Phase I oncology studies: rule- versus model-based design
Posted: 8 February 2022 | Karen Ooms (Quanticate) | No comments yet
In this article, Karen Ooms, Head of Statistics at Quanticate, compares the rule- and model-based approaches to Phase I trial design, exploring their benefits and weaknesses in defining maximum tolerated dose for oncology products.
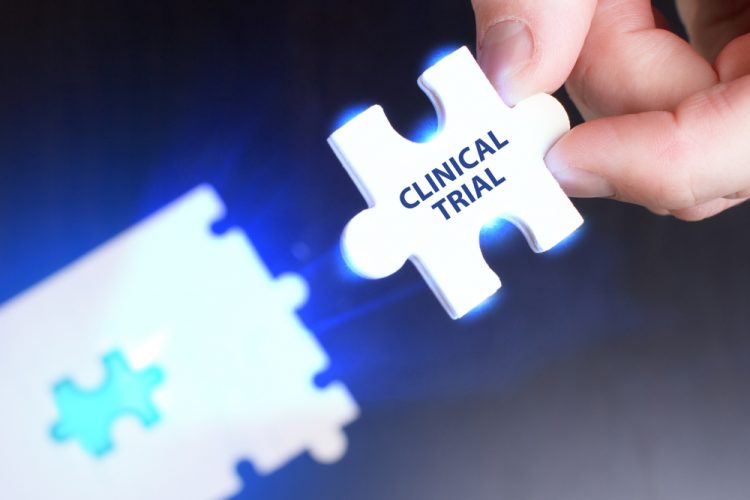
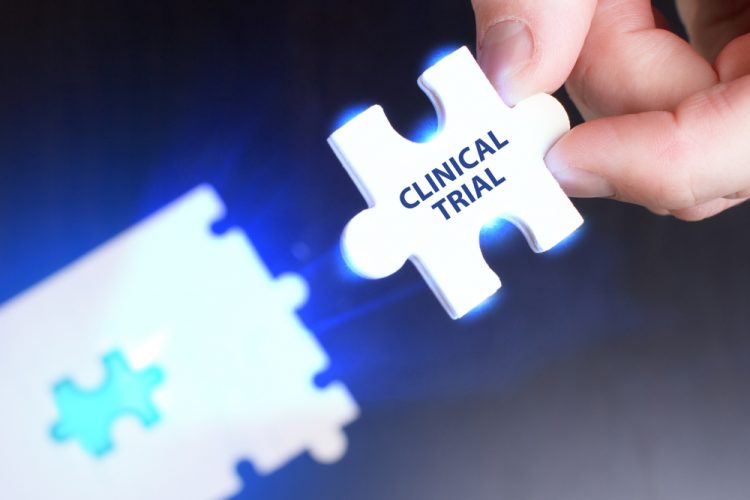
Optimising Phase I oncology study design
Reliable, efficient Phase I dose-finding trials are essential in drug development as they establish a recommended dose for later-phase testing and enable faster, cheaper drug development.
As cancer treatments often have many, and potentially, severe side-effects, the primary objective of a Phase I study in oncology clinical development is to establish the ‘highest’ dose that can be given to a patient that does not result in ‘too many’ toxic effects. This is referred to as the maximum tolerated dose (MTD). The decision on how to define a suitable MTD should be part of the clinical considerations taken in the design phase of the study; what is an acceptable rate of dose-limiting toxicity (DLTs) compared to the severity of the disease in the patient population being studied.
The first phase in clinical trials for these drugs is conducted in patients with the disease rather than healthy volunteers, as it is deemed unethical to expose them to the side effects.
An important but often overlooked part of study design at this phase includes defining what constitutes ‘too many’ toxic effects. To help clarify this, the concept of a DLT has been introduced.
The decision on how to define a suitable MTD should be taken in the design phase and include a definition of the acceptable rate of DLTs compared to the severity of the disease in the study’s patient population. This will then inform the Recommended Phase II Dose (RP2D).
Two approaches are invariably used in these studies: rule- and model-based, with the former being the more widely used by a notable margin. Below, I weigh the comparative benefits and drawbacks of each when it comes to oncology trial design.
