does not matter too much which commercially available sequencing technology is employed for the purpose of obtaining RNAseq data. Having said that, some sequencing technologies certainly lend themselves to the task of generating RNAseq data better than others generate. The reviews written by Liu et al.29, Branton et al.30, Mardis31 and Glen32 provide a good introduction to each individual next generation sequencing platform and their relative strengths and weaknesses, which in the main haven’t been described in this article.
The differential expression of two (or more) datasets is often what a biologist is most interested in, however the sequencing technology can affect the mapping, which in turn will affect the quantification of reads across a transcriptome. For the moment at least there seems to be a trade-off between the collection of data in the form of long reads or short reads at a higher depth. The higher sequencing depth allows for the characterisation of transcripts that are rarer or are less efficiently sequenced. However, a subset of the short reads obtained from NGS can be difficult to map (Figure 3). Furthermore, sequencers that give data in the form of short reads (fewer than 50 base pairs) can generate reads that can be wrongly mapped due to the presence of even one or two single nucleotide polymorphisms (SNPs) or sequencing errors.
Rapid advances within the NGS fields have made it possible to acquire a terabyte worth of data in a single run and easily accumulate terabytes of data. Illumina sequencing is arguably the most easily analysed at present. Nevertheless, individual researchers can often be ill prepared for meeting the challenge of transferring, storing, processing such massive datasets. Nor do they recognise the hidden costs of next generation sequencing once you have the data. Many researchers do not store their raw data; rather revert to keeping the data after base calling33. Indeed, many researchers do not have knowledge of how to conduct the bioinformatic analysis of their sequencing data and can sit on the unprocessed data for many years.
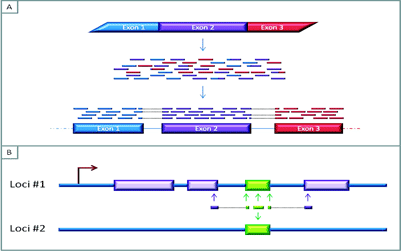
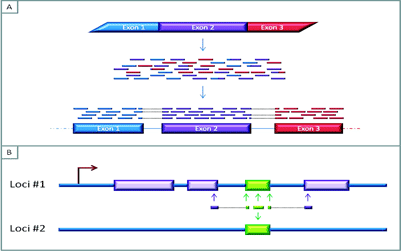
Figure 3A: The mapping of RNAseq reads to a reference genome. The short reads are generated by sequencing fragmented cDNA produced from messenger RNA (mRNA), the overlapping areas between these reads aid in the bioinformatic re-construction of the sequence of the expressed RNA. Furthermore, these overlaps can provide information about exon-exon boundaries and possibly allow for the characterisation of splicing variants
Figure 3B: Reads that map to multiple areas of the genome. Difficulties in assigning a read to the genome can emerge if a gene is expressed and contains regions that are not unique to that area of the genome. In this Figure, the green region represents a region that is repeated elsewhere in the genome. If the read contains nucleotides from unique neighbouring areas, it might be possible to assign this read to the genome. But if the read is fully within the non-unique/repeat region, it may not be possible to distinguish which part of the genome the read represents
Getting the most out of RNAseq
It is interesting to note that whilst NGS appears to have become the preferred method of genome / transcriptome characterisation, very little attention is paid to the fundamental aspects of the experimental design. Biologists have tended to not treat sequencing experiments as they would any other standard experiment and do not pay close attention to their controls and replicates34. Observational studies with no biological replication are common in RNAseq literature, previously this was acceptable because of the high cost of conducting RNAseq, providing further validation of targets is conducted. However, close attention should be paid to conducting well designed and statistically robust RNAseq experiments, especially now that the cost of sequencing has fallen so rapidly and is set to become even more affordable.
Biological replicates can be barcoded and pooled directly after RNA extraction and fragmentation. The pooling ensures that any bias that is introduced during the reverse transcription, PCR amplification and sequencing is the same across the biological replicates. The pooled samples can then be split across multiple lanes to gain the same sequencing depth as an experiment that processes the replicates independently and sequences in independent flow cells34.
