Mass cytometry could reveal best cancer drug regimens for clinical trials
Posted: 24 March 2020 | Rachael Harper (European Pharmaceutical Review) | No comments yet
Researchers have developed a new technology that could provide real-time analysis of patient samples during clinical trials to reveal the best cancer treatment regimens.
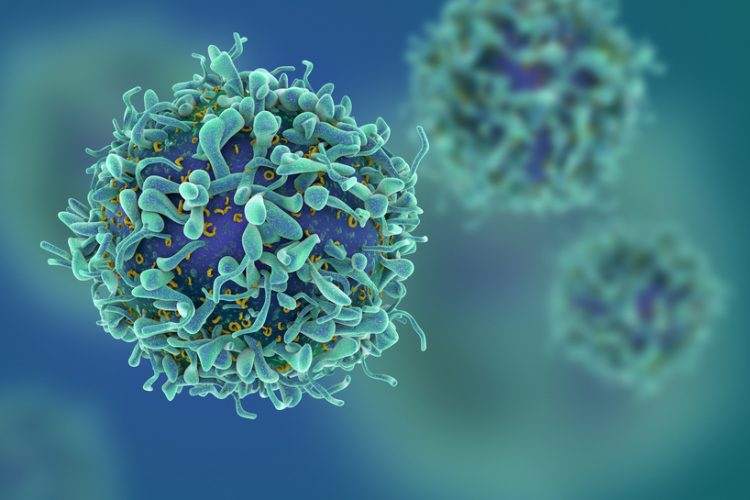
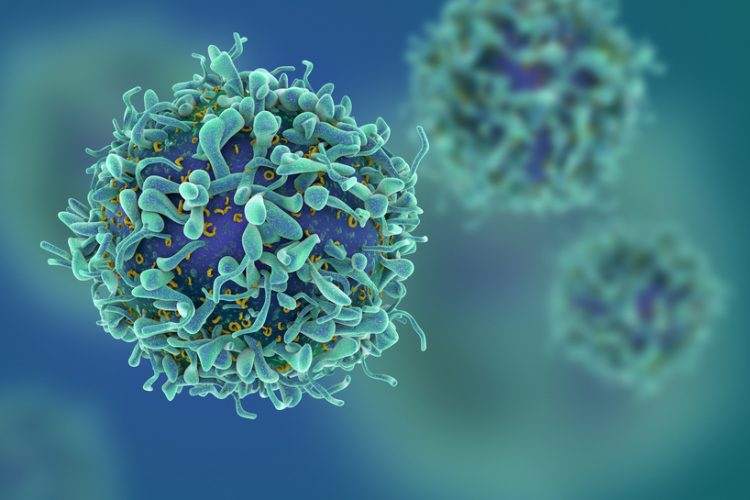
The inner workings of individual cancer cells have been revealed with new technology and could potentially identify more effective treatment combinations for people with cancer.
A joint Walter and Eliza Hall Institute, Australia, and Stanford University, US, team used a technique called mass cytometry (CyTOF) to simultaneously analyse the levels of more than 20 different proteins in millions of individual blood cancer cells. This revealed how these cells responded to different anti-cancer medicines, even suggesting potential new treatment combinations, they said.
The research team has said they hope that the new technique could be integrated into clinical trials both to understand why some patients are resistant to anti-cancer therapies and to predict suitable ‘biomarkers’ for matching patients with the most effective therapies for their disease.
The study was led by Walter and Eliza Hall Institute researchers Dr Charis Teh and Associate Professor Daniel Gray, in collaboration with Professor Garry Nolan and Dr Melissa Ko from Stanford University.
“We wanted to better understand the molecular differences between individual cancer cells so we could discover how these differences impact the cancer’s response to therapies – for example, whether some cells are more resistant than others to an anti-cancer drug,” Dr Teh said. “We decided that a new technology, called mass cytometry, would be an ideal approach to address this question.”
Mass cytometry can simultaneously measure the quantity of different proteins in a single cell.
“The system we developed simultaneously and precisely measures 26 separate proteins in a blood cancer cell line derived from myeloma – an incurable cancer of immune B cells,” Dr Teh said. “We focused on understanding why some cells are sensitive to anti-cancer agents, while others are resistant.
“We used machine learning to analyse the mass cytometry results of thousands of cells and were able to distinguish which cells survived treatment with standard medicines used to treat myeloma – and see how they differed from cells that were sensitive to these medicines,” she said.
Mass cytometry may even have a role in providing real-time detailed analysis of patient samples from clinical trials, Associate Professor Gray said.
“The panel of markers developed in this study gives researchers considerable scope to understand how cancer cells are responding to anti-cancer therapies – and as we found, it can even help to identify better drug combinations,” he continued.
“Adding mass cytometry to the analysis of clinical studies could reveal why some patients respond to therapies differently from others and how resistance to anti-cancer medicines can develop in a small fraction of cancer cells. Mass cytometry could also identify a small number of proteins that can be used as specific ‘biomarkers’ that can predict a patient’s response to therapy and be used to match that patient with the most effective treatments.”
The research was published in Cell Death & Differentiation.
Related topics
Analytical techniques, Anti-Cancer Therapeutics, Clinical Development, Clinical Trials, Drug Development, Research & Development (R&D)
Related organisations
Related people
Associate Professor Daniel Gray, Dr Charis Teh, Dr Melissa Ko, Professor Garry Nolan