Acceptable rate of DLTs
As cancer patients are often very ill, or likely to become very ill without treatment, it is often deemed acceptable that a patient can suffer a DLT when they are receiving their cancer treatment. For many drugs and indications, the targeted DLT rate (ie, the maximum acceptable probability of experiencing a DLT per patient) is usually set within the range of 0.16 to 0.33, although this may be more.
a well-planned design to determine the MTD should minimise the number of patients subjected to both inefficacious doses and excessive levels of study drug”
Therefore, in this scenario, the MTD would be the highest dose where the chance of a patient having a DLT is at most the targeted dose limiting toxicity rate.
To determine the MTD, a Phase I oncology study will usually consist of several cohorts of patients, with the first cohort being given a low starting dose of the treatment and subsequent cohorts being given varying doses according to an escalation plan derived from the patients’ responses during the trial.
An important principle to remember is that a well-planned design to determine the MTD should minimise the number of patients subjected to both inefficacious doses and excessive levels of study drug where the risk of a DLT becomes unacceptable.
Rule-based designs
One of the most used rule-based design is called the 3+3 algorithm; other rule-based designs have been developed that are adaptions of it.
The design is simple – the study begins at a low dose and escalates after every three to six patients per dose; the recommended dose is defined as the largest dose with fewer than two patients experiencing a predefined DLT during the first course of treatment.
Model-based designs
A model-based design assumes a relationship between the dose of the drug given to the patient and the likelihood of the patient having a DLT; usually that there is a higher chance of having a DLT at higher doses.
Mathematically, there are many ways of representing a dose-toxicity relationship. By including a prior distribution, rate of a DLT at a given dose can be modelled using Bayesian methods. This allows maximal use of the data collected. It is more complex than estimating the MTD using the rule-based approach, requiring input from statisticians with a good grasp of working with Bayesian methodologies.
A comparison
With rule-based approaches there a simple linear process to dose escalation decision-making. Trial data is collected, increases to the dose levels are made according to the 3+3 (or another adaptation) algorithm and a clinician reviews medical history, trial data and recommended dose, then makes dose escalation decisions. The number of points where a clinician can influence decision is comparatively low – and potentially restrictive.
The model-based approach is arguably more circular and consultative. By assuming an underlying dose-response model, all data comes into play at every decision point, provided that the assumed model is roughly correct. Historical data is included as prior information, and model-based dose-toxicity relationship data is considered. The clinician’s expertise is used to include relevant prior information to improve the estimates from the models and to rationalise estimated rates before they review medical history, trial data and recommended dose and make any dose escalation decision.
Model-based
Model-based designs are more likely to recommend the correct MTD and dose more patients appropriately according to the US Food and Drug Administration (FDA) guidance for therapeutic cancer vaccine trials. The guidance goes as far as to discount 3 + 3 designs as they are not likely to identify the true MTD, instead they recommend model-based approaches such as the continuous reassessment method (CRM).1
The Adaptive Designs Working Group of the MRC Network of Hubs for Trials Methodology Research have expressed a very clear preference for model-based designs.2 The Group argues that a model-based approach offers the following benefits:
- Target DLT is more clearly defined and can be flexibly chosen
- Relatively more patients are treated at the optimal dose and fewer patients are treated at sub-therapeutic doses
- More efficient utilisation of available data
- More simplified extension of the process to address more complex questions
- Simpler to accommodate deviations from the original plan (eg, other doses or a different numbers of patients on a dose).
Additionally, simulations have demonstrated that a median of three to four fewer patients are required in trials that use a model-based design as compared to the 3+3 approach3 and further analysis found a median time gain of 10 months.4
With new software, statistical expertise and advocacy from within the industry, it is likely that model-based approaches will be more widely adopted in the coming years”
However, it is more complex than estimating the MTD using the rule-based approach because it requires input from statisticians with a good grasp of working with Bayesian methodologies.
Some model-based designs are highly resource intensive, as they demand a complete reanalysis of data and the dosing scheme with each new patient. This creates a need to decide how to proceed at every interim analysis, which can easily consume more resource time than many institutions can allocate. Rule-based designs automate these decisions which explains their continued popularity.