RNAseq can be an extremely powerful tool and it can give vast amounts of information regarding every classification of RNA and the data can be (relatively) easily compared to NGS and EST data obtained from a variety of different cell types. This attribute lends itself nicely to the characterisation of transcripts up regulated in normal and tumour tissue associated endothelial cells. However, as the technology has advanced and entered more routine use, it appears that it is not realistic to conduct a differential next generation sequencing analysis and expect a high impact publication. The ability of RNAseq to directly answer a hypothesis is questionable; you can say that two datasets are different. But the biologically relevant (and interesting) question is to ask how the datasets are different, why and what is responsible. Indeed, different software packages for determining the differential expression of two transcripts can give wildly variable results, therefore some candidate confirmation is required if you wish to draw conclusions regarding a specific region. Future advances in sequencing technology will enable the identification of novel drug targets.
Acknowledgements
The authors would like to extend their thanks to the MRC for financial support and the ‘muses’ in the Bicknell group for the inspiration they have provided.
References
- Dougherty GJ, Chaplin DJ. Development of Vascular Disrupting Agents. In: Meyer T, editor. Vascular Disruptive Agents for the Treatment of Cancer. New York, USA: Springer Science; 2010. p. 1-30
- Burrows FJ, Thorpe PE. Eradication of large solid tumors in mice with an immunotoxin directed against tumor vasculature. Proceedings of the National Academy of Sciences. 1993;90:8996-9000
- St. Croix B, Rago C, Velculescu V, Traverso G, Romans KE, Montgomery E, et al. Genes Expressed in Human Tumor Endothelium. Science. 2000 August 18, 2000;289(5482):1197-202
- Neri D, Bicknell R. Tumour vascular targeting. Nature Reviews Cancer. 2005;5(6):436-46
- Heath VL, Bicknell R. Anticancer strategies involving the vasculature. Nature Reviews Clinical Oncology. 2009;6(7):395-404
- Fukumura D, Xu L, Chen Y, Gohongi T, Seed B, Jain RK. Hypoxia and Acidosis Independently Up-Regulate Vascular Endothelial Growth Factor Transcription in Brain Tumors in Vivo. Cancer Research. 2001;61(6020-6024)
- Dachs GU, Chaplin DJ. Microenvironmental control of gene expression: Implications for tumor angiogenesis, progression, and metastasis. Seminars in Radiation Oncology. 1998;8(3):208-16
- Helmlinger G, Yuan F, Dellian M, Jain RK. Interstitial pH and pO2 gradients in solid tumors in vivo: High-resolution measurements reveal a lack of correlation. Nat Med. [10.1038/nm0297-177]. 1997;3(2):177-82
- Ando J, Yamamoto K. Vascular Mechanobiology – Endothelial Cell Responses to Fluid Shear Stress. Circulation Journal. 2009;73:1983-92
- Zhang H-T, Gorn M, Smith K, Graham AP, Lau KKW, Bicknell R. Transcriptional profiling of tuman microvascular endothelial cells in the proliferative and quiescent state using cDNA arrays. Angiogenesis. 1999;3(3):211-9
- Ahmed Z, Bicknell R. Angiogenesis Protocols. 2nd ed. Martin S, Murray C, editors. New York, USA: Humana Press; 2009
- Arroyo AG, Iruela-Arispe ML. Extracellular matrix, inflammation, and the angiogenic response. Cardiovascular Research. 2010;86(2):226-35
- Ferrara N, Kerbel RS. Angiogenesis as a therapeutic target. Nature. 2005;438:967-74
- Risau W. Mechanisms of Angiogenesis. Nature. 1997;671:386-90
- Adams RH, Eichmann A. Axon Guidance Molecules in Vascular Patterning. Cold Spring Harbor Perspectives in Biology. 2010;2(5):a001875-a
- Huminiecki L, Bicknell R. In Silico Cloning of Novel Endothelial-Specific Genes. Genome Research. 2000;10(11):1796-806
- Huminiecki L, Gorn M, Suchting S, Poulsom R, Bicknell R. Magic Roundabout Is a New Member of the Roundabout Receptor Family That Is Endothelial Specific and Expressed at Sites of Active Angiogenesis. Genomics. 2002;79(4):547-52
- Yoshikawa M, Mukai Y, Okada Y, Tsumori Y, Tsunoda S-i, Tsutsumi Y, et al. Robo4 is an effective tumor endothelial marker for antibody-drug conjugates based on the rapid isolation of the anti-Robo4 cell-internalizing antibody. Blood. 2013
- Herbert JMJ, Stekel D, Sanderson S, Heath VL, Bicknell R. A novel method of differential gene expression analysis using multiple cDNA libraries applied to the identification of tumour endothelial genes. BMC Genomics. 2008;9(1):153
- Herbert JJ, Stekel D, Mura M, Sychev M, Bicknell R. Bioinformatic Methods for Finding Differentially Expressed Genes in cDNA Libraries, Applied to the Identification of Tumour Vascular Targets. In: Lu C, Browse J, Wallis JG, editors. cDNA Libraries: Humana Press; 2011. p. 99-119
- Phillips DD, Fattah RJ, Crown D, Zhang Y, Liu S, Moayeri M, et al. Engineering Anthrax Toxin Variants That Exclusively Form Octamers, and Their Application to Targeting Tumors. Journal of Biological Chemistry. 2013 February 7, 2013.
