Machine learning boosts spirulina bioproduction by up to 100 percent
Posted: 17 August 2021 | Hannah Balfour (European Pharmaceutical Review) | No comments yet
Collaborating with Google, Lumen Bioscience applied Bayesian black-box optimisation, a machine learning approach, to increase spirulina biomanufacturing productivity.
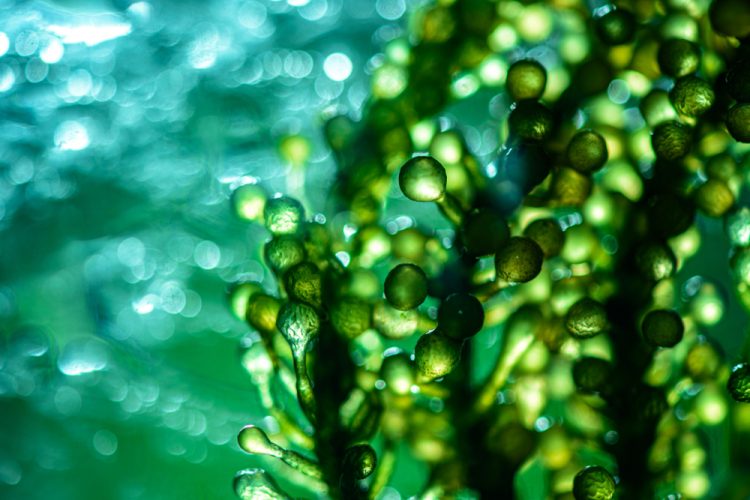
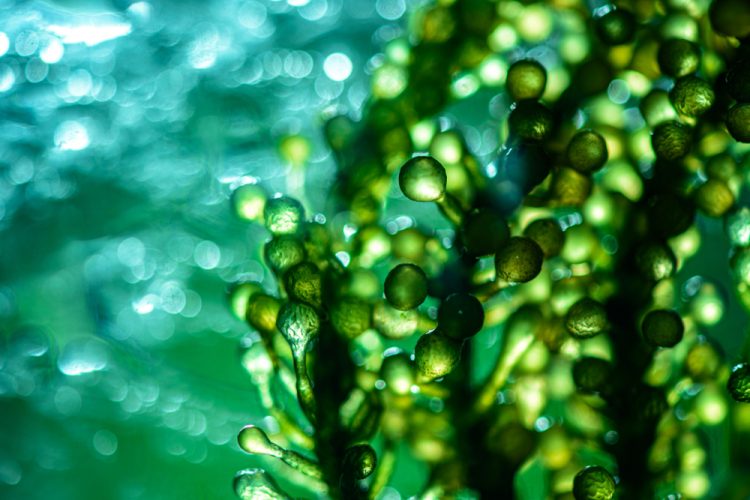
A new paper shows that applying machine learning (ML) to bioproduction can significantly increase recombinant protein production and thus advance the scalability of spirulina-based biologic drugs.
Under a research collaboration funded in part by the Bill & Melinda Gates Foundation, Lumen Bioscience worked with Google Accelerated Science to apply ML to increase the productivity of Arthrospira platensis (spirulina) using Bayesian black-box optimisation. The results of the collaboration, which are pending peer-review, have been published in pre-print on the bioRxiv server.
The paper describes how the ML approach of Bayesian black-box optimisation was used to guide experiments in 96 photobioreactors, exploring the relationship between production outcomes and 17 environmental variables, including pH, temperature and light intensity. The investigators reported that, after 16 iterative rounds of experiments, they identified key variable adjustments that doubled the production of heterologous proteins in spirulina, improving volumetric productivity by 70-100 percent.
The company explained that using ML for process optimisation was key because, even in a simple biomanufacturing system like one using spirulina, the number of potentially interacting variables is too vast to efficiently explore with traditional one-factor-at-a-time experimentation. Adding that using Bayesian black-box optimisation enabled them to short-cut the productivity improvement process that took decades for older biomanufacturing platforms such as yeast, Escherichia coli and Chinese hamster ovary (CHO).
According to Lumen, spirulina-based biomanufacturing is easily affordable for the developing world, but the productivity increases enabled by ML should facilitate the broader distribution of biomanufactured products in the developing world, a major priority of the Gates Foundation.
“The combination of two pioneering innovations – the machine-learning of Google and our spirulina-based therapeutics production – brings us even closer to a fully optimised approach that could have a major impact on devastating diseases globally,” commented Dr Jim Roberts, co-founder and Chief Scientific Officer of Lumen Bioscience. “We believe this paper is the first to describe the application of [artificial intelligence] AI techniques to biologics manufacturing. We look forward to the future implementation of these practices, as supported with funding from the Department of Energy, to provide mucosally and topically delivered biologics for highly prevalent diseases that, until now, have been infeasible due to the cost and scaling challenges of traditional biomanufacturing platforms.”
Lumen has recently received $2 million in extra funding from the US Department of Energy for ACCESS CARBON – a project to further improve the productivity of spirulina-based biomanufacturing. This project will build on the work described in the paper by expanding the number of variables evaluated to also include improvements from alternative, genetically diverse production strains and other key variables, and significantly increase complexity and scale.
“Lumen Bioscience’s spirulina-based biopharmaceutical manufacturing platform represented a unique and meaningful challenge for our ML team,” said Drew Bryant, leader of the research project at Google Accelerated Science. “Applying ML techniques to the challenge, we were able to significantly improve outcomes at a speed and cost that would not be possible using traditional methods. Further enhancements to this platform hold promise to revolutionise ideas about feasible disease targets and global access to spirulina-based biologic drugs.”
Lumen currently has three clinical stage investigational biologic drugs, all produced in spirulina: LMN-201 for Clostridium difficile infection; LMN-101 for traveller’s diarrhoea; and LMN-301 for COVID-19 gastrointestinal (GI) infection.
Related topics
Artificial Intelligence, Biologics, Bioproduction, Drug Manufacturing, Research & Development (R&D), Technology, Therapeutics