Rule-based
Despite the potential advantages of a model-based approach in phase I dose escalation studies, its use remains low. A recent review showed that only 5.4 percent trials use model-based designs.5
Several reasons are given for this, including:
- A lack of expertise in the clinical and statistical communities
- A lack of knowledge of available software packages
- Chief investigators’ preference for algorithm-based designs (eg, 3+3)
- High complexity with no assurance that the benefits justify the more resource and time intensive process.
Further work is being done to improve rule-based designs such as augmenting the process with more robust steps. The cohort-sequence design,6 for example, begins with a small cohort size and escalating through the planned doses, increasing the cohort size when toxicities are encountered. The decision to escalate, add more participants at the current dose, or de-escalate is informed by whether the observed DLT count in the cohort falls below, equals or exceeds the corresponding critical value.
The design allows efficient identification of a target toxicity rate without unnecessarily enrolling patients at low doses – and potentially includes a built-in dose-expansion cohort.
Conclusion
With the emergence of several novel, innovative, complex, often expensive technologies in the oncology therapeutic area, ensuring that trials are appropriately designed to reach the MTD quickly whilst maintaining patient safety is paramount.
Traditionally, Phase I trial designs have been rule-based, but there is an emerging trend towards model-based designs. Simulations show that model-based designs perform better, faster and are safer to establish the RP2D. The results of a literature review and survey reported in the British Journal for Cancer found that, “many pharmaceutical companies have overcome practical barriers to implementing model-based designs, motivated by inaccurate doses from 3+3 trials causing failed phase II and III studies.”7 However, their purported superiority is yet to be confirmed with practical trial performance. In contrast, the familiarity and accessibility of rule-based approaches maintains their appeal.
With new software, statistical expertise and advocacy from within the industry, it is likely that model-based approaches will be more widely adopted in the coming years as they become less resource intensive to implement and the snowball effect of familiarity takes hold.
About the author
Karen Ooms, Executive Vice President, Head of Statistics, Quanticate
Karen is responsible for overseeing the Statistics department at Quanticate. She is a Chartered Fellow of the Royal Statistical Society and has a background in biostatistics spanning over 25 years. Prior to joining Quanticate in 1999 (Statwood), Karen was a Senior Statistician at Unilever.
References
- Guidance for Industry – Clinical Considerations for Therapeutic Cancer Vaccines [Internet]. US Food and Drug Administration; 2011 [cited 2 May 2019]. Available from: https://www.fda.gov/media/82312…
- A quick guide why not to use A+B designs Adaptive Designs [Internet]. Adaptive Designs Working Group (ADWG) of the MRC Network of Hubs for Trials Methodology Research; 2022 [cited 16 April 2019]. Available from: http://methodologyhubs.mrc.ac.uk/files/6814/6253/2385…
- Onar A, Kocak M, Boyett JM. Continual reassessment method vs. traditional empirically based design: modifications motivated by phase I trials in pediatric oncology by the Pediatric Brain Tumor Consortium. J Biopharm Stat. 2009:19(3): 437–455.
- van Brummelen E, Huitema A, van Werkhoven E, Beijnen J, Schellens J. The performance of model-based versus rule-based phase I clinical trials in oncology. Journal of Pharmacokinetics and Pharmacodynamics. 2016;43(3):235-242.
- Chiuzan C, Shtaynberger J, Manji GA, et al. Dose-finding designs for trials of molecularly targeted agents and immunotherapies. J Biopharm Stat. 2017;27:477–94
- Li S, Xie X, Heitjan D. Flexible, rule-based dose escalation: The cohort-sequence design. Contemporary Clinical Trials Communications. 2020;17:100541.
- Love S, Brown S, Weir C, et al. Embracing model-based designs for dose-finding trials. British Journal of Cancer. 2017;117(3):332-339.
Related topics
Anti-Cancer Therapeutics, Clinical Development, Clinical Trials, Drug Development, Drug Safety, Industry Insight, Research & Development (R&D)
Related organisations
British Journal for Cancer, US Food and Drug Administration (FDA)