- Cryan LM, Rogers MS. Targeting the anthrax receptors, TEM-8 and CMG-2, for anti-angiogenic therapy. Frontiers in Bioscience. 2011;16:1574-88
- Chaudhary A, Hilton Mary B, Seaman S, Haines Diana C, Stevenson S, Lemotte Peter K, et al. TEM8/ANTXR1 Blockade Inhibits Pathological Angiogenesis and Potentiates Tumoricidal Responses against Multiple Cancer Types. Cancer Cell. 2012;21(2):212-26
- Simpson JC, Wellreuther R, Poustka A, Pepperkok R, Wiemann S. Systematic subcellular localization of novel proteins identified by large-scale cDNA sequencing. EMBO Reports. 2000;1(3):287-92
- Wang Z, Mark G, Michael S. RNA-Seq: a revolutionary tool for transcriptomics. Nature Reviews Genetics. 2009;10:57-64
- Mortazavi A, Williams BA, McCue K, Schaeffer L, Wold B. Mapping and quantifying mammalian transcriptomes by RNA-Seq. Nature Methods. 2008;5(7):621-8
- Ghilardi C, Chiorino G, Dossi R, Nagy Z, Giavazzi R, Bani M. Identification of novel vascular markers through gene expression profiling of tumor-derived endothelium. BMC Genomics. 2008;9(1):201
- Ho M, Yang E, Matcuk G, Deng D, Sampas N, Tsalenko A, et al. Identification of endothelial cell genes by combined database mining and microarray analysis. Physiological Genomics. 2003;13:249-62
- Liu L, Li Y, Li S, Hu N, He Y, Pong R, et al. Comparison of Next-Generation Sequencing Systems. Journal of Biomedicine and Biotechnology. 2012;2012:1-11
- Branton D, Deamer DW, Marziali A, Bayley H, Benner SA, Butler T, et al. The potential and challenges of nanopore sequencing. Nature Biotechnology. 2008;26(10):1146-53
- Mardis ER. Next-Generation DNA Sequencing Methods. Annual Review of Genomics and Human Genetics. 2008;9(1):387-402
- Glenn TC. Field guide to next-generation DNA sequencers. Molecular Ecology Resources. 2011;11(5):759-69
- Nature Publishing Group. Byte-ing off more than you can chew. Nature Methods. 2008;5(7):577
- Auer PL, Doerge RW. Statistical Design and Analysis of RNA Sequencing Data. Genetics. 2010;185(2):405-16
Biographies
Klarke M. Sample has a fervent interest in human genetics, which led him to achieve a Masters in Medical Genetics from the University of Glasgow in 2009. He is currently utilising NGS technology to probe the endothelial cell transcriptome as part of his doctoral degree at the University of Birmingham.
Roy Bicknell carried out his undergraduate and doctoral studies at Oxford University and his postdoctoral training at Harvard Medical School. He was formerly Professor of Cancer Cell Biology at Oxford and is currently Professor of Cancer Biology and Genomics at the University of Birmingham. He has published over 200 peer reviewed articles in the fields of endothelium and angiogenesis and a particular interest is the difference between tumours compared to healthy tissue endothelium.