Evolving real-time dissolution prediction and product release
Posted: 7 September 2022 | Hannah Balfour (European Pharmaceutical Review) | No comments yet
EPR highlights the development of a model to predict dissolution of sustained release formulations based on Raman process analytical technology (PAT) sensor data that could enable real-time release testing.
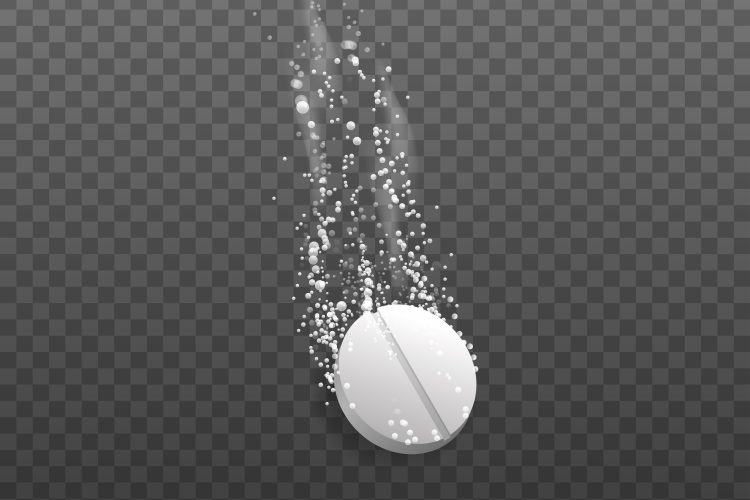
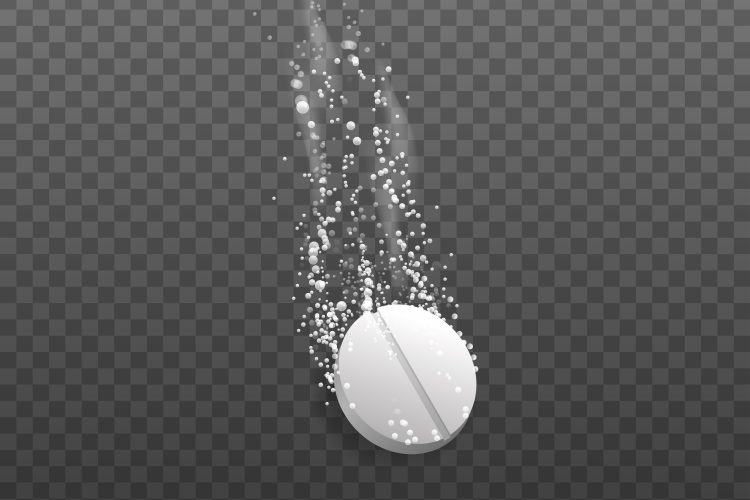
Researchers have developed a deterministic permeation model (DPM) that predicts the in vitro release profile of an active pharmaceutical ingredient (API) embedded in hydroxypropyl-methylcellulose (HPMC) matrix tablets. The model, based on Raman spectra, has the potential to process in real-time data yielded by process analytical technology (PAT) sensors, which could allow it to be utilised for real-time release testing (RTRt).
Unlike with immediate release systems, the release of drugs from sustained release solid dosage formulations (such as HPMC matrix tablets) is extremely complex, involving many steps and changing rates of diffusion. Dissolution is critical to the bioavailability of a pharmaceutical product – a critical quality attribute – and so is closely monitored using in vitro methods and modelling. Raman chemical imaging has been used to elucidate the structure of formulations and how these change during drug release.
As the industry works to implement continuous processes, leveraging PAT to provide real-time information for decision making and process control, real-time product release is becoming an increasingly desirable proposition. This requires RTRt to be developed.
Two methods can be used to predict dissolution in pharmaceuticals: first principles-based methods are typically used in formulation development to study how formulation parameters relate to dissolution, while data-driven empirical approaches like Partial Least Squares (PLS) regression are used in the later stages with models for RTRt built on these empirical methods.
According to a paper published in the International Journal of Pharmaceutics, so far, a first-principles method has not been used to process PAT sensor data for real-time dissolution prediction. In their work, Horkovics-Kovats et al. created a mechanistic approach-based model and used it to predict published dissolution profiles based on Raman PAT data. To determine its applicability, the dissolution predictions from the mechanistic model were compared to those of a typical empirical-based model called an artificial neural network (ANN).
Tablets containing various amounts of the highly soluble API drotaverine hydrochloride and HPMC were produced using different compression pressures according to a 33 full factorial design. Their Raman spectra were recorded before dissolution testing, and this was used to develop the DPM (mechanistic model).
In the study, the researchers showed that the developed mechanistic model “is capable of accurately predicting the dissolution profile of extended-release tablets manufactured with various settings” by comparing the predicted dissolutions with measured values. Thus they deemed it “the first utilisation of such a model… to predict dissolution profiles based on data provided by PAT sensors”.
Moreover, when the dissolution predictions of the DPM were compared to the outputs of a typical empirical model (an artificial neural network [ANN]), they were found to perform similarly, with the mechanistic model having two notable benefits: being based on physical phenomena and needing half the number of samples to train the model.
The authors concluded that, since the DPM could allow the rapid prediction of dissolution profiles, their method has the potential to be used for the real-time processing of PAT data and therefore could be leveraged for RTRt.