Applying statistical inference in genomics with evidence based pathways: Towards elucidating new functional correlations of biomarkers
Posted: 22 February 2010 |
In conventional pharmacogenomic studies, genetic polymorphisms (including single nucleotide and copy number variations) are elucidated from case-control distribution of individuals usually representing ethnicity, severity of disease, and positive or negative response to treatment. However, the interpretation of a single genetic marker in this context is complicated, as the same marker may lead to multiple different phenotypes. Likewise, similar phenotypes can arise under different genetic backgrounds (models). This problem has led to the emergence of integrative approaches for combining statistical inference methods with bioinformatics-based text-mining, sequencing and expression analyses. An increasingly popular approach based on network analysis is challenged by the fact that a certain amount of the interpretation of the data is left to the subjective choices of the user. More rigorous statistical methods can be applied. However, these methods are also challenged by the fact that the developed measures of significance are provided outside the context of biology. In this perspective, we outline statistical methods and the development of evidence-based pathway analysis for identifying new biomarker correlations.
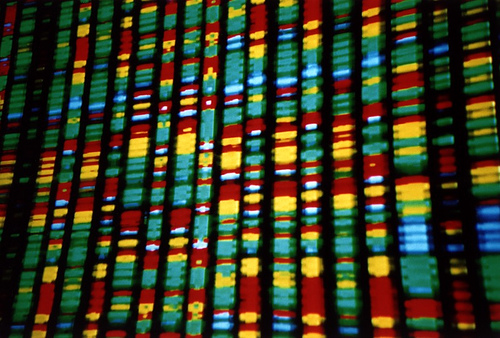
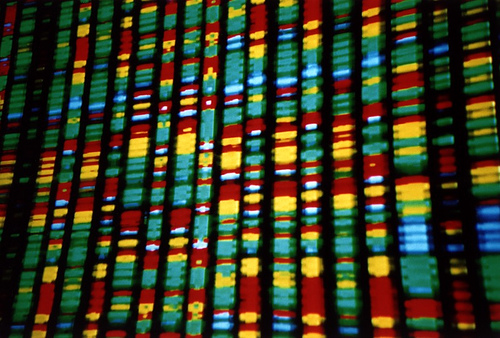
In conventional pharmacogenomic studies, genetic polymorphisms (including single nucleotide and copy number variations) are elucidated from case-control distribution of individuals usually representing ethnicity, severity of disease, and positive or negative response to treatment. However, the interpretation of a single genetic marker in this context is complicated, as the same marker may lead to multiple different phenotypes. Likewise, similar phenotypes can arise under different genetic backgrounds (models). This problem has led to the emergence of integrative approaches for combining statistical inference methods with bioinformatics-based text-mining, sequencing and expression analyses. An increasingly popular approach based on network analysis is challenged by the fact that a certain amount of the interpretation of the data is left to the subjective choices of the user. More rigorous statistical methods can be applied. However, these methods are also challenged by the fact that the developed measures of significance are provided outside the context of biology. In this perspective, we outline statistical methods and the development of evidence based pathways for identifying new biomarker correlations.
Statistical inference methods applied to pharmacogenomic studies
Statistical analysis of pharmacogenomic genotyping studies tend to be both compartmentalised (for instance, using structural polymorphisms in HapMap samples or population-specific linkage disequilibrium maps), and “top-to-bottom”, comprising a number of optional pre-defined steps in the assessment of the entire genome[1,2]. A single method of analysis, however, does not enable the full value of the data to be realised, and thus multiple approaches, described in more detail below, are recommended wherever possible.
Assuming for the moment that the probes being used represent single nucleotide polymorphisms (SNPs), a first step consists of removing SNPs from analysis based on quality or biological relevance. These may have low quality scores, minor allele frequencies (MAF) below a set threshold, or be out of Hardy-Weinberg-Equilibrium in control samples. Importantly, genotyping errors and population structure can introduce misleading signals that mimic true association. Association of each SNP with phenotype or phenotypic outcome (case/control, dose response) is then assessed with a range of statistical tests. The nature of the test and the degrees of freedom associated with it depend on the genetic models assumed or covered. The range of tests covers trend tests (Cochran-Armitage), and hypergeometric tests like Chi-Squared and Fisher’s exact test, which are applied for general, dominant-recessive, or allelic models of inheritance. At this stage, one also has to consider tackling the problem of multiple testing, including adjustment of p-values, the false positive report probability and the false discovery rate. After a much smaller set of statistically significant SNPs is identified, more detailed statistics are applied, identifying odds ratios and relative risks or potential gene-gene and gene-environment interactions. In many cases, a “two-step” approach is followed, in which this smaller set of probes is either then measured in a completely new set of samples, or re-analysed with a higher density of SNP markers. Overall, a detailed consideration of quality control issues with careful interpretation of summary statistics and graphical signal intensity plots can lead to informative associations for the follow-up or fine-mapping experiments.
