AI-supported spectroscopy delivers superior pharmaceutical packaging QC
Posted: 29 September 2023 | Catherine Eckford (European Pharmaceutical Review) | No comments yet
A combination of mid-infrared spectroscopy and artificial intelligence (AI) achieved excellent prediction capability for inline pharmaceutical packaging content verification, a study has shown.
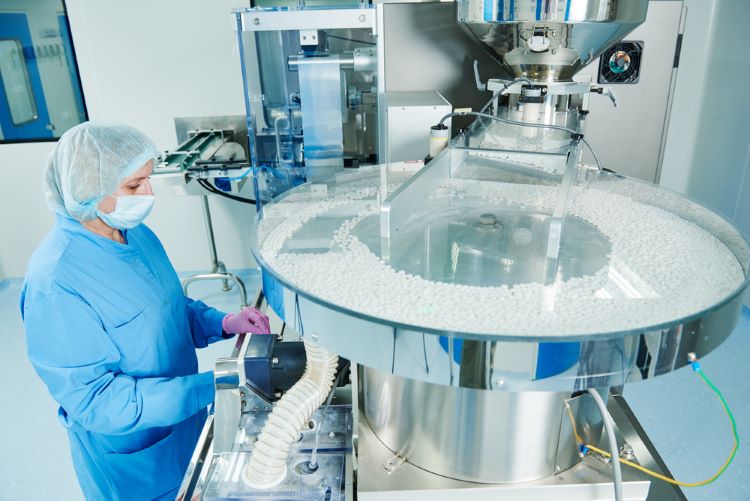
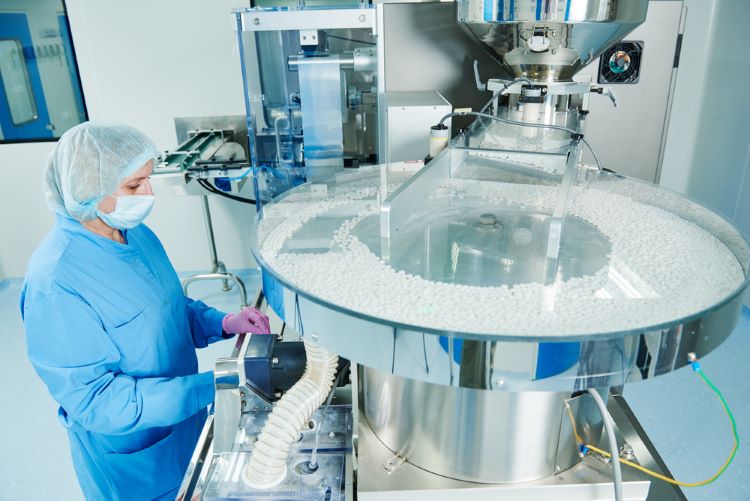
A paper published in the IEEE Sensors Journal has demonstrated 100 percent inline pharmaceutical packaging content verification by combining standard diffuse reflectance MOEMS-EC-QCL spectroscopy with artificial intelligence (AI).
It described how a quantum cascade laser (QCL)-based blister-verification sensor with verification via backscattering mid-infrared (IR) spectroscopy substance chemical identification can support blistering machines to achieve a better inline content verification.
Automatic detection and classification of a number of pills was achieved in the study. Ultimately, the innovative approach offers fast, non-invasive, contactless, and highly selective classification of pharmaceutical pills. The system can function uninterrupted and operator free, the authors stated.
Current quality control challenges in pharmaceutical packaging
Flores et al. explained that for quality control (QC) of medicine blister packaging, currently, visual inspection verifies number, colour, and shape of each individual blister package. At present, regulations imply in practise that an experienced pharmacist is required for final verification.
Therefore, with a need for inline content verification for this type of pharmaceutical packaging, sensors must provide rapid, non-contact, and accurate chemical information of each individual blister content. However, for solid doses with similar physical appearance, those visual quality control measures fail. No chemical verification is done by default, due to time constraints and a limit in the number of qualified personnel available, the authors wrote.
Study method
In the study, the sensor concept combined machine vision and machine learning with laser-based diffuse reflectance spectroscopy to verify the blister content. It was found that principal component analysis (PCA)-fed machine learning was the most accurate and resilient classifier approach.
According to the authors, 100 percent identification accuracy was demonstrated for 13 different medication types using machine learning classification. This level of accuracy also achieved no reduction in PCA-dimensionality.
In comparison, only 97.4 percent identification accuracy is achieved by standard cross correlation to fourier transform infrared (FTIR) data. The authors summarised that the machine-learning classifier offered greater performance compared to the standard FTIR-supported classifier.
Flores et al. concluded that specific advantages of this approach, such as superior accuracy, measurement time and the selectivity makes it applicable for quality control in pharmaceutical packaging lines.