Machine learning can predict outcomes for inkjet printed pharmaceuticals
Posted: 23 May 2023 | Catherine Eckford (European Pharmaceutical Review) | No comments yet
A paper has shown how machine learning (ML) models predicted the inkjet printing printability of drug formulations with high accuracy.
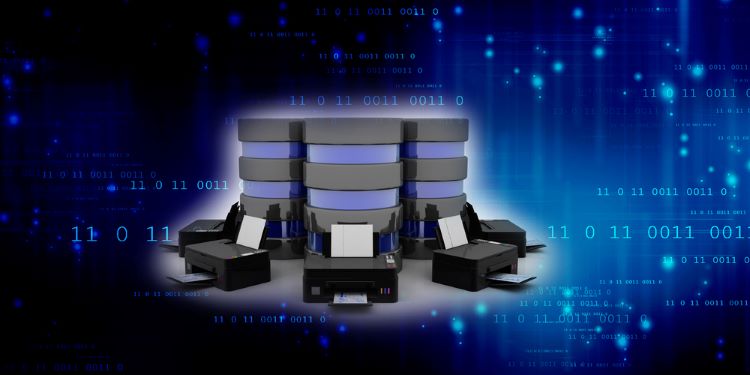
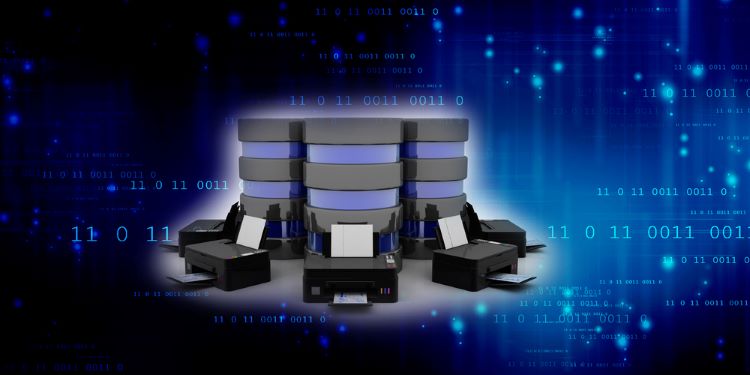
Using inkjet printing, optimised machine learning (ML) models predicted the printability of drug formulations with an accuracy of 97.22 percent, a paper published in International Journal of Pharmaceutics: X has shown.
Inkjet printing makes formulation and printing parameter optimisation time-consuming. This is especially true for additive manufacturing and the production of unique dosage forms and personalised medicines. Benefits of the latter include low cost and versatility. For example, piezoelectric inkjet printing is one printing method used for personalised medicines.
ML has been used to predict printing outcomes and dissolution behaviours of fused deposition modelling (FDM™) -printed dosage forms and digital light processing (DLP)-printed tablets, the paper noted.
The study evaluated how ML can analyse nuance differences and provide more reliable predictions compared to the conventional guidance on jettability based on Z values, which is a printhead setting.
There is potential for a predictive model for inkjet printing outcomes to be developed, the researchers theorised. Therefore, the study aimed to develop and evaluate the performance of ML models for predicting inkjet printing printability and the total drug dose in the final printed dosage form.
The ML models used in the study for predicting printability were:
- Random forest
- Multilayer perceptron
- Support vector machine.
How well did the optimised machine learning models predict printability?
In addition to predicting the printability of formulations with a high accuracy, the optimised ML models also predicted the quality of the prints with an accuracy of 97.14 percent. Current guidance states that only inks with Z values <10 are printable. As a comparison, following this guidance produces an accuracy of 64.39 percent.
Benefits of machine learning for predicting printability and current guidelines
Formulation development and optimisation is a time- and resource-intensive process that can be considerably accelerated by guidance from predictive in silico tools.
The researchers stated that a predictive tool to better determine the printability of inks prior to the actual preparation and testing would allow pharmaceutical researchers to focus to devising more unique dosage forms to solve unmet clinical challenges.
Analysis of the dataset comprising of 687 formulations revealed that positive printing outcomes were overwhelmingly published in favour of negative outcomes. Despite the imbalanced dataset, the optimised ML model for predicting printability performed significantly better than the conventional guidance.
Applications of inkjet printing in drug formulation
For drug formulation, inkjet printing has been used to load drugs onto orodispersible films, bioadhesive films for cervical administration and transdermal microneedles, noted the authors. Inkjet printing has also been used to dispense drug-loaded micro-and nanoparticles dispersed in the ink liquid.
Inkjet printing may also be combined with other additive manufacturing technologies otherwise unattainable with conventional manufacturing technologies.
For instance, one study highlighted in the paper used inkjet printing in conjunction with FDM™ 3D printing to produce drug-loaded tablets with quick response (QR) codes printed on them. These QR codes were designed to encode patient-related information that could be read using a smartphone, and to serve as an anti-counterfeit strategy.
Another study also applied the fabrication of orodispersible substrates and capsules with printed QR codes. In another case study featured in the paper, inkjet printing has been used to fabricate the entire 3D drug-loaded tablets.
Carou-Senra et al. concluded that the study demonstrates that machine learning models “can feasibly provide predictive insights to inkjet printing outcomes prior to formulation preparation, affording resource- and time-savings.”
Related topics
Big Data, Dosage, Drug Development, Drug Manufacturing, Formulation, Models, Personalised medicine, Research & Development (R&D), Technology, Therapeutics