However, it is not always so straight-forward, and it is worth noting that population stratification and multiple testing issues still remain difficult problems. The former can be controlled for by the obvious variables such as age, gender, and ethnicity, but it remains less tractable for “unknown” stratification factors, even if attempts are made to address it through principal-component-analysis approaches. Nevertheless, depending on the number of samples used in a study, and hence the power, genome-wide association studies will deliver anything from zero to a large number of candidate markers, with an associated false discovery rate (FDR). For the statistician, this is often the endpoint of an analysis, although at this stage little can be said about the biological mechanisms at work, patterns of responses in samples, or patient-specific effects.
There then remain a range of possibilities to make full pharmacogenomic use of the data:
- Investigate epigenetic factors e.g. by the use of methylation arrays
- Integrate with other publicly available genotyping data (available from NCBI dbgap http://www.ncbi.nlm.nih.gov/sites/entrez?db=gap)
- Integrate with other relevant data transcriptomic data[3].
The output from SNP studies are often no more than a series of statistically significant markers with their genomic locations. The task is to convert this comparatively information-light list of loci into a body of integrated information that can be efficiently searched, summarised and the key biological information retrieved. By mapping the loci to their respective positions either within or outwith a gene, biologically relevant information (e.g. tissue specificity of expression, transcript structure/alternative splicing forms, miRNA binding sites, predicted protein function, interactome information, metabolic pathway membership, transcriptional regulation groups, Gene Ontology terms, etc., etc.) can be ascribed to the locus. How best to mine a high dimension dataset like this?
Network analysis offers a solution to such a problem. Over recent years, it has been applied to systems biology with increasing frequency and success, as both biological knowledge and computing resources develop in power[4,5]. Network analysis permits relationships (e.g. correlations) to be investigated in an un-supervised manner, in which the data, for example, expression data, can be grouped into network graphs. These can subsequently be clustered into intra-graph groups using Markov clustering, and viewed in real-time three-dimensional space[6]. Annotation of the input data with statistical information (e.g. significance in a comparison, SNP association) enables both co-ordinate expression and statistical inference techniques to be synergistically applied to the dataset. An attractive feature of this approach is that it is essentially unsupervised, with no a priori knowledge assumed; as such, gene loci without a demonstrated function can acquire “guilt by association” status, and thus be taken forward for subsequent validation.
Validation necessarily involves mapping markers to the underlying biology and which is further fuelling a move from more traditional focussed single gene analysis to the study of multiple component interactions. For this purpose, the synthesis and construction of evidence based pathways is the next logical step.
Constructing evidence based pathways
When the output from high dimensional genomic data is integrated with the wealth of information from previously published investigations, the assembly of known and novel network associations is possible. The cause-effect relationships and annotation of multiple genes and gene products by such methods can aptly be described as a pathway biology approach of systems biology[7]. In support of this task, a comprehensive ‘evidence-based’ annotation of biological pathways could greatly enhance the current analysis paradigm, which to date has yet to fully explore the potential of causality linkage derived from pathway biology.
There is an increasing effort being put into representing biological pathways in a human-readable and computational accessible manner. These efforts include databases that aim to curate pathways, such as KEGG[8], Reactome[9], aMAZE[10] or PATIKA[11]; databases of experimentally and computationally derived protein interactions, such as MIPS[12] and DIP[13]; or tools that aim to extract pathway information from the scientific literature, for which examples include Ingenuity Pathway Analysis (Ingenuity Systems; www.ingenuity.com) and WikiPathways (www.wikipathways.org). However, challenges, common to all these resources, are the need to unambiguously describe pathways and minimise erroneous or false data that can lead to invalid models of their underlying biological complexity and/or context of the individual components.
To overcome these challenges, it is important that the acquisition of pathway information follows a systematic evidence-based approach tailored to the biology under investigation. Information relating to the components and interactions of pathways should follow a three-stage process. The first step is formulating the start and end of pathways. The start might be a drug-target, and the end a downstream marker or process. It could be a biological process, for instance a cytokine, and as an end point, apoptosis. Alternatively, it might be a known gene mutation, with the end point a clinical phenotype. The second stage is to search effectively for evidence of cause-effect relationships between the start and end points of the pathway. This may involve both automated and manual reading of text, mining of interaction databases and transcriptional networks. In the third stage, it is essential to critically appraise evidence about pathway members and their relationships for validity. As a rule-of-thumb, this involves acquiring multiple, independent, reports of association. To cast as large a net as possible, this approach requires the adoption of both hypothesis- and data-driven research sources in the synthesis, analysis and most importantly, interpretation of results.
The resultant pathway information represents a consensus of knowledge and can accordingly be stored in relational database resource and visualised either as an interaction network or as process-diagram.
Biomarker correlation and future need for integrating pathways
Thus the task of uncovering functional association of genetic biomarkers will be greatly aided in the future by coupling the power of statistical association studies with prior knowledge, thereby mapping biomarkers to a pathway. This process gives rise to an important statistical correlation benefit for classifying biomarkers based on pathway assignment.
Concluding remarks
The emerging field of biomarker discovery for the pharmaceutical industry has benefited greatly from the human genome project, which introduced the field of pharmacogenomics. However, interpreting the data from genomic based screens still typically relies on genetic locus-specific assessment relative to the disease biology. To move the field forward, it is necessary to relate genetic markers to specific evidence-based pathway models. This is indeed the objective of many of the current efforts in the functional annotation of the human genome. Arguably, the field of pharmacogenomics is developing as a viable field for biomarker identification. However, some serious challenges remain in developing techniques that are computationally faster and allow for the integration of validated pathway models. The emerging availability of large-scale DNA sequencing data sets produced by next-generation sequencing technologies will pose yet further exciting challenges to the efficient performance of statistical biomarker pathway association studies.
References
- Ziegler A, König IR, Thompson JR. Biostatistical aspects of genome-wide association studies. Biom J. 2008 Feb;50(1):8-28. PMID: 18217698
- McCarroll SA. Extending genome-wide association studies to copy-number variation. Hum Mol Genet. 2008 Oct 15;17(R2):R135-42. PMID: 18852202
- Nica AC, Dermitzakis ET. Using gene expression to investigate the genetic basis of complex disorders. Hum Mol Genet. 2008 Oct 15;17(R2):R129-34. PMID: 18852201
- Feist AM, Herrgård MJ, Thiele I, Reed JL, Palsson BØ. Reconstruction of biochemical networks in microorganisms. Nat Rev Microbiol. 2009 Feb;7(2):129-43. Epub 2008 Dec 31. PMID: 19116616
- Horvath S, Dong J. Geometric interpretation of gene coexpression network analysis. PLoS Comput Biol. 2008 Aug 15;4(8):e1000117. PMID: 18704157
- Freeman TC, Goldovsky L, Brosch M, van Dongen S, Mazière P, Grocock RJ, Freilich S, Thornton J, Enright AJ. Construction, visualisation, and clustering of transcription networks from microarray expression data. PLoS Comput Biol. 2007 Oct;3(10):2032-42. PMID: 17967053
- Watterson S, Marshall S, Ghazal P. Logic models of pathway biology. Drug Discov Today. 2008 May;13(9-10):447-56. PMID: 18468563
- Aoki-Kinoshita KF, Kanehisa M. Gene annotation and pathway mapping in KEGG. Methods Mol Biol. 2007 396:71-91. PMID: 18025687
- Joshi-Tope G, Gillespie M, Vastrik I, D’Eustachio P, Schmidt E, de Bono B, Jassal B, Gopinath GR, Wu GR, Matthews L, Lewis S, Birney E, Stein L. Reactome: a knowledgebase of biological pathways. Nucleic Acids Res. 2005 Jan 1;33(Database issue):D428-32. PMID: 15608231
- Lemer C, Antezana E, Couche F, Fays F, Santolaria X, Janky R, Deville Y, Richelle J, Wodak SJ. The aMAZE LightBench: a web interface to a relational database of cellular processes. Nucleic Acids Res. 2004 Jan 1;32(Database issue):D443-8. PMID: 14681453
- Dogrusoz U, Erson EZ, Giral E, Demir E, Babur O, Cetintas A, Colak R. PATIKAweb: a Web interface for analyzing biological pathways through advanced querying and visualization. Bioinformatics. 2006 Feb 1;22(3):374-5. PMID: 16287939
- Pagel P, Kovac S, Oesterheld M, Brauner B, Dunger-Kaltenbach I, Frishman G, Montrone C, Mark P, Stümpflen V, Mewes HW, Ruepp A, Frishman D. The MIPS mammalian protein-protein interaction database. Bioinformatics. 2005 Mar;21(6):832-4. PMID: 15531608
- Salwinski L, Miller CS, Smith AJ, Pettit FK, Bowie JU, Eisenberg D. The Database of Interacting Proteins: 2004 update. Nucleic Acids Res. 2004 Jan 1;32(Database issue):D449-51. PMID: